Publications
Last Updated: September 2023.
An up to date list of all publications can be found on my Google Scholar profile.
2024
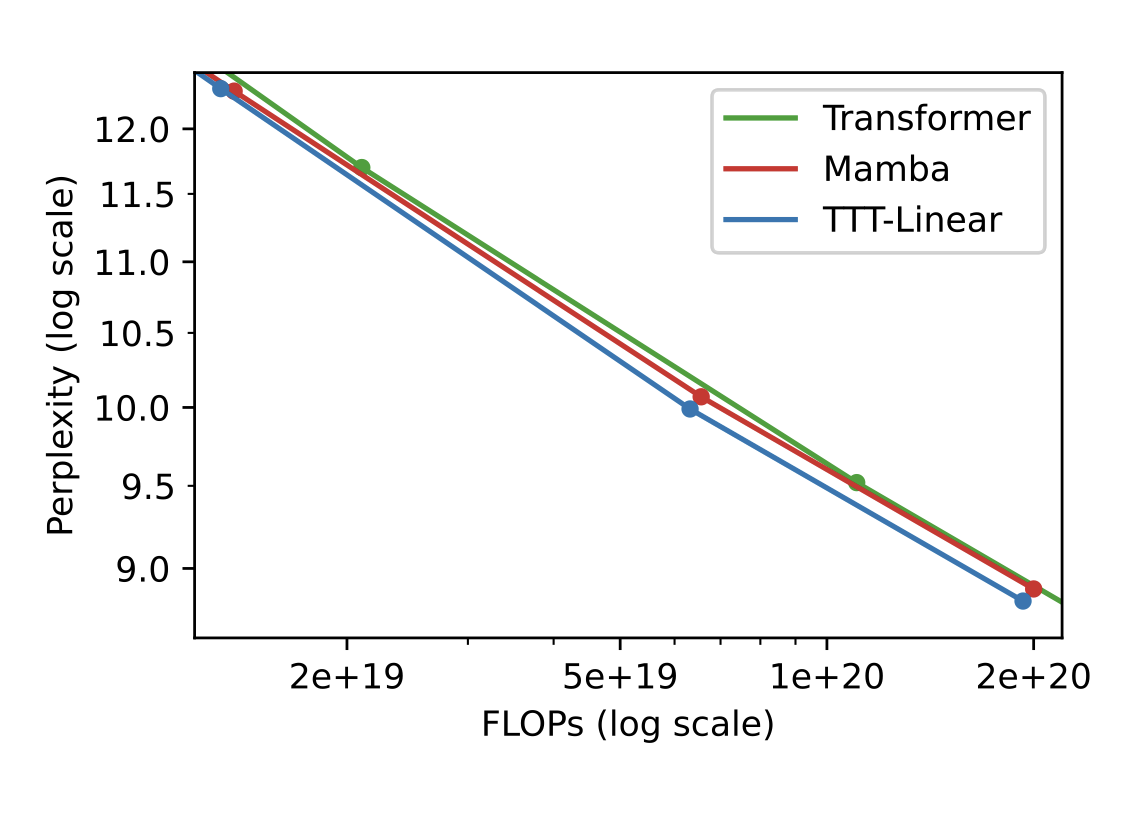
Yu Sun, Xinhao Li, Karan Dalal, Jiarui Xu, Arjun Vikram, Genghan Zhang, Y. Dubois, Xinlei Chen, Xiaolong Wang, Sanmi Koyejo, Tatsunori Hashimoto, Carlos Guestrin
ArXivTLDR: A new language model layer that is more expressive than RNN but more efficient than attention.
NLP, Self-Supervised Learning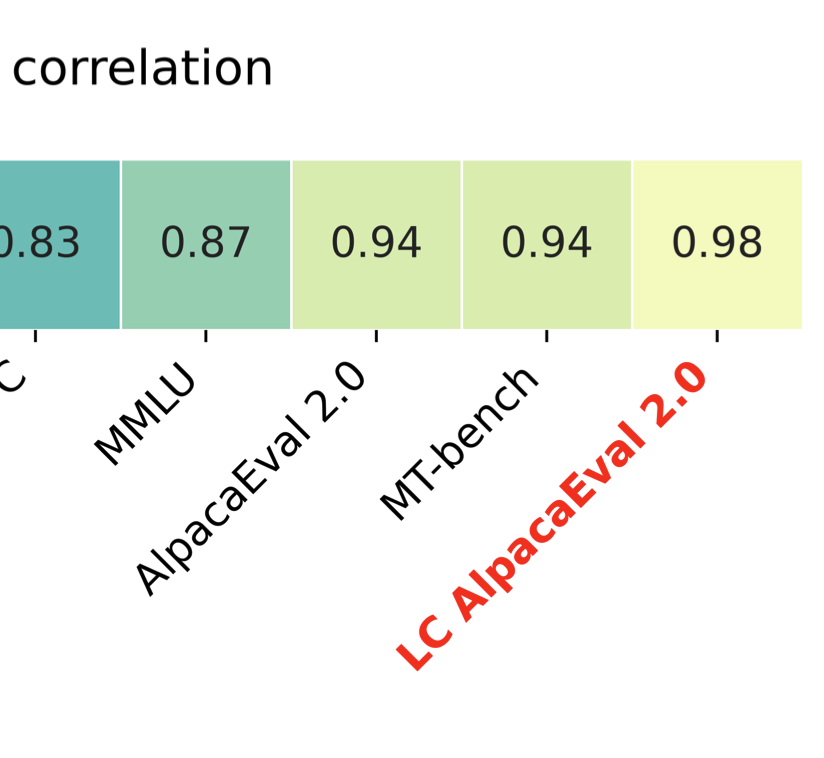
Y. Dubois, B. Galambosi, P. Liang, T. Hashimoto
COLM 2024TLDR: We decrease the bias of AlpacaEval for longer outputs using regression analysis.
Evaluation, NLP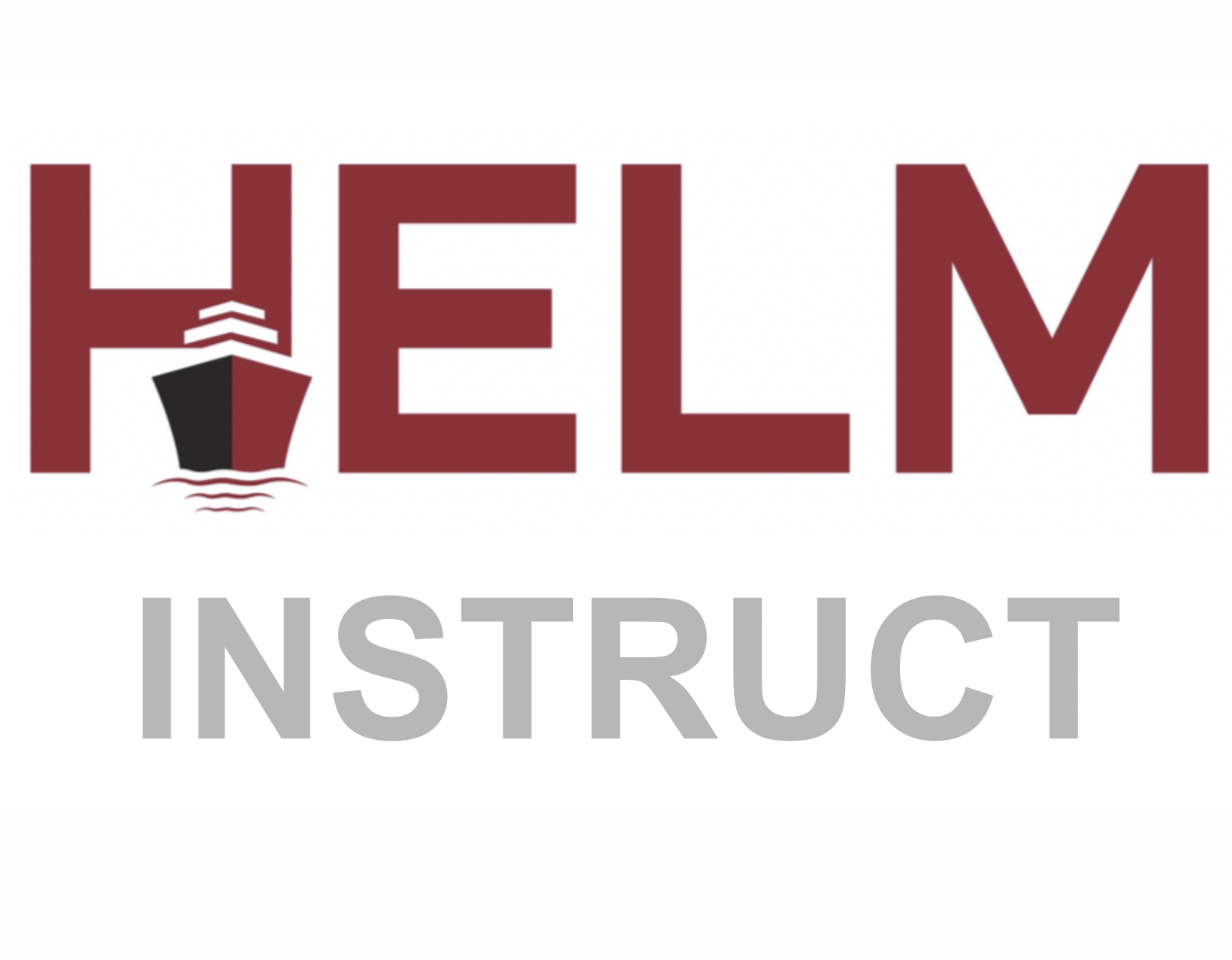
Y. Zhang, Y. Mai, J. Somerville Roberts, R. Bommasani, Y. Dubois, P. Liang
GitHubTLDR: Multidimensional evaluation of instruction following LLM with absolute scores.
Evaluation, NLP2023
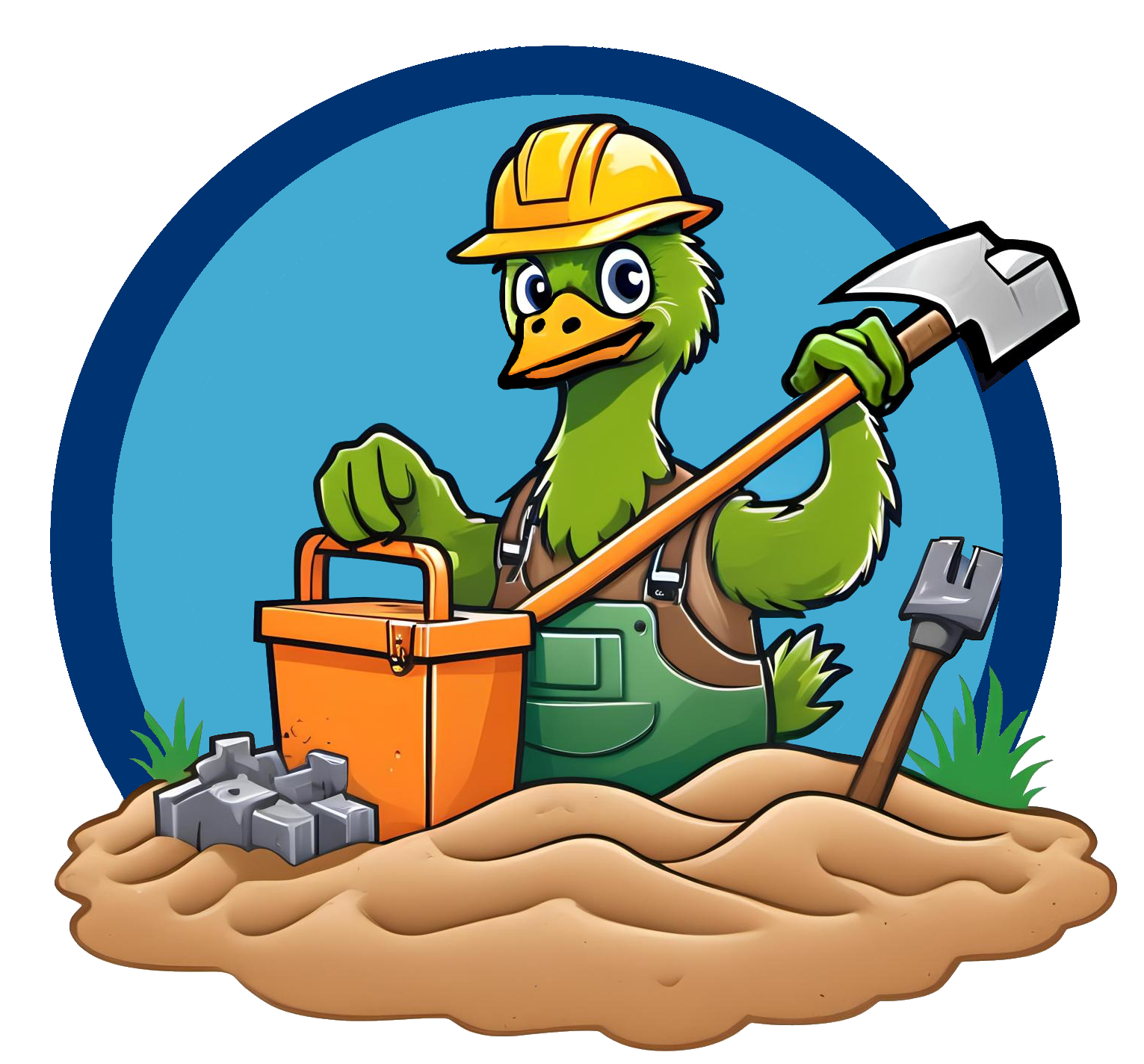
Y. Ruan*, H. Dong*, A. Wang, S. Pitis, Y. Zhou, J. Ba, Y. Dubois, C. J. Maddison, T. Hashimoto
ICLR 2024 Spotlight Presentation πTLDR: An LM-based emulation framework for identifying the risks of LM agents at scale.
Selected Papers Evaluation, NLP, Safety,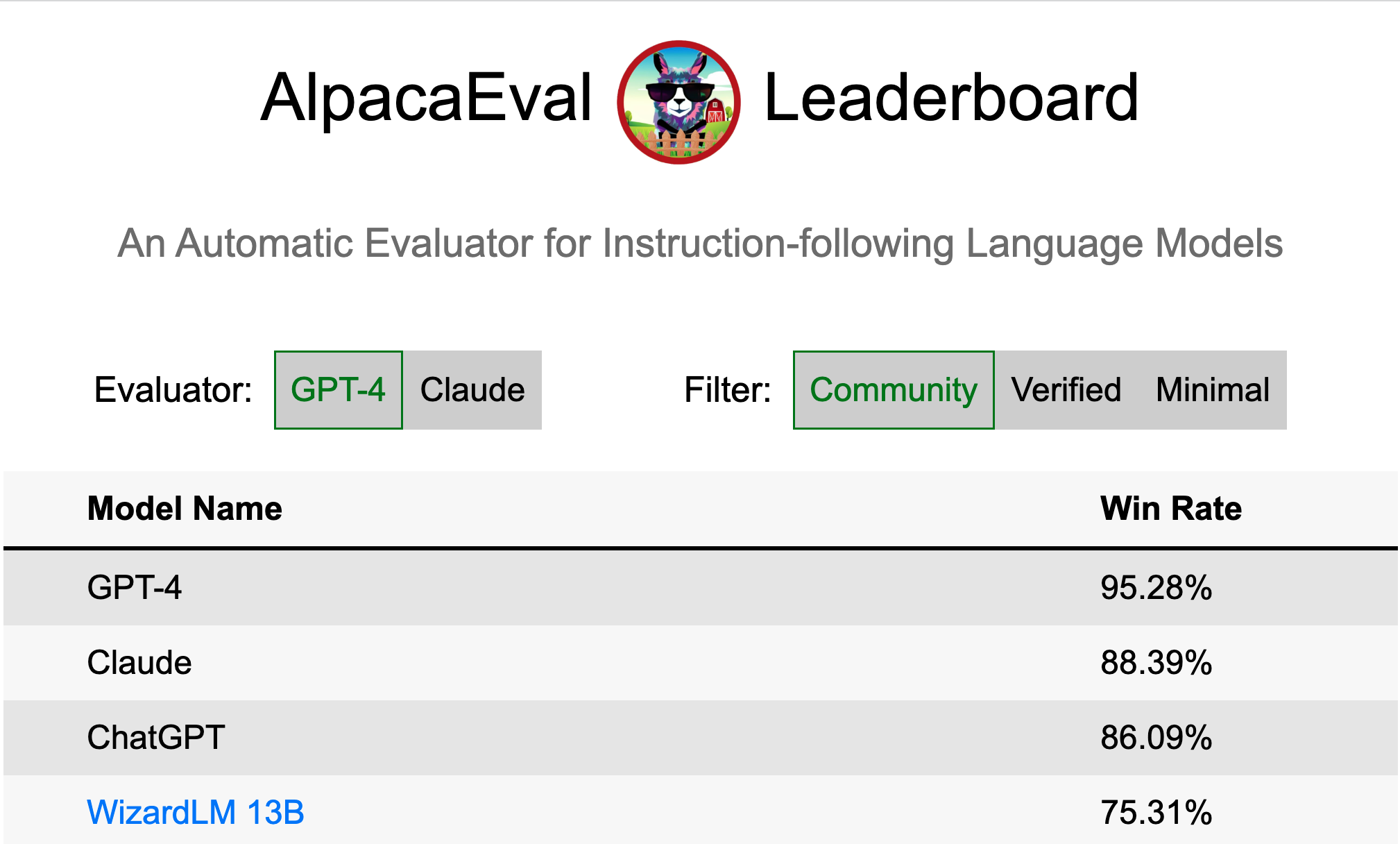
X. Li*, T. Zhang*, Y. Dubois*, R. Taori*, I. Gulrajani, C. Guestrin, P. Liang, T. Hashimoto
GitHubTLDR: A validated automatic evaluator for instruction-following language models. High-quality, cheap, and fast.
Selected Papers Evaluation, NLP, RLHF,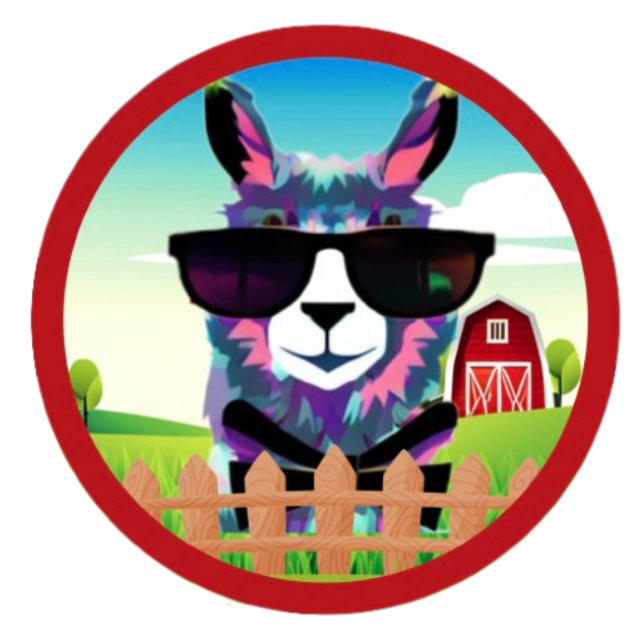
Y. Dubois*, X. Li*, R. Taori*, T. Zhang*, I. Gulrajani, J. Ba, C. Guestrin, P. Liang, T. Hashimoto
NeurIPS 2023 Spotlight Presentation πTLDR: AlpacaFarm replicates the RLHF process at a fraction of the time (<24h) and cost ($<200), enabling the research community to advance instruction following research.
Selected Papers Evaluation, NLP, RLHF,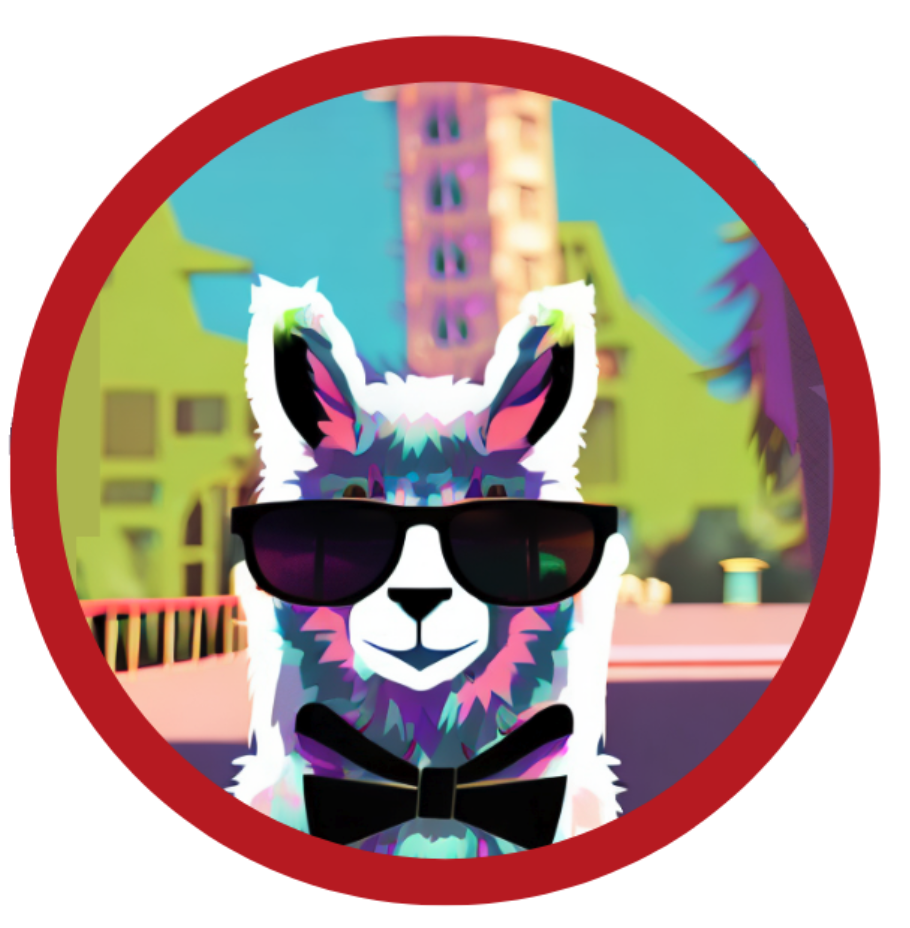
R. Taori*, I. Gulrajani*, T. Zhang*, Y. Dubois*, X. Li*, C. Guestrin, P. Liang, T. Hashimoto
GitHubTLDR: We introduce Alpaca 7B, a instruction-following fine-tuned LLaMA model. On our preliminary evaluation, Alpaca behaves qualitatively similarly to OpenAIβs text-davinci-003, while being surprisingly small and easy/cheap to reproduce (<600$).
Selected Papers NLP, RLHF, Self-Supervised Learning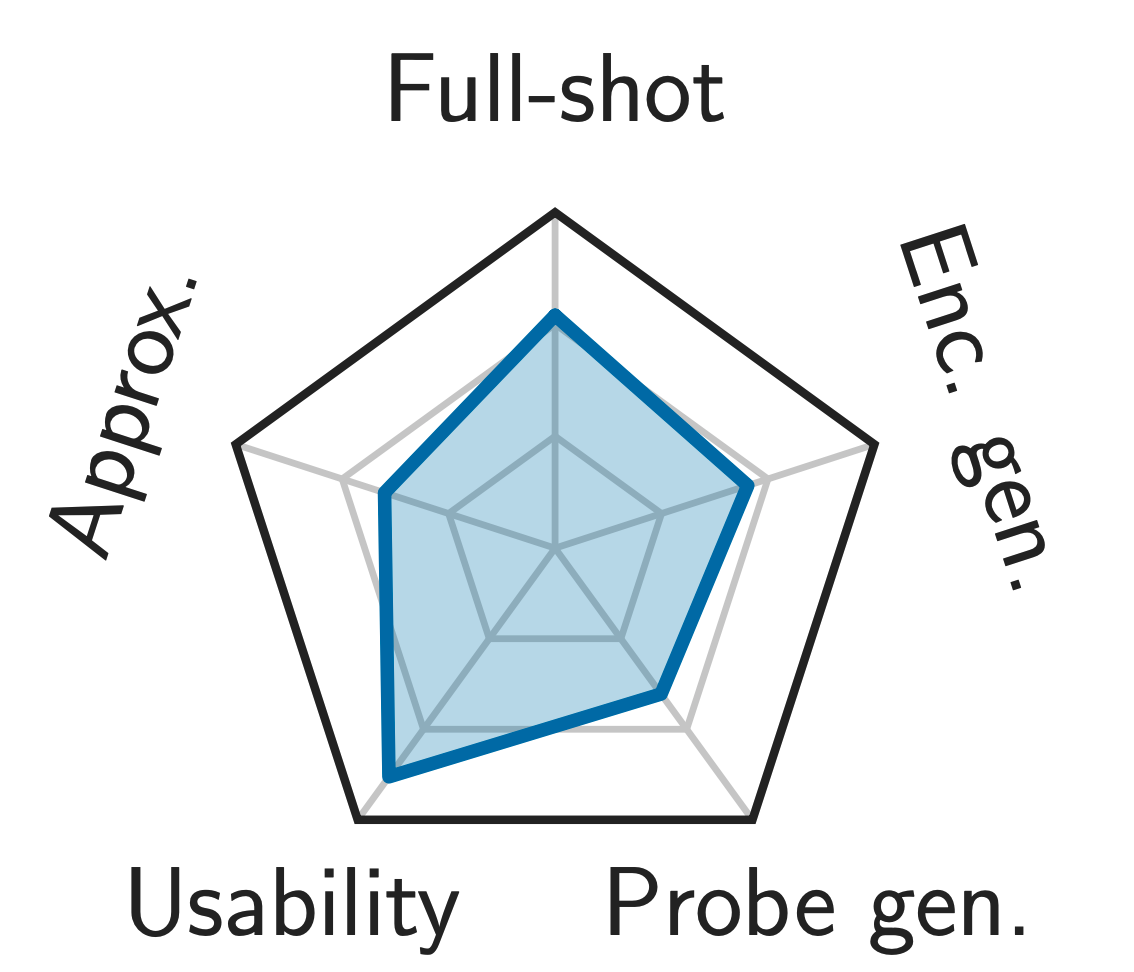
Y. Dubois, T. Hashimoto, P. Liang
ICML 2023 Oral Presentation πTLDR: We derive a risk decomposition for self-supervised learning and use it to evaluate 169 pretrained models.
Selected Papers Evaluation, Representation Learning, Self-Supervised Learning, Vision2022
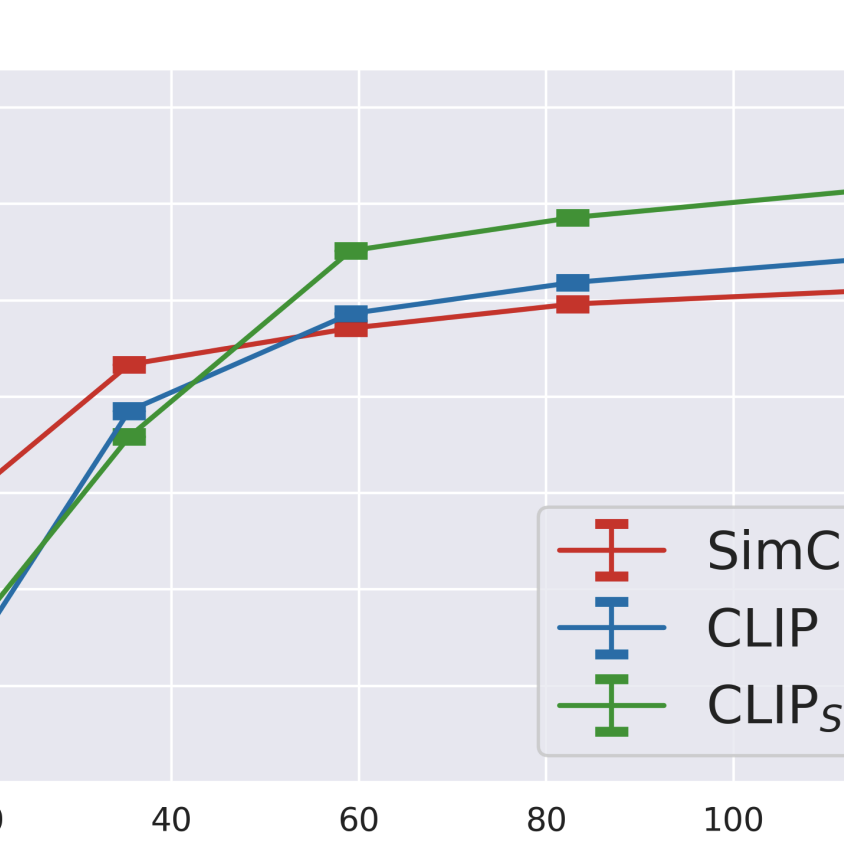
S. Santurkar, Y. Dubois, R. Taori, P. Liang, T. Hashimoto
ICLR 2022TLDR: Our work performs a systematic investigation into whether additional language supervision (in CLIP) helps models learn more transferrable representations.
NLP, Representation Learning, Self-Supervised Learning, Vision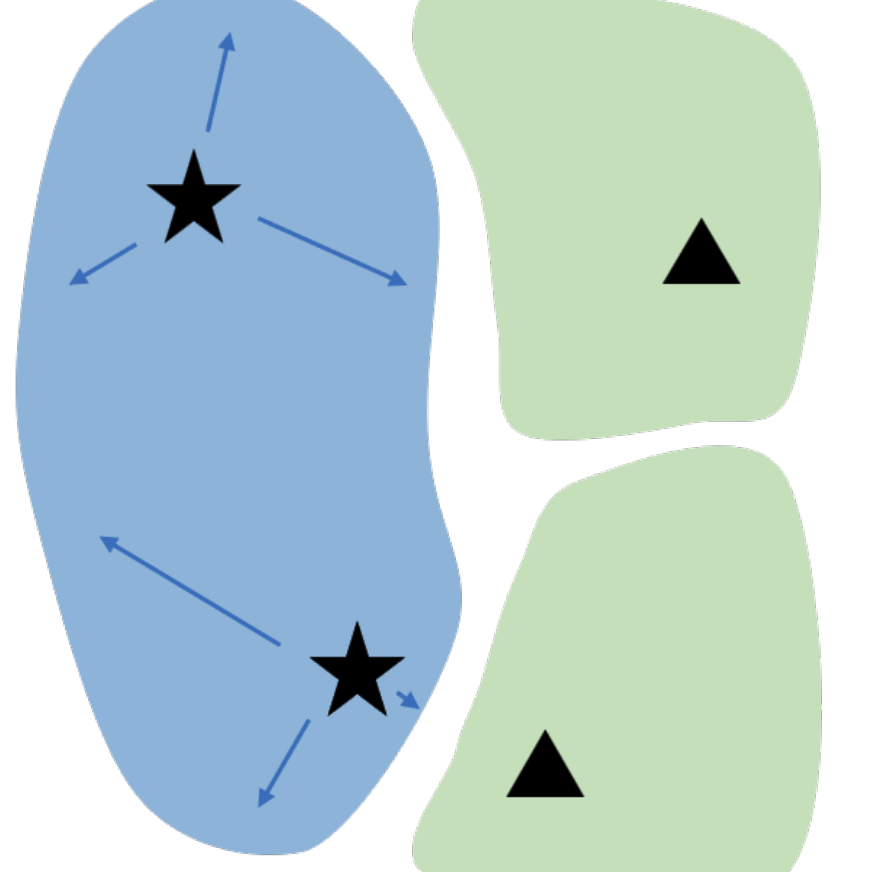
N. Miao, E. Mathieu, Y. Dubois, T. Rainforth, Y. W. Teh, A. Foster, H. Kim
ICML 2023TLDR: We introduce a method for automatically learning input-specific augmentations from data.
Invariance, Vision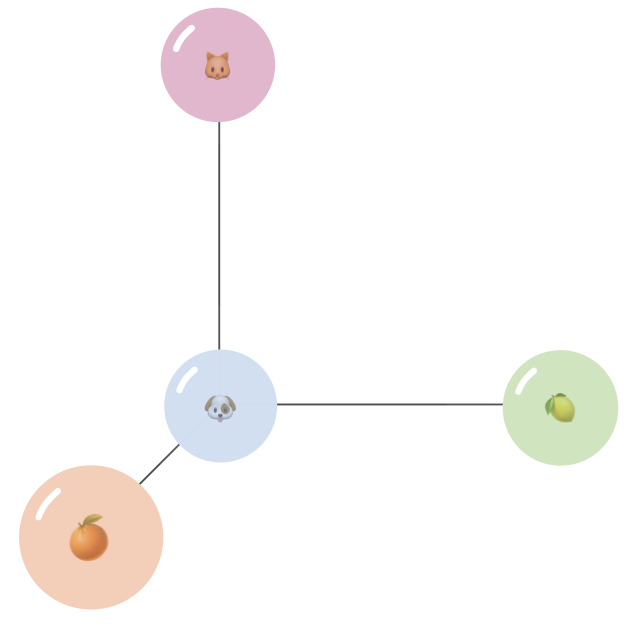
Y. Dubois, T. Hashimoto, S. Ermon, P. Liang
NeurIPS 2022TLDR: We characterize idealized self-supervised representations, which leads to actionable insights for improving SSL algorithms.
Selected Papers Invariance, Representation Learning, Self-Supervised Learning, Vision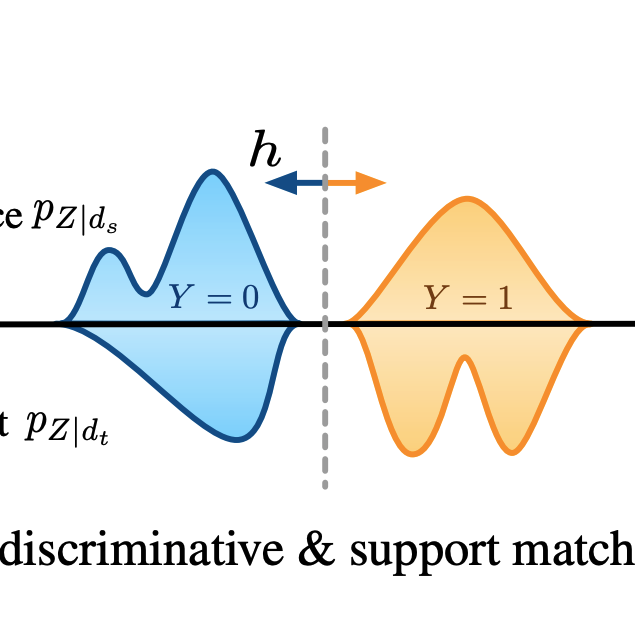
Y Ruan*, Y. Dubois*, C. J. Maddison
ICLR 2021TLDR: We give a simple variational objective whose optima are exactly the set of representations that are robust under covariate shift.
Information Theory, Invariance, Representation Learning, Robustness, Self-Supervised Learning, Vision2021
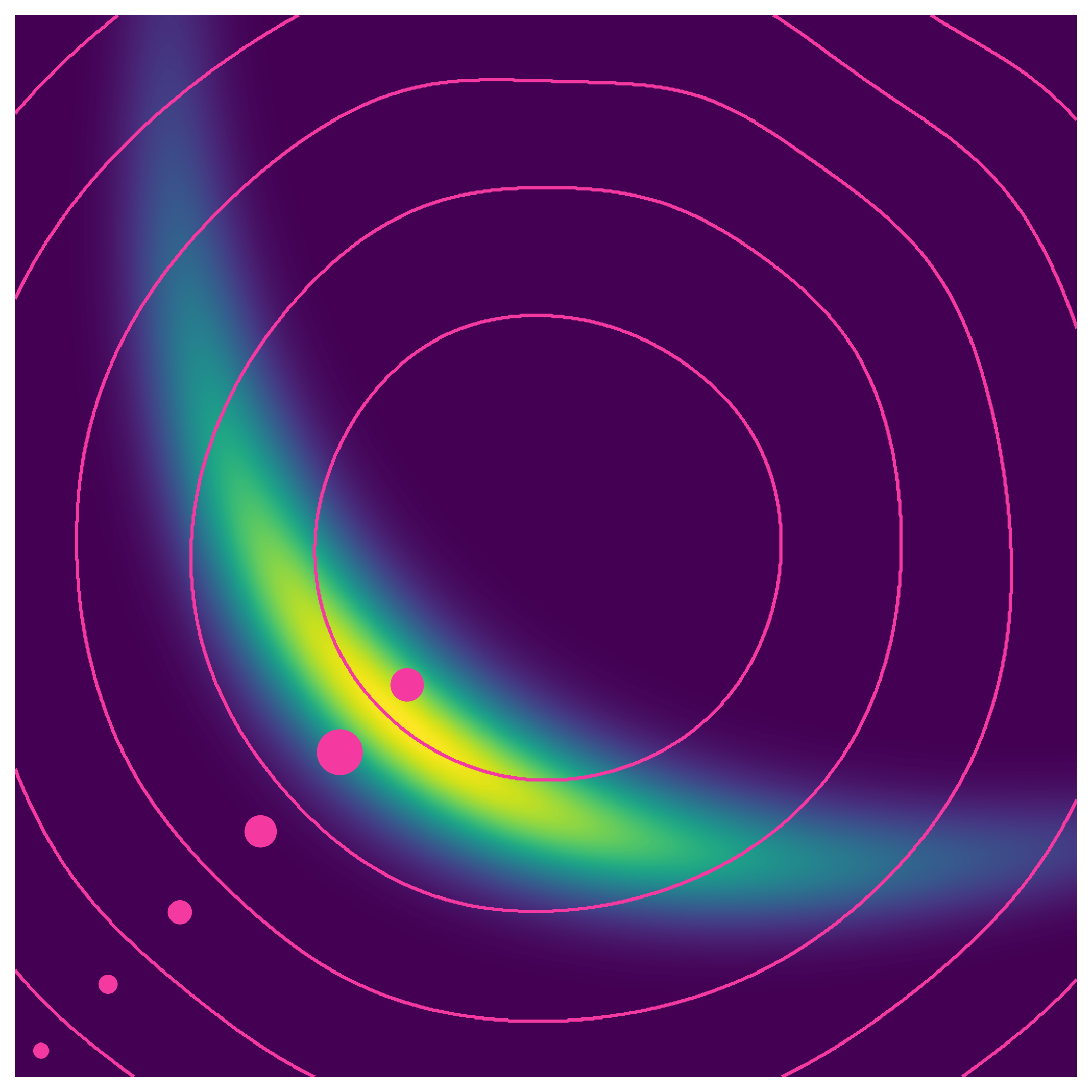
Y. Dubois, B. Bloem-Reddy, K. Ullrich, C. J. Maddison
NeurIPS 2021 Spotlight Presentation πTLDR: We formalize compression with respect to ML algorithms rather than human perception.
Selected Papers Compression, Information Theory, Invariance, Representation Learning, Self-Supervised Learning, Vision2020
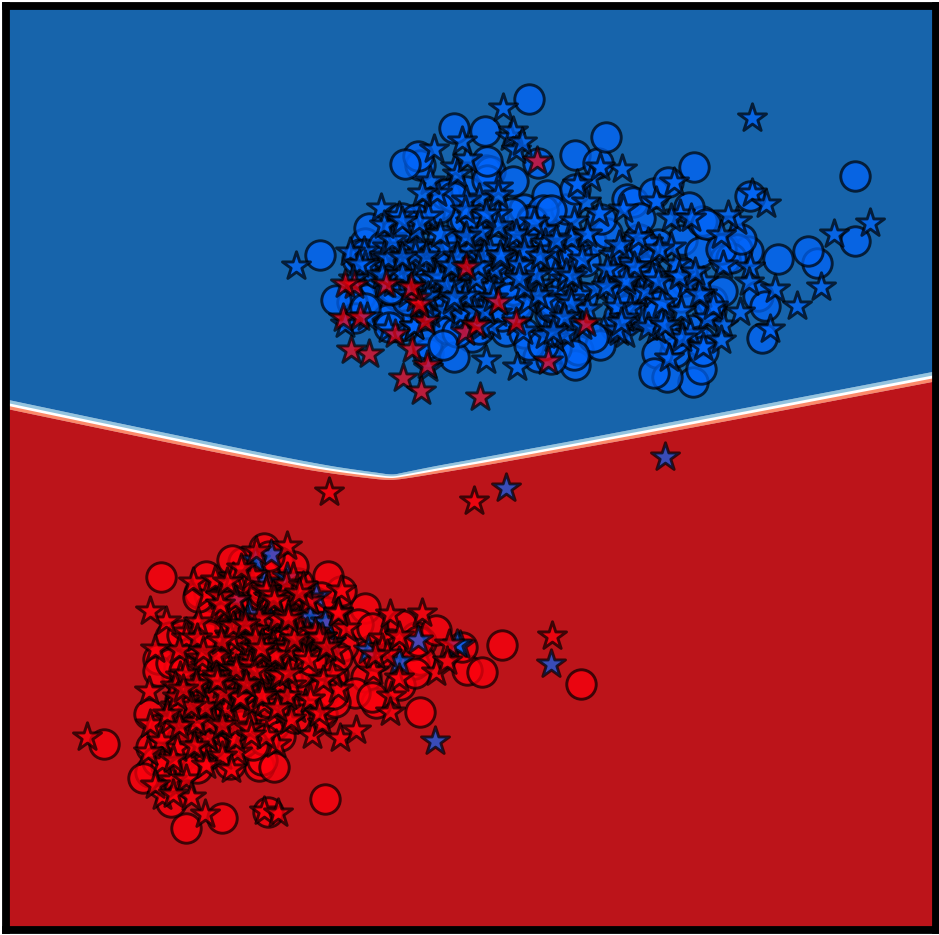
Y. Dubois, D. Kiela, D. J. Schwab, R. Vedantam
NeurIPS 2020 Spotlight Presentation πTLDR: We characterize and approximate optimal representations for supervised learning.
Information Theory, Representation Learning, Vision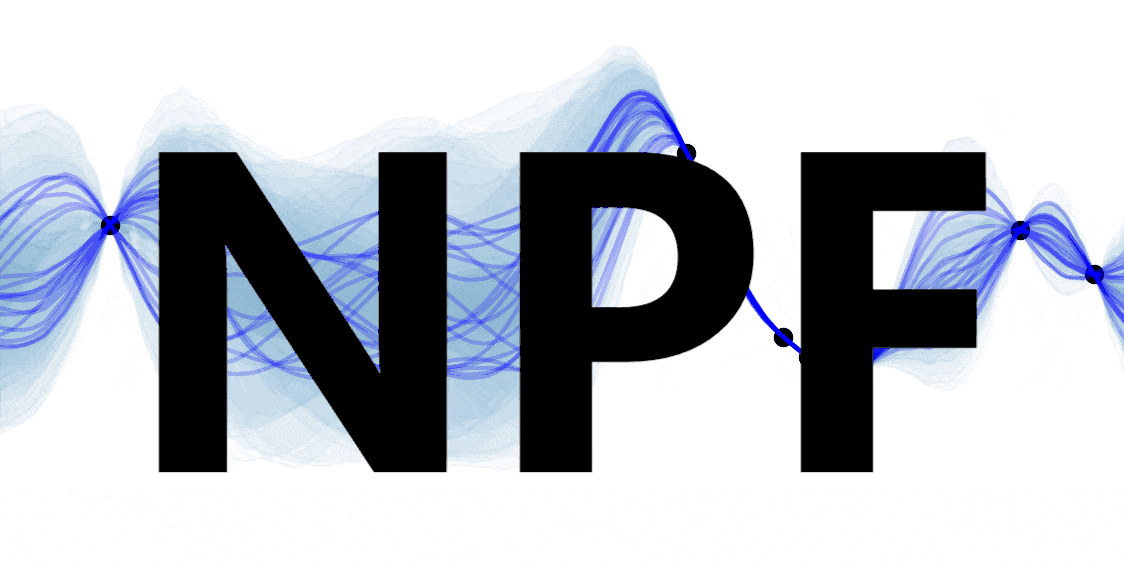
Y. Dubois*, J. Gordon*, A. Foong*
GitHubTLDR: A simple and unifying explanation of the neural process family, which are a collection of models that meta-learn a distribution over predictors.
Equivariance, Neural Processes, Time Series, Uncertainty, Vision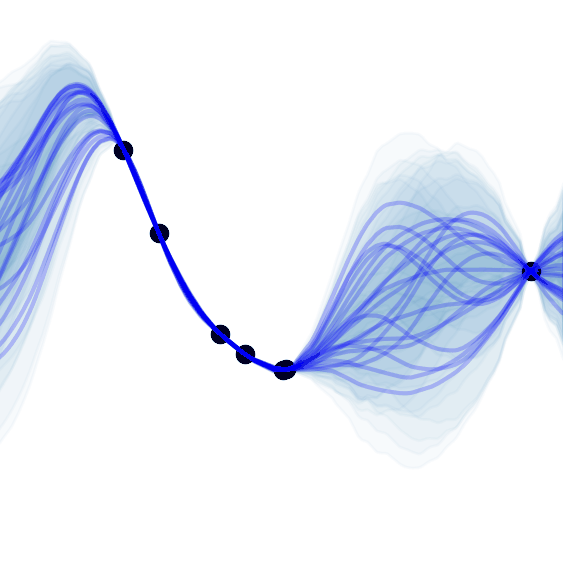
A. Y. K. Foong*, W. P. Bruinsma*, J. Gordon*, Y. Dubois, J. Requeima, R. E. Turner
NeurIPS 2020TLDR: We propose a translation equivariant (latent) neural process.
Equivariance, Neural Processes, Time Series, Uncertainty, Vision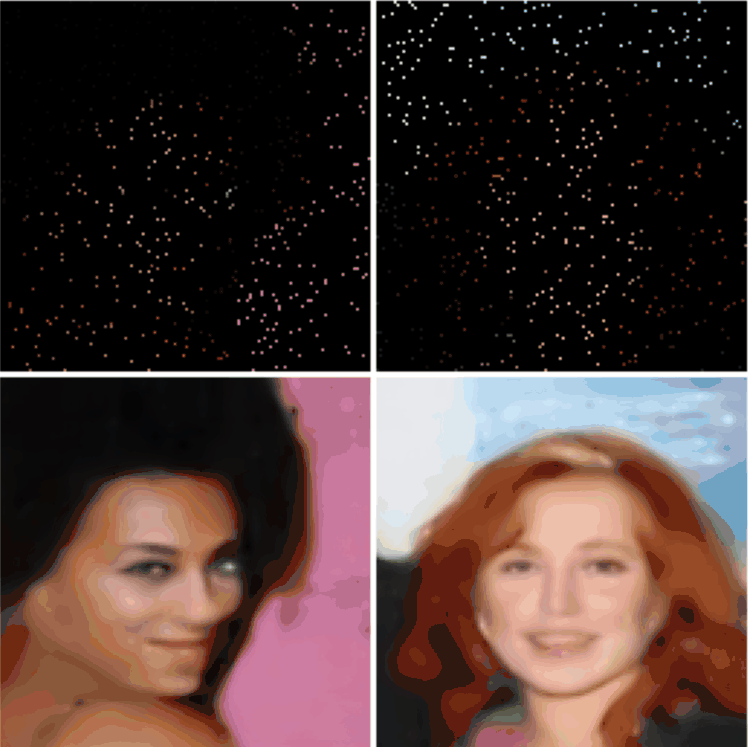
J. Gordon*, W. P. Bruinsma*, A. Y. K. Foong, J. Requeima,Y. Dubois, R. E. Turner
ICLR 2020 Oral Presentation πTLDR: We propose a translation equivariant conditional neural process.
Equivariance, Neural Processes, Time Series, Uncertainty, Vision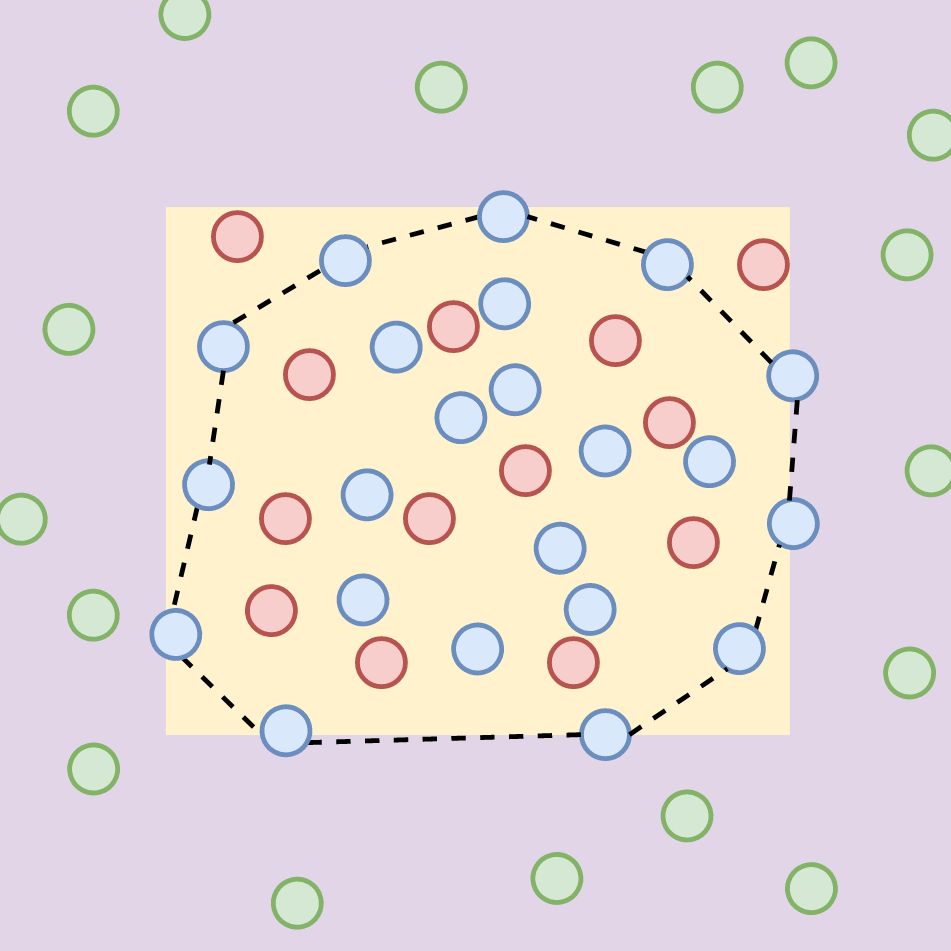
Y. Dubois, Gautier Dagan, Dieuwke Hupkes, Elia Bruni
ACL 2020TLDR: We propose an attention that improves extrapolation capacity of neural NLP models.
NLP, Robustness