Publications by Tags
Selected Papers Compression, Equivariance, Evaluation, Information Theory, Invariance, Neural Processes, NLP, Representation Learning, RLHF, Robustness, Safety, Self-Supervised Learning, Time Series, Uncertainty, VisionSelected Papers
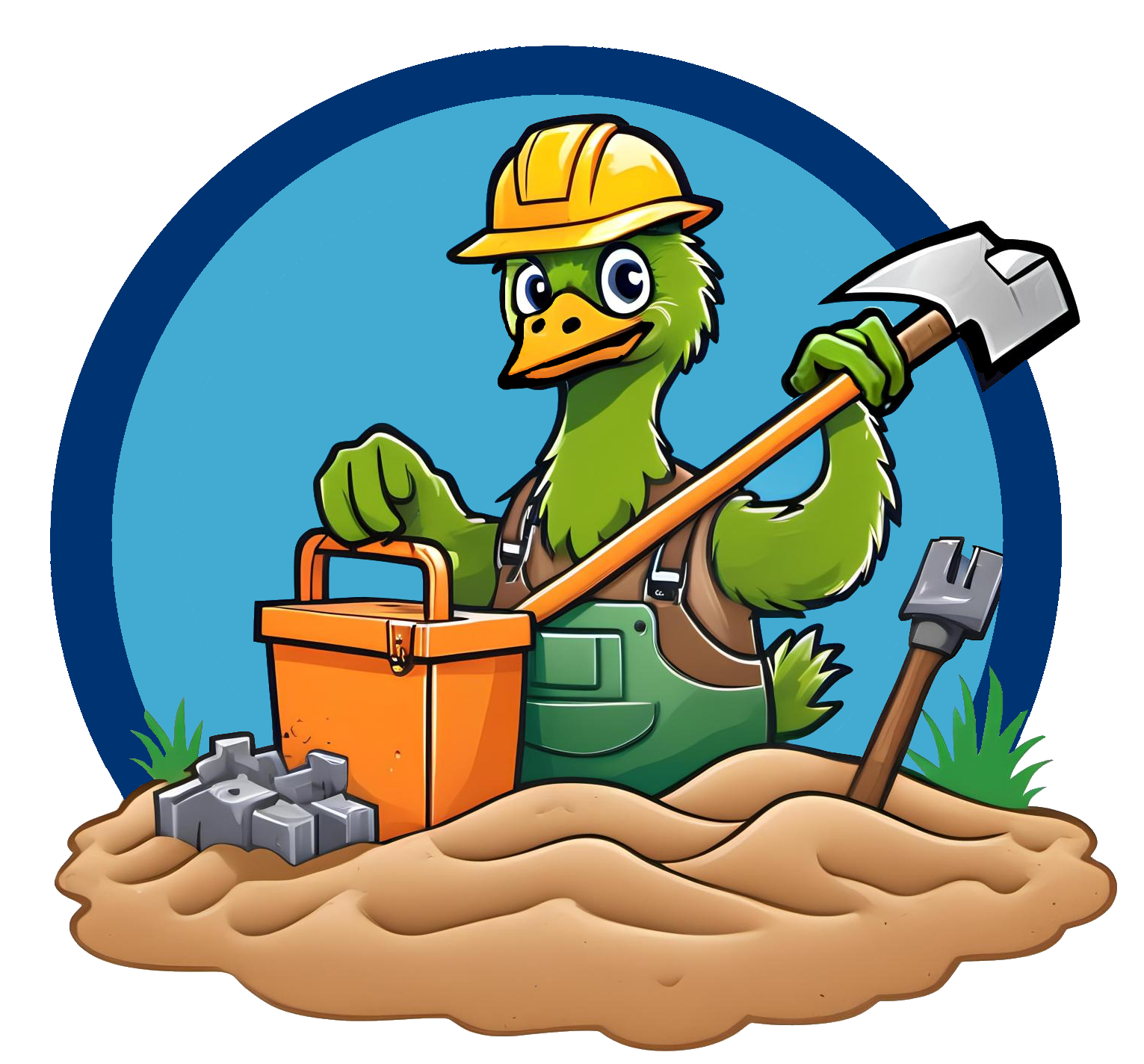
Y. Ruan*, H. Dong*, A. Wang, S. Pitis, Y. Zhou, J. Ba, Y. Dubois, C. J. Maddison, T. Hashimoto
ICLR 2024 Spotlight Presentation πTLDR: An LM-based emulation framework for identifying the risks of LM agents at scale.
Selected Papers Evaluation, NLP, Safety,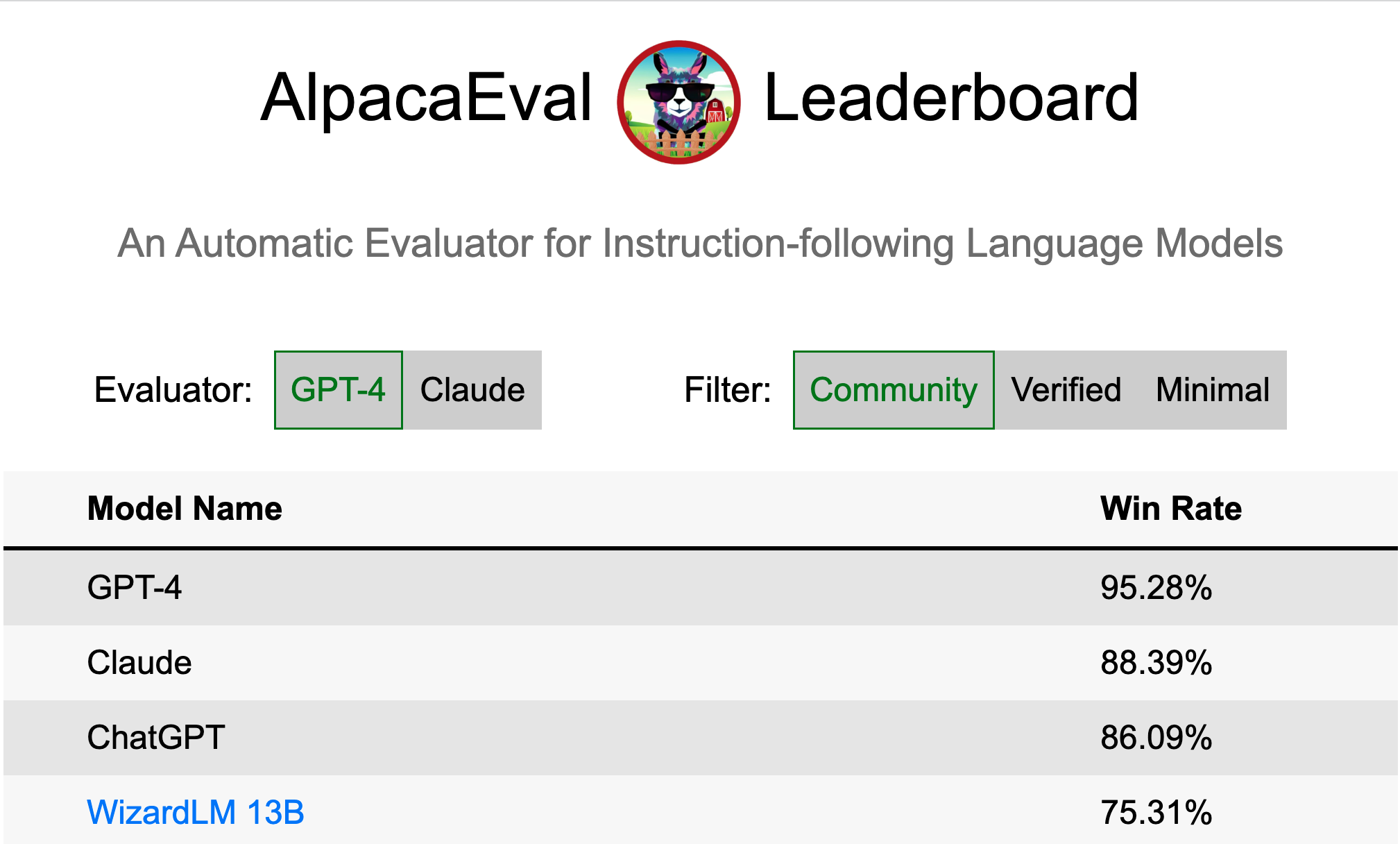
X. Li*, T. Zhang*, Y. Dubois*, R. Taori*, I. Gulrajani, C. Guestrin, P. Liang, T. Hashimoto
GitHubTLDR: A validated automatic evaluator for instruction-following language models. High-quality, cheap, and fast.
Selected Papers Evaluation, NLP, RLHF,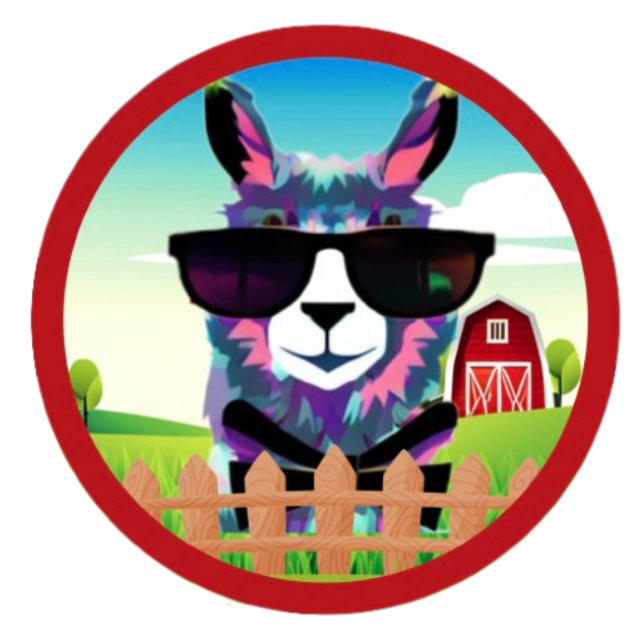
Y. Dubois*, X. Li*, R. Taori*, T. Zhang*, I. Gulrajani, J. Ba, C. Guestrin, P. Liang, T. Hashimoto
NeurIPS 2023 Spotlight Presentation πTLDR: AlpacaFarm replicates the RLHF process at a fraction of the time (<24h) and cost ($<200), enabling the research community to advance instruction following research.
Selected Papers Evaluation, NLP, RLHF,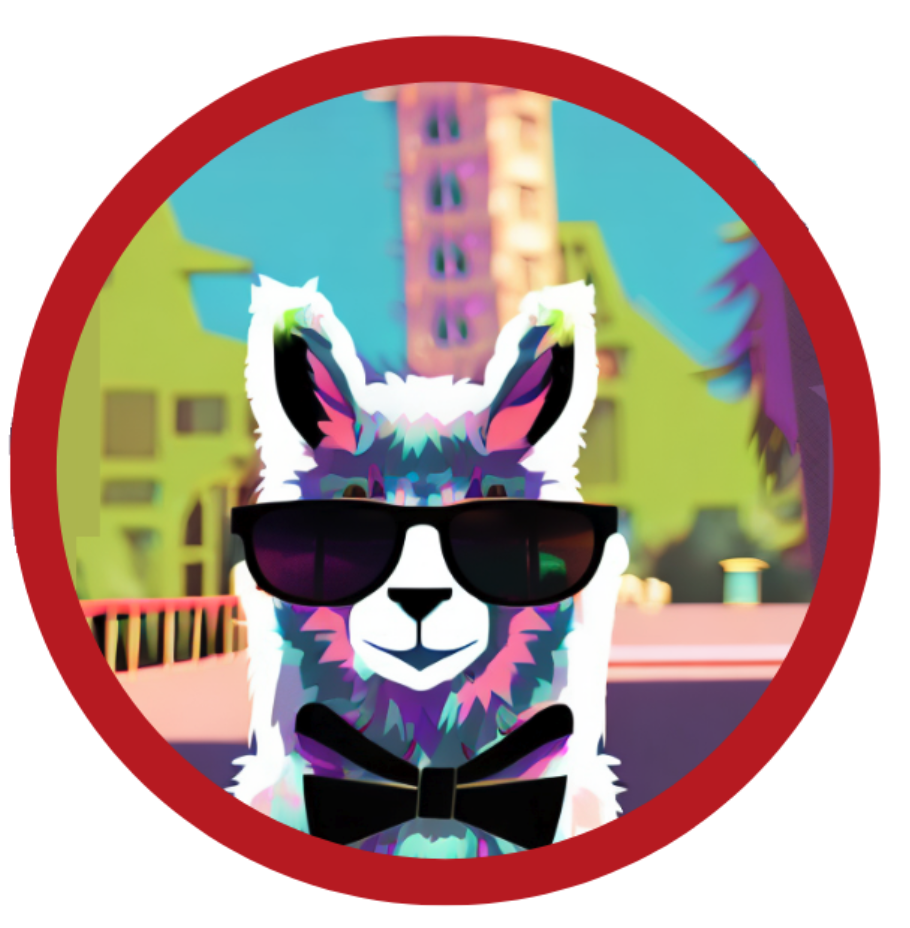
R. Taori*, I. Gulrajani*, T. Zhang*, Y. Dubois*, X. Li*, C. Guestrin, P. Liang, T. Hashimoto
GitHubTLDR: We introduce Alpaca 7B, a instruction-following fine-tuned LLaMA model. On our preliminary evaluation, Alpaca behaves qualitatively similarly to OpenAIβs text-davinci-003, while being surprisingly small and easy/cheap to reproduce (<600$).
Selected Papers NLP, RLHF, Self-Supervised Learning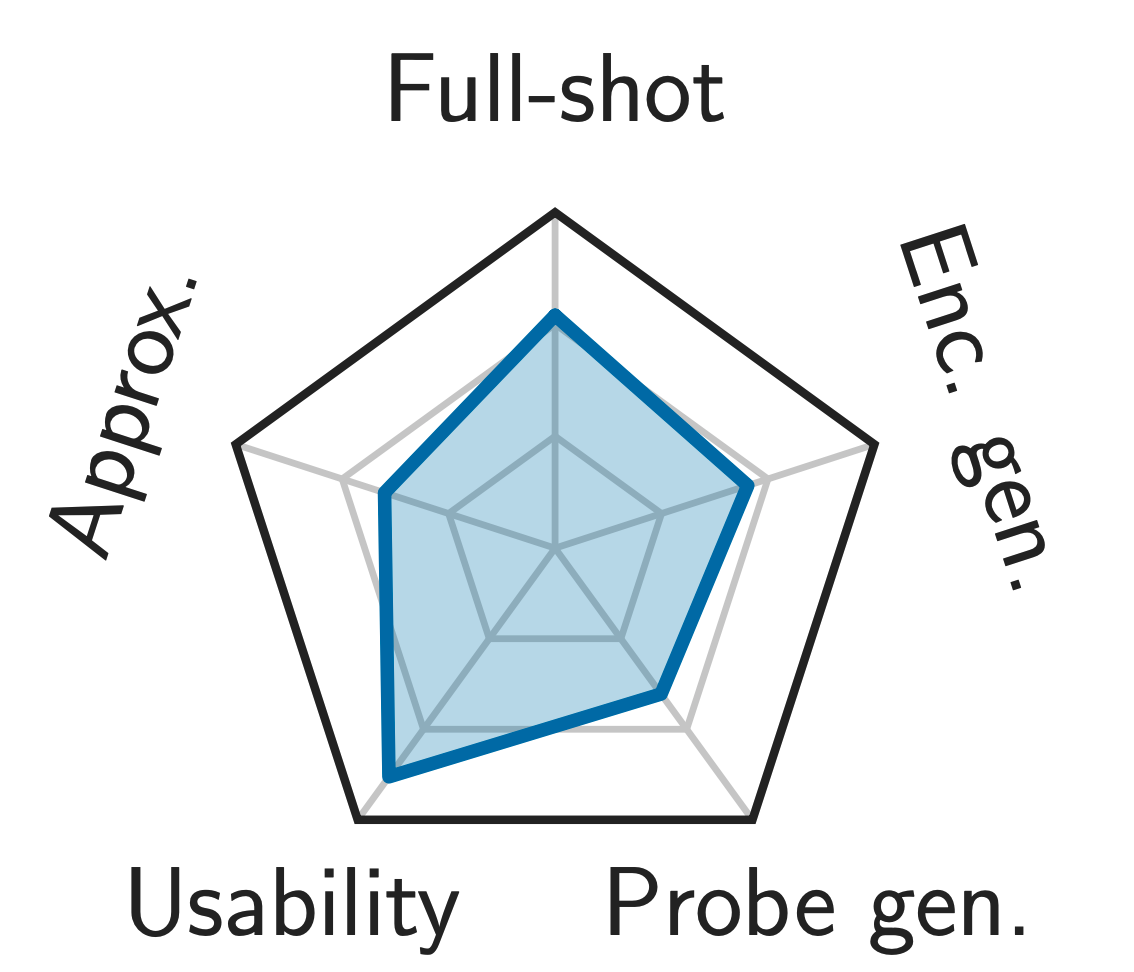
Y. Dubois, T. Hashimoto, P. Liang
ICML 2023 Oral Presentation πTLDR: We derive a risk decomposition for self-supervised learning and use it to evaluate 169 pretrained models.
Selected Papers Evaluation, Representation Learning, Self-Supervised Learning, Vision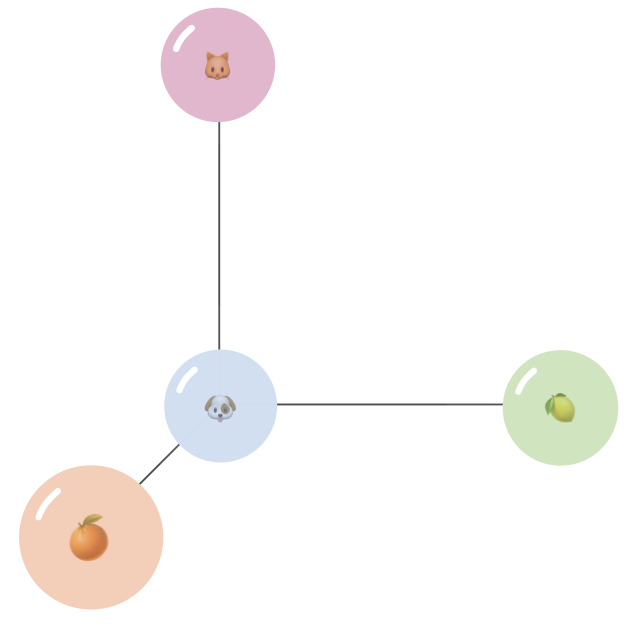
Y. Dubois, T. Hashimoto, S. Ermon, P. Liang
NeurIPS 2022TLDR: We characterize idealized self-supervised representations, which leads to actionable insights for improving SSL algorithms.
Selected Papers Invariance, Representation Learning, Self-Supervised Learning, Vision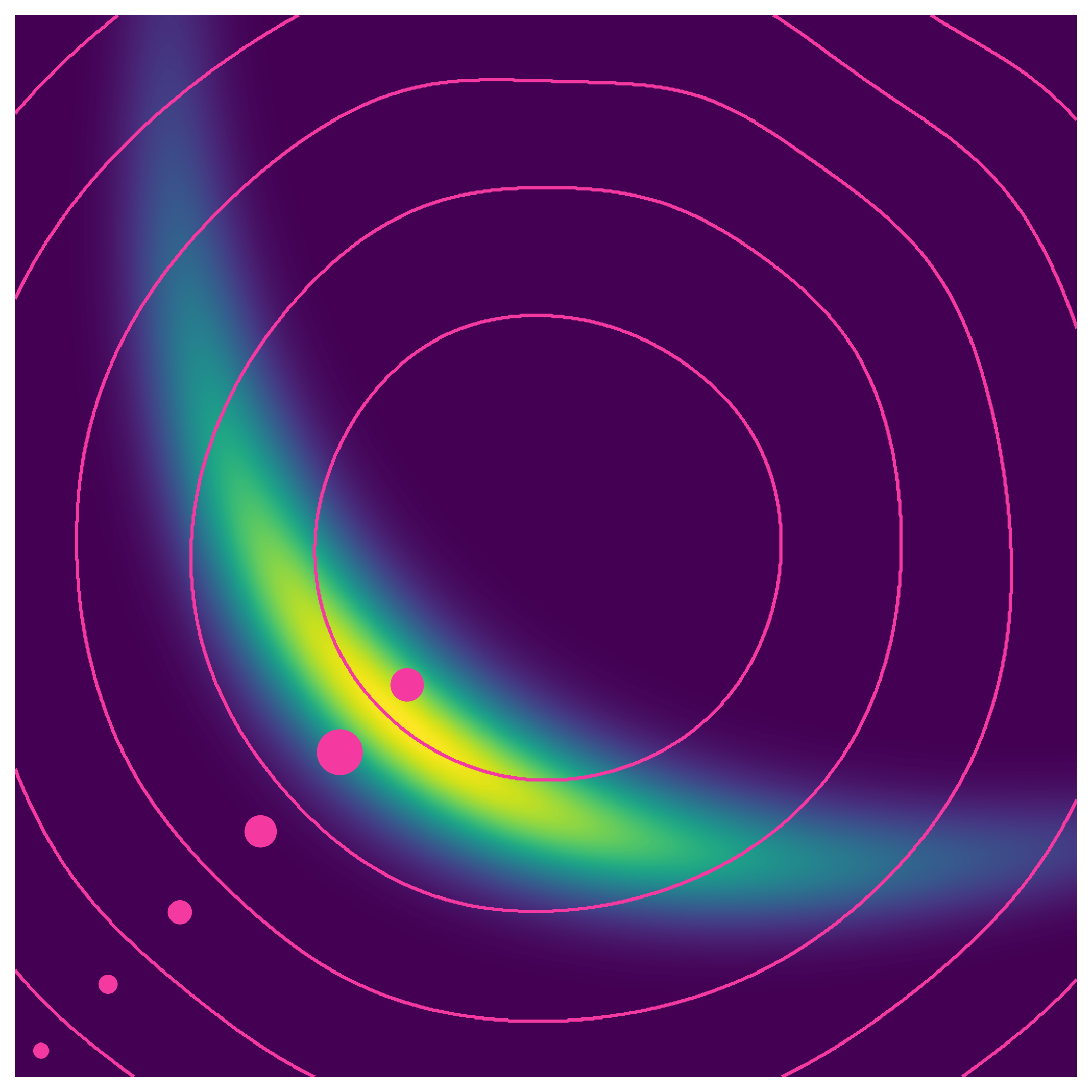
Y. Dubois, B. Bloem-Reddy, K. Ullrich, C. J. Maddison
NeurIPS 2021 Spotlight Presentation πTLDR: We formalize compression with respect to ML algorithms rather than human perception.
Selected Papers Compression, Information Theory, Invariance, Representation Learning, Self-Supervised Learning, VisionCompression
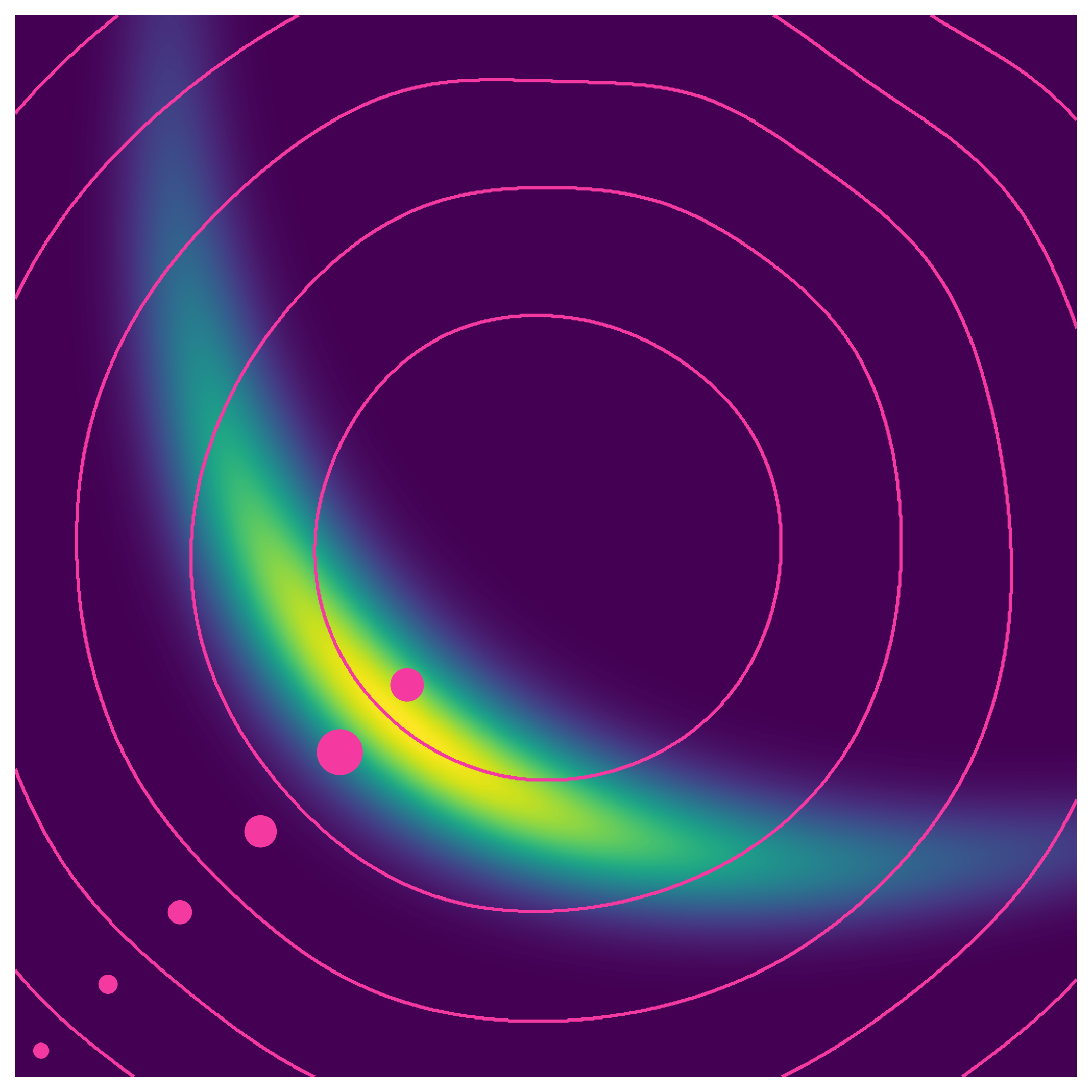
Y. Dubois, B. Bloem-Reddy, K. Ullrich, C. J. Maddison
NeurIPS 2021 Spotlight Presentation πTLDR: We formalize compression with respect to ML algorithms rather than human perception.
Selected Papers Compression, Information Theory, Invariance, Representation Learning, Self-Supervised Learning, VisionEquivariance
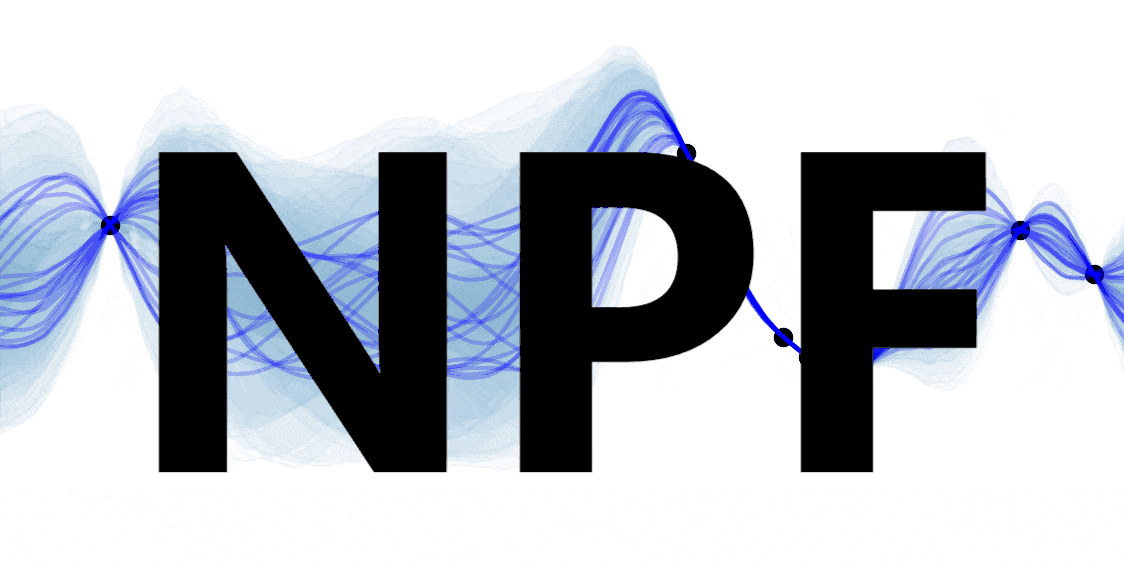
Y. Dubois*, J. Gordon*, A. Foong*
GitHubTLDR: A simple and unifying explanation of the neural process family, which are a collection of models that meta-learn a distribution over predictors.
Equivariance, Neural Processes, Time Series, Uncertainty, Vision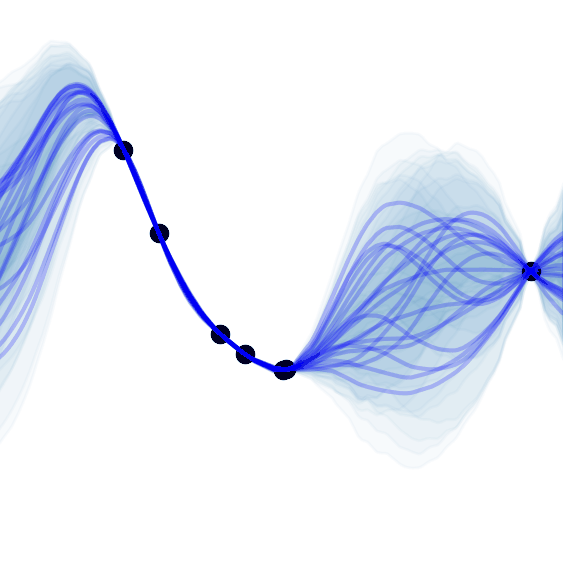
A. Y. K. Foong*, W. P. Bruinsma*, J. Gordon*, Y. Dubois, J. Requeima, R. E. Turner
NeurIPS 2020TLDR: We propose a translation equivariant (latent) neural process.
Equivariance, Neural Processes, Time Series, Uncertainty, Vision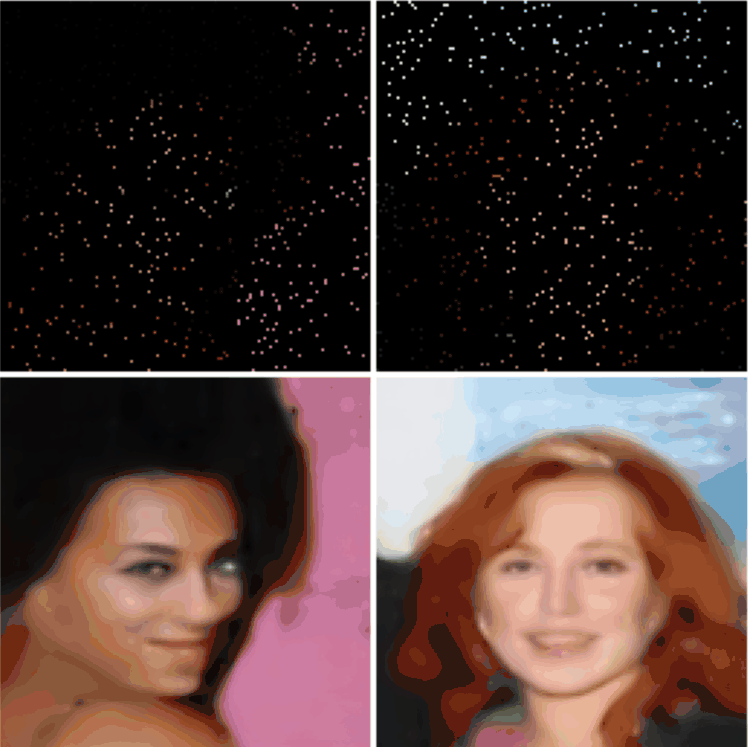
J. Gordon*, W. P. Bruinsma*, A. Y. K. Foong, J. Requeima,Y. Dubois, R. E. Turner
ICLR 2020 Oral Presentation πTLDR: We propose a translation equivariant conditional neural process.
Equivariance, Neural Processes, Time Series, Uncertainty, VisionEvaluation
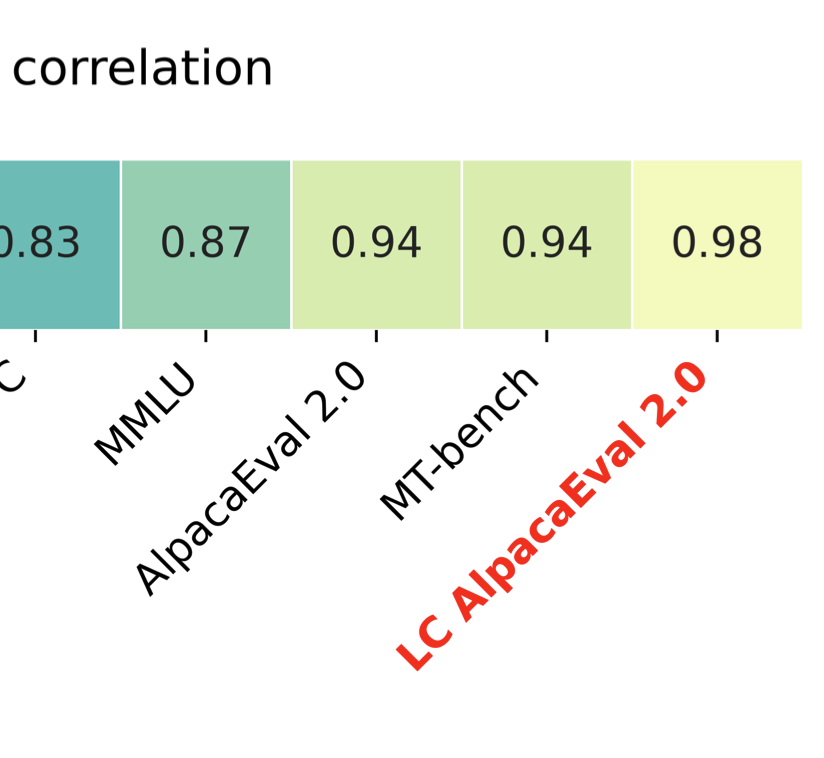
Y. Dubois, B. Galambosi, P. Liang, T. Hashimoto
COLM 2024TLDR: We decrease the bias of AlpacaEval for longer outputs using regression analysis.
Evaluation, NLP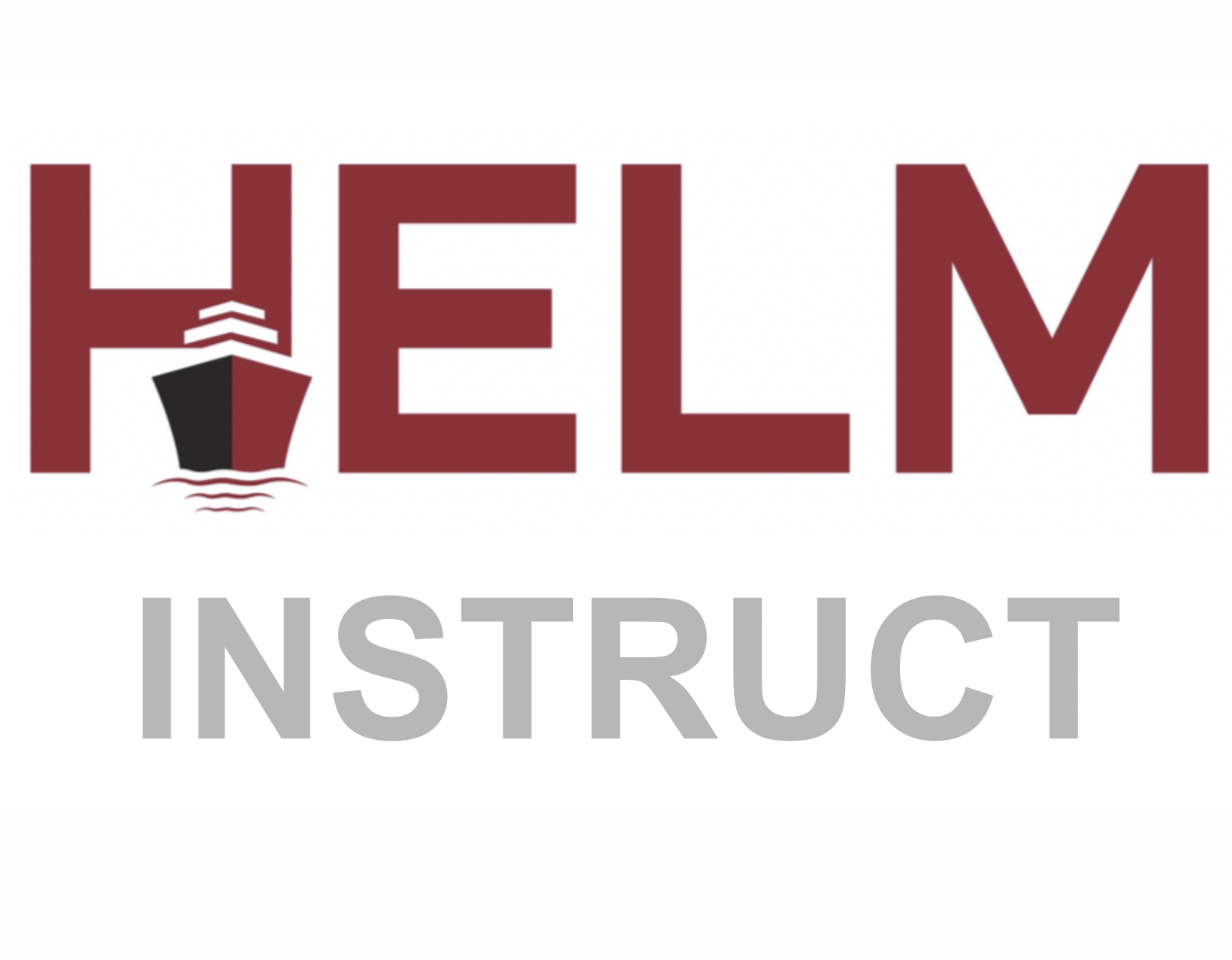
Y. Zhang, Y. Mai, J. Somerville Roberts, R. Bommasani, Y. Dubois, P. Liang
GitHubTLDR: Multidimensional evaluation of instruction following LLM with absolute scores.
Evaluation, NLP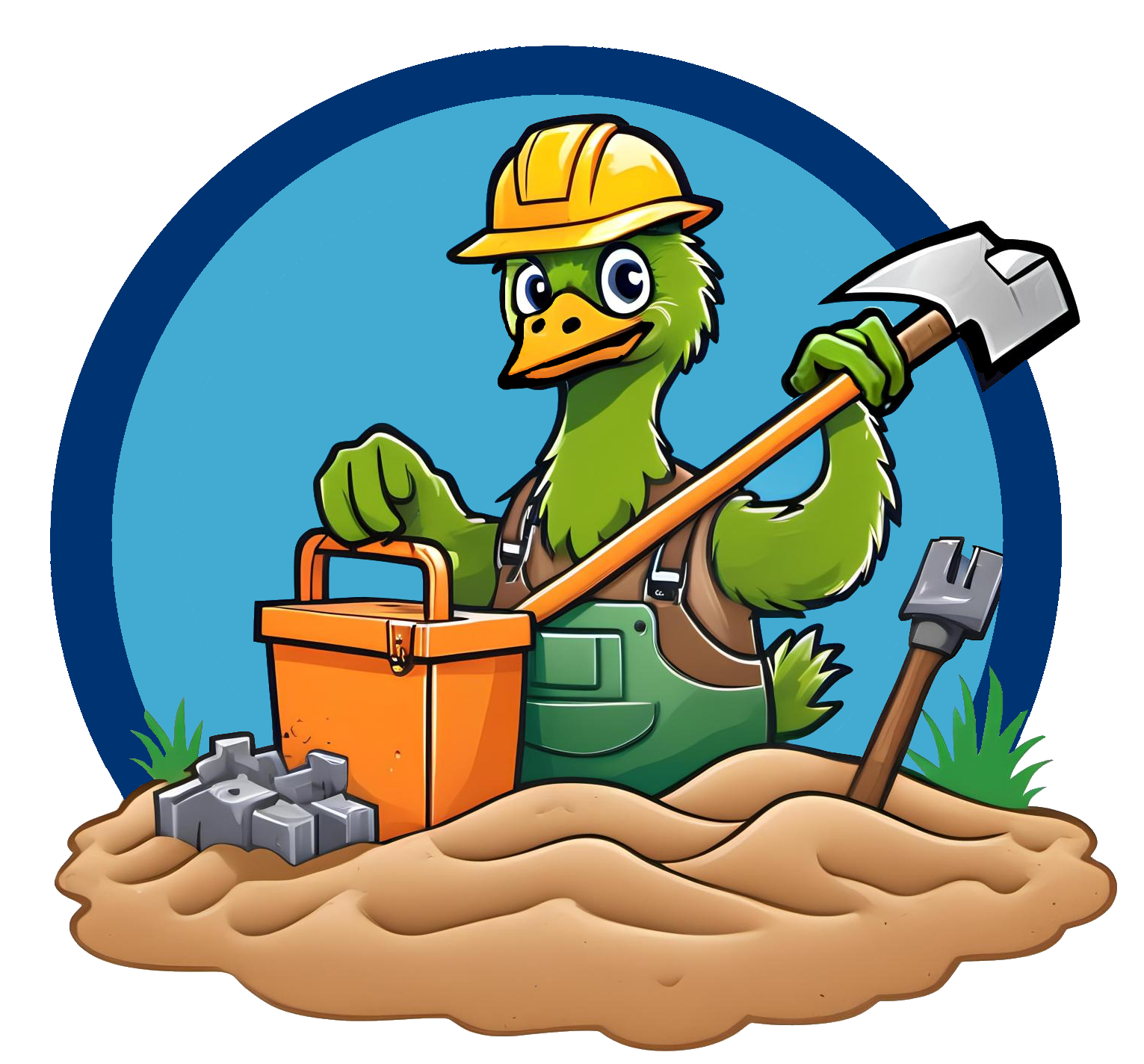
Y. Ruan*, H. Dong*, A. Wang, S. Pitis, Y. Zhou, J. Ba, Y. Dubois, C. J. Maddison, T. Hashimoto
ICLR 2024 Spotlight Presentation πTLDR: An LM-based emulation framework for identifying the risks of LM agents at scale.
Selected Papers Evaluation, NLP, Safety,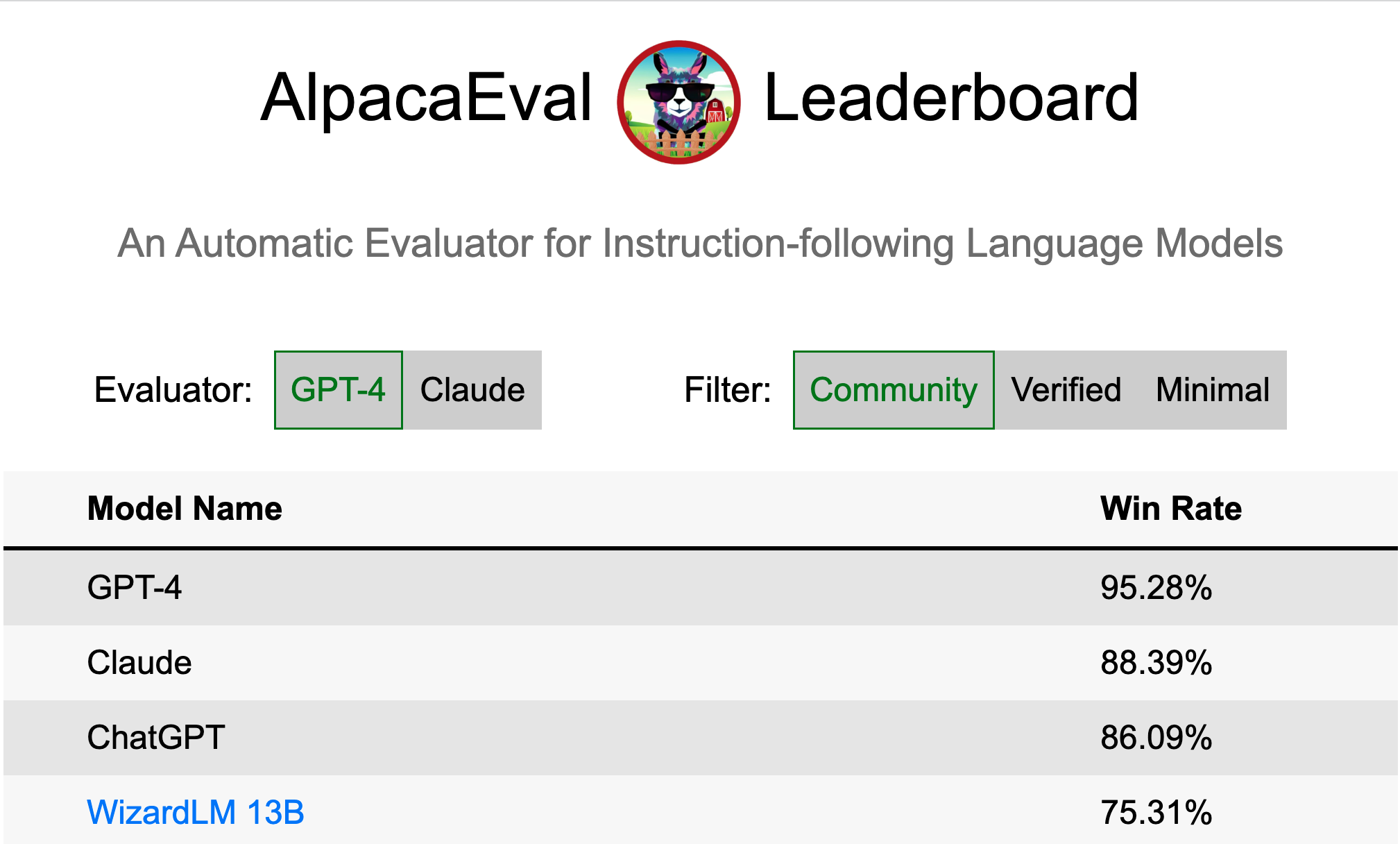
X. Li*, T. Zhang*, Y. Dubois*, R. Taori*, I. Gulrajani, C. Guestrin, P. Liang, T. Hashimoto
GitHubTLDR: A validated automatic evaluator for instruction-following language models. High-quality, cheap, and fast.
Selected Papers Evaluation, NLP, RLHF,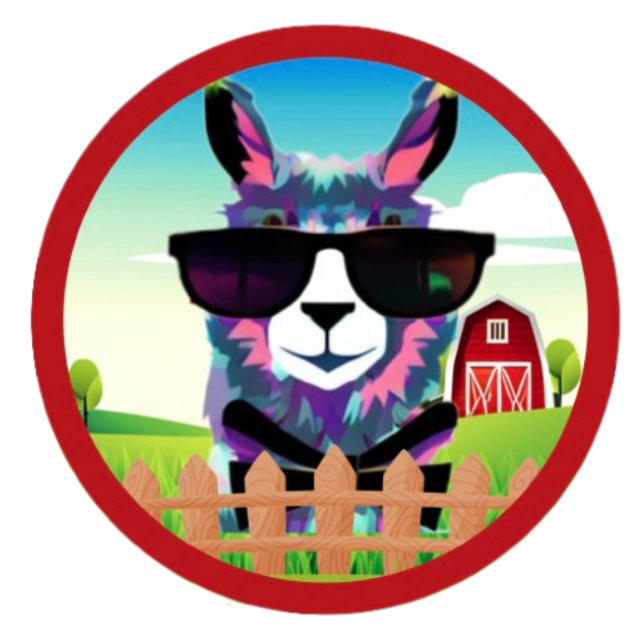
Y. Dubois*, X. Li*, R. Taori*, T. Zhang*, I. Gulrajani, J. Ba, C. Guestrin, P. Liang, T. Hashimoto
NeurIPS 2023 Spotlight Presentation πTLDR: AlpacaFarm replicates the RLHF process at a fraction of the time (<24h) and cost ($<200), enabling the research community to advance instruction following research.
Selected Papers Evaluation, NLP, RLHF,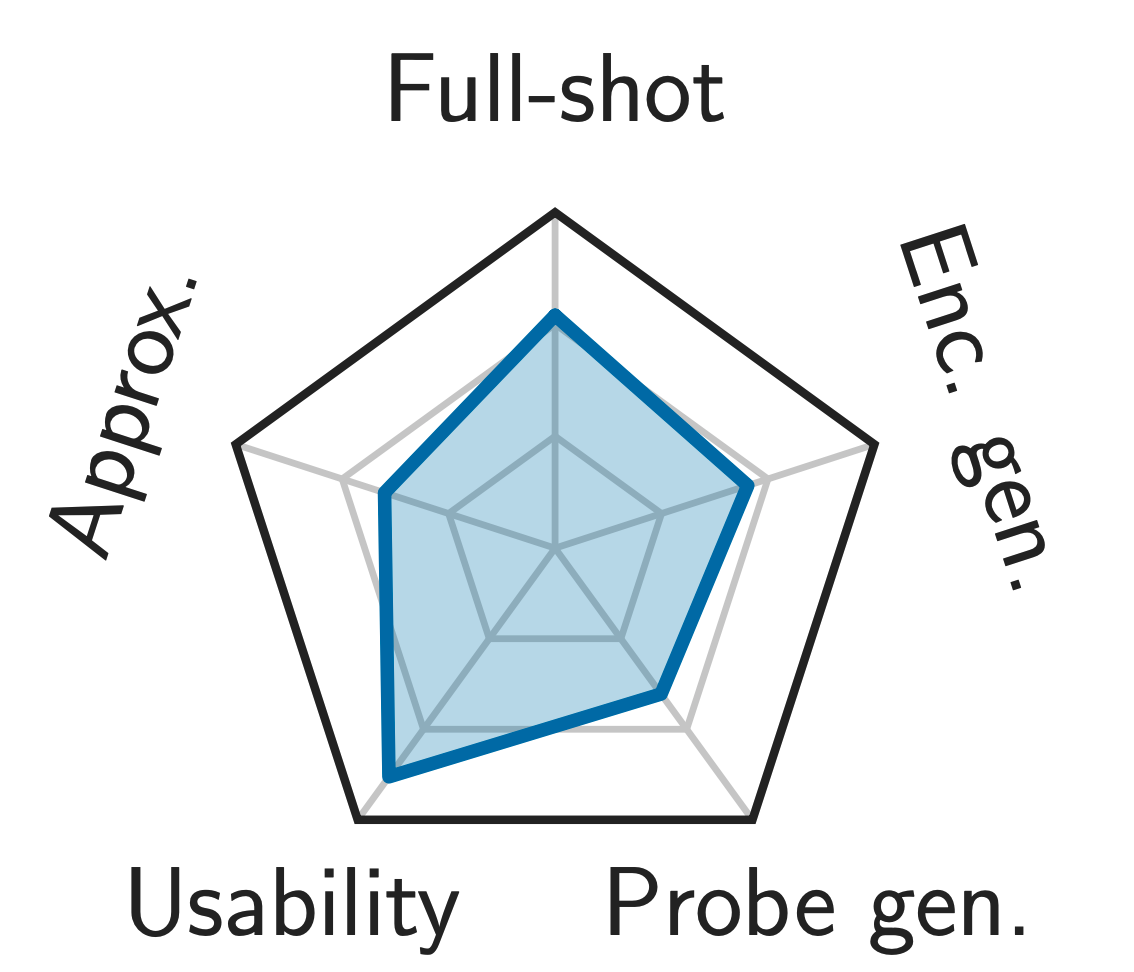
Y. Dubois, T. Hashimoto, P. Liang
ICML 2023 Oral Presentation πTLDR: We derive a risk decomposition for self-supervised learning and use it to evaluate 169 pretrained models.
Selected Papers Evaluation, Representation Learning, Self-Supervised Learning, VisionInformation Theory
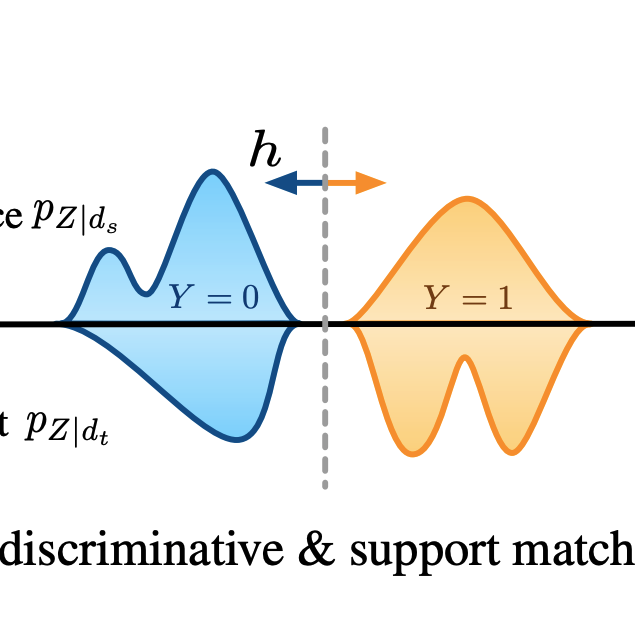
Y Ruan*, Y. Dubois*, C. J. Maddison
ICLR 2021TLDR: We give a simple variational objective whose optima are exactly the set of representations that are robust under covariate shift.
Information Theory, Invariance, Representation Learning, Robustness, Self-Supervised Learning, Vision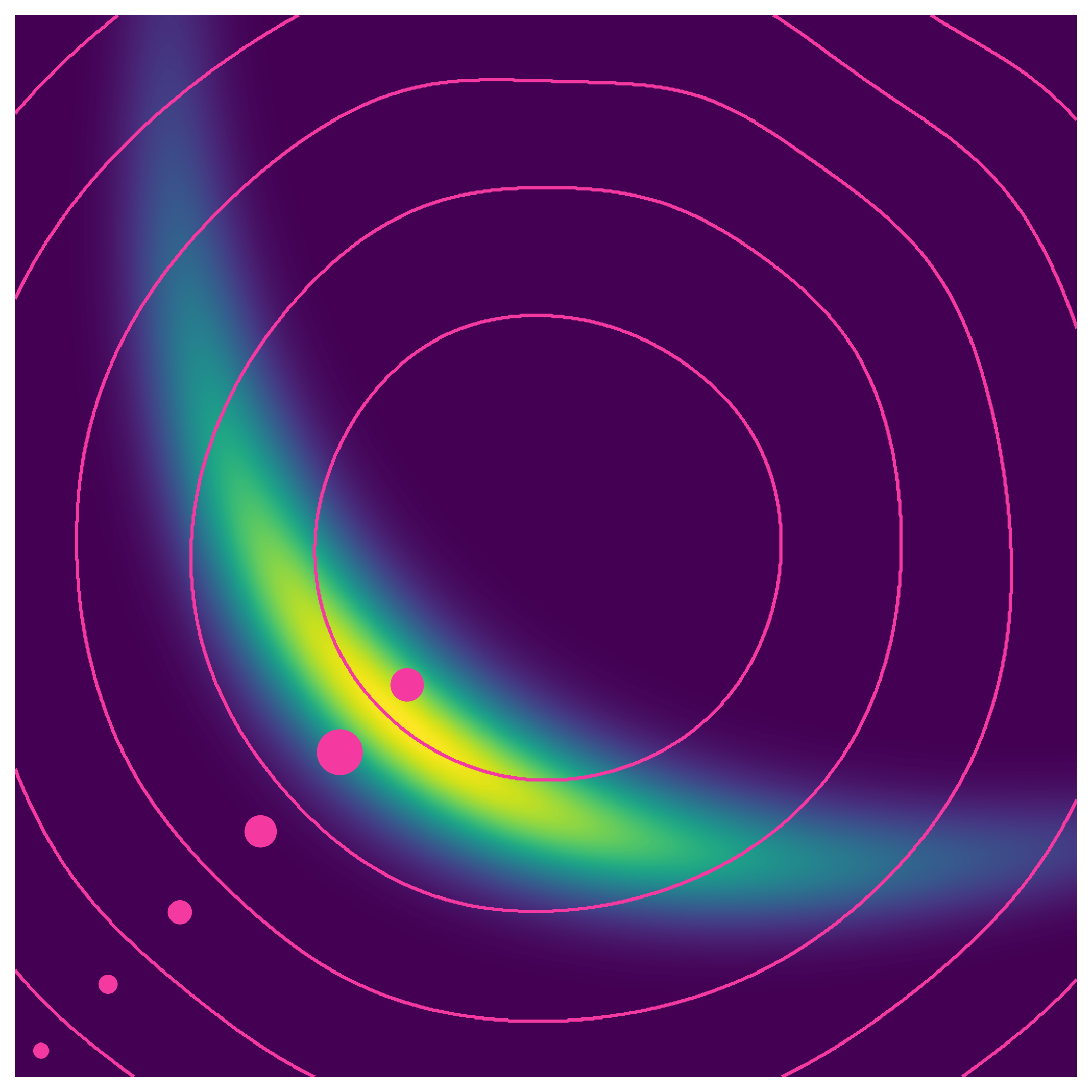
Y. Dubois, B. Bloem-Reddy, K. Ullrich, C. J. Maddison
NeurIPS 2021 Spotlight Presentation πTLDR: We formalize compression with respect to ML algorithms rather than human perception.
Selected Papers Compression, Information Theory, Invariance, Representation Learning, Self-Supervised Learning, Vision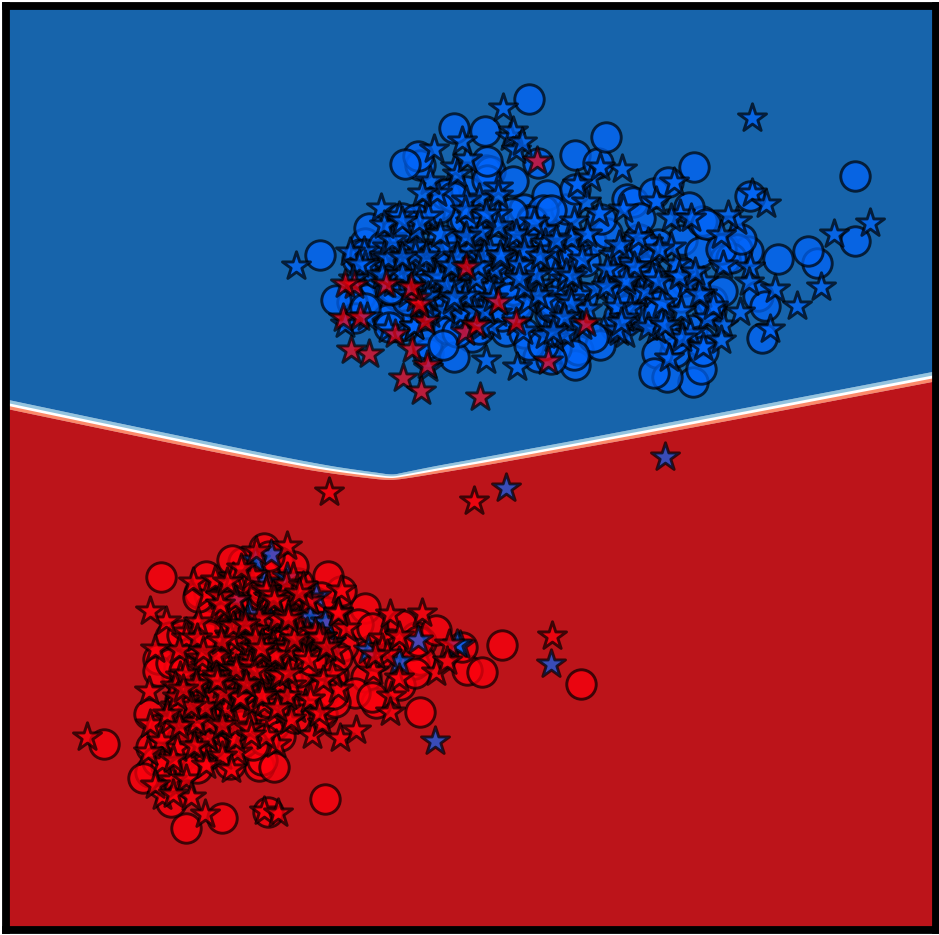
Y. Dubois, D. Kiela, D. J. Schwab, R. Vedantam
NeurIPS 2020 Spotlight Presentation πTLDR: We characterize and approximate optimal representations for supervised learning.
Information Theory, Representation Learning, VisionInvariance
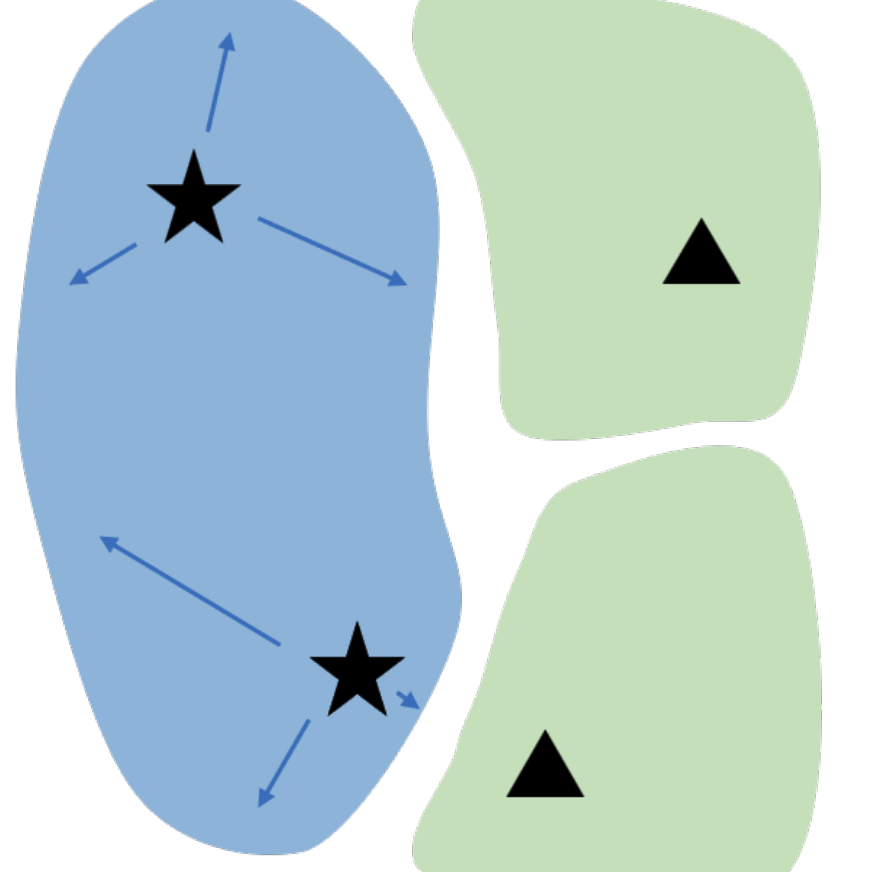
N. Miao, E. Mathieu, Y. Dubois, T. Rainforth, Y. W. Teh, A. Foster, H. Kim
ICML 2023TLDR: We introduce a method for automatically learning input-specific augmentations from data.
Invariance, Vision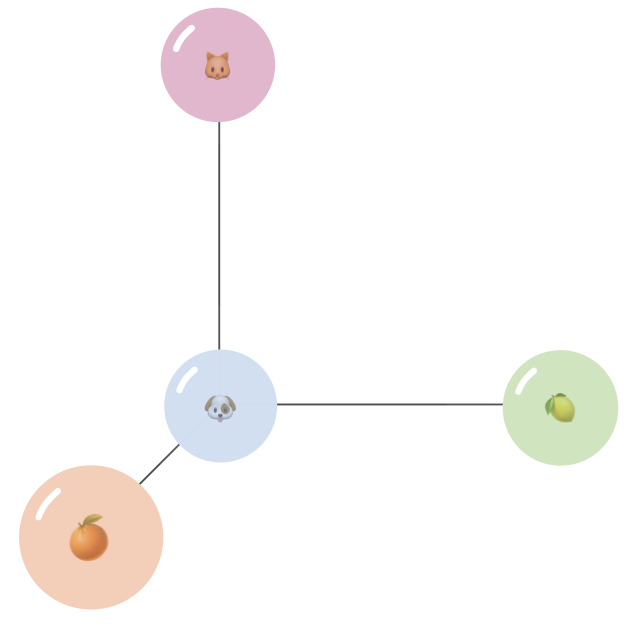
Y. Dubois, T. Hashimoto, S. Ermon, P. Liang
NeurIPS 2022TLDR: We characterize idealized self-supervised representations, which leads to actionable insights for improving SSL algorithms.
Selected Papers Invariance, Representation Learning, Self-Supervised Learning, Vision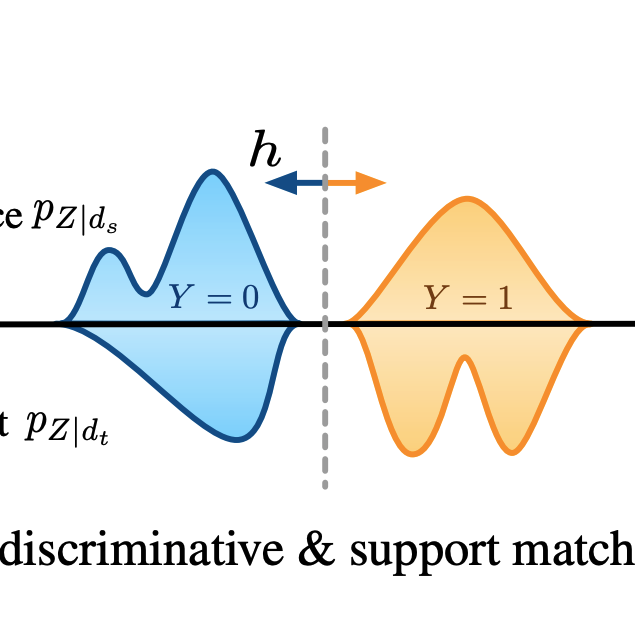
Y Ruan*, Y. Dubois*, C. J. Maddison
ICLR 2021TLDR: We give a simple variational objective whose optima are exactly the set of representations that are robust under covariate shift.
Information Theory, Invariance, Representation Learning, Robustness, Self-Supervised Learning, Vision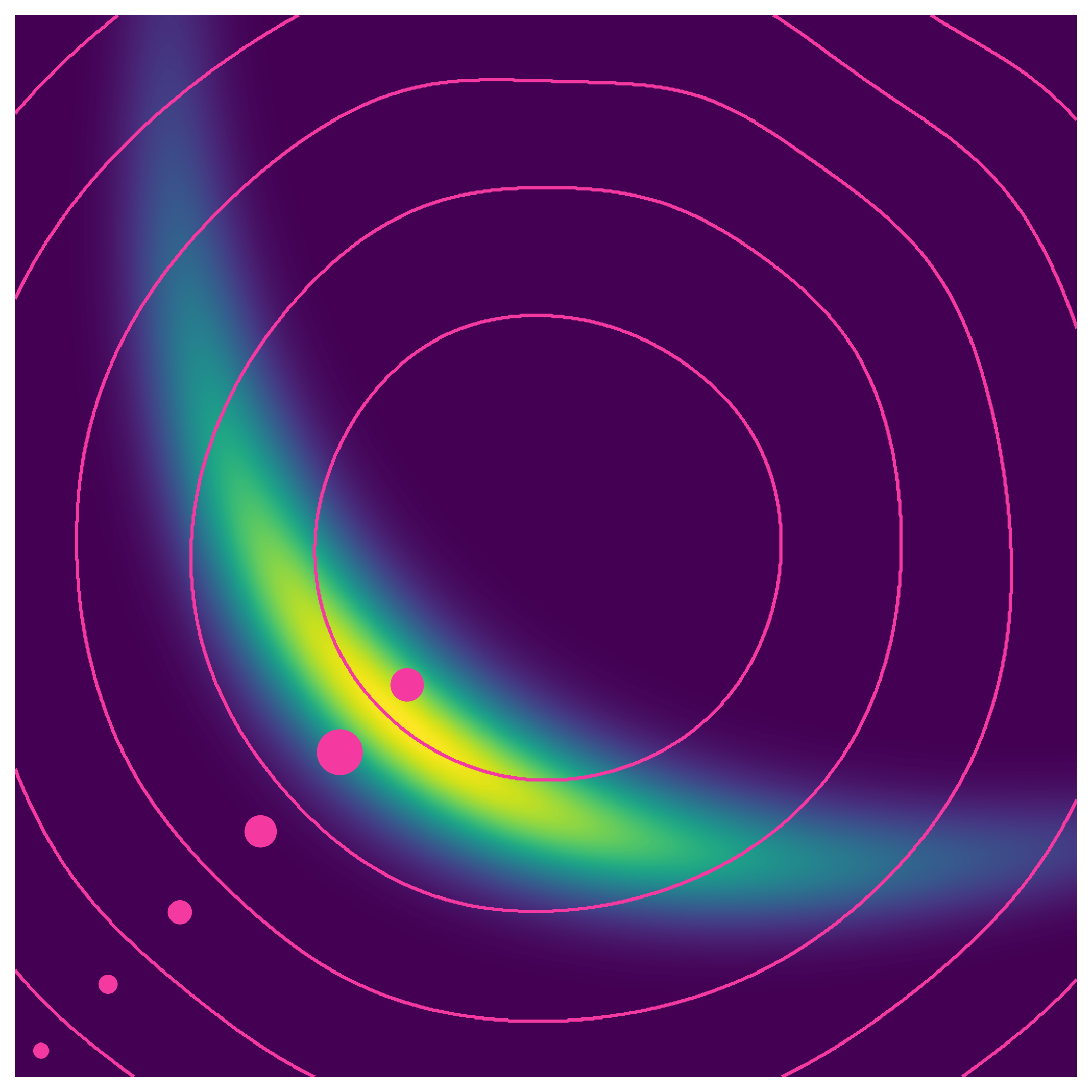
Y. Dubois, B. Bloem-Reddy, K. Ullrich, C. J. Maddison
NeurIPS 2021 Spotlight Presentation πTLDR: We formalize compression with respect to ML algorithms rather than human perception.
Selected Papers Compression, Information Theory, Invariance, Representation Learning, Self-Supervised Learning, VisionNLP
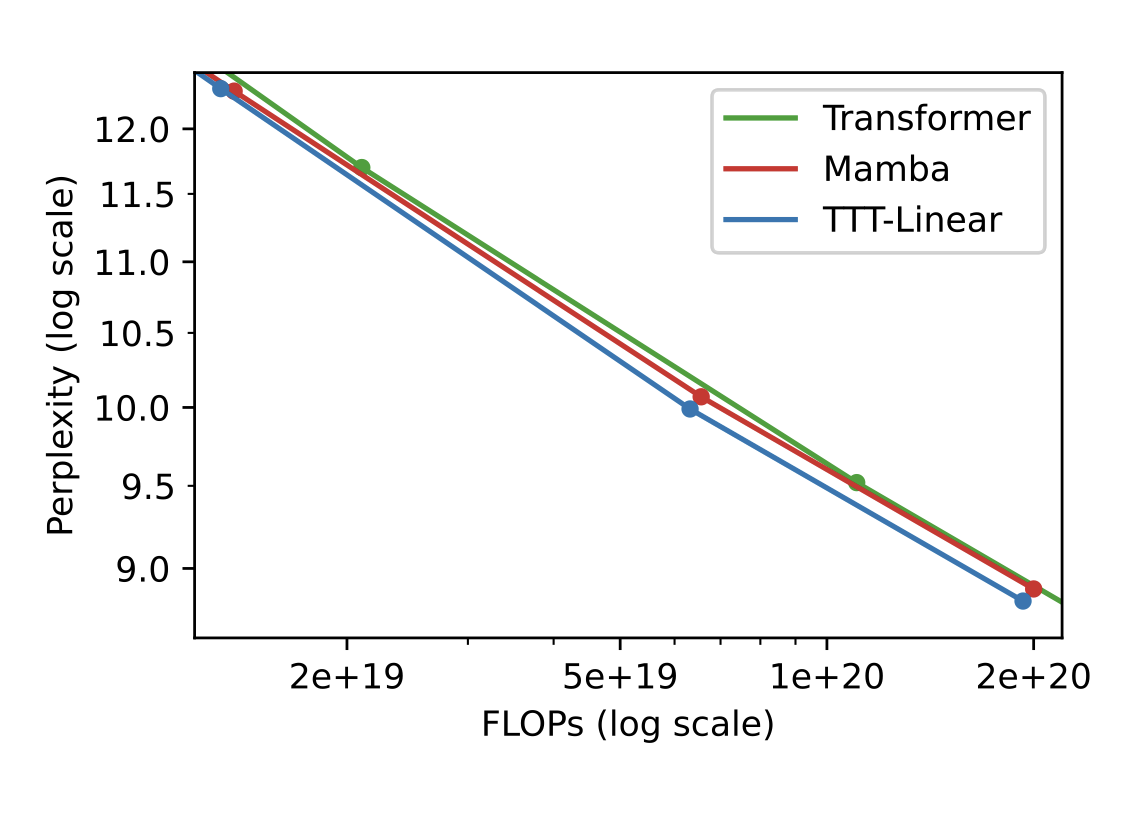
Yu Sun, Xinhao Li, Karan Dalal, Jiarui Xu, Arjun Vikram, Genghan Zhang, Y. Dubois, Xinlei Chen, Xiaolong Wang, Sanmi Koyejo, Tatsunori Hashimoto, Carlos Guestrin
ArXivTLDR: A new language model layer that is more expressive than RNN but more efficient than attention.
NLP, Self-Supervised Learning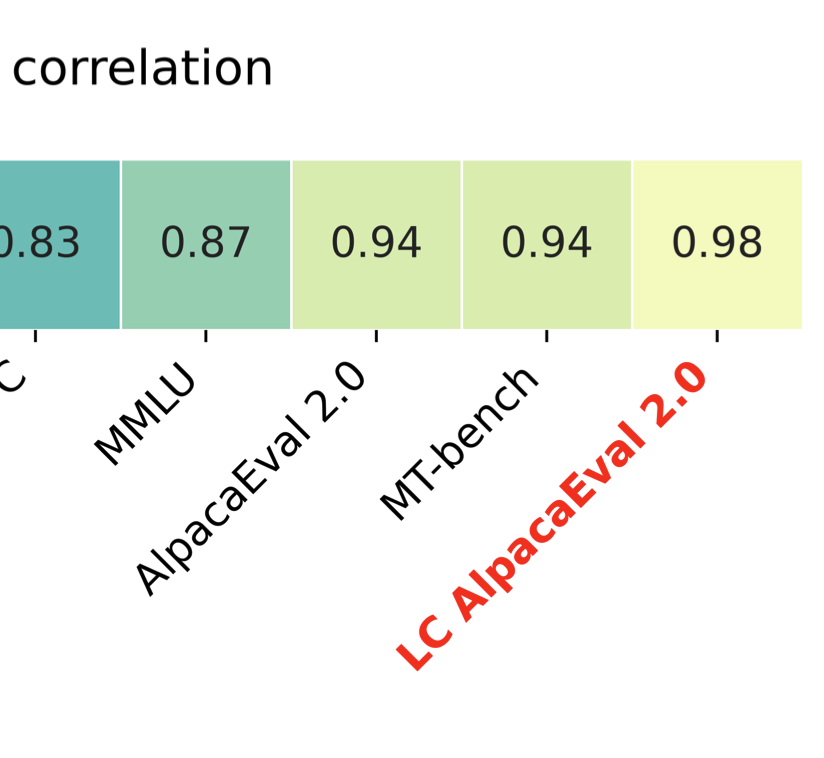
Y. Dubois, B. Galambosi, P. Liang, T. Hashimoto
COLM 2024TLDR: We decrease the bias of AlpacaEval for longer outputs using regression analysis.
Evaluation, NLP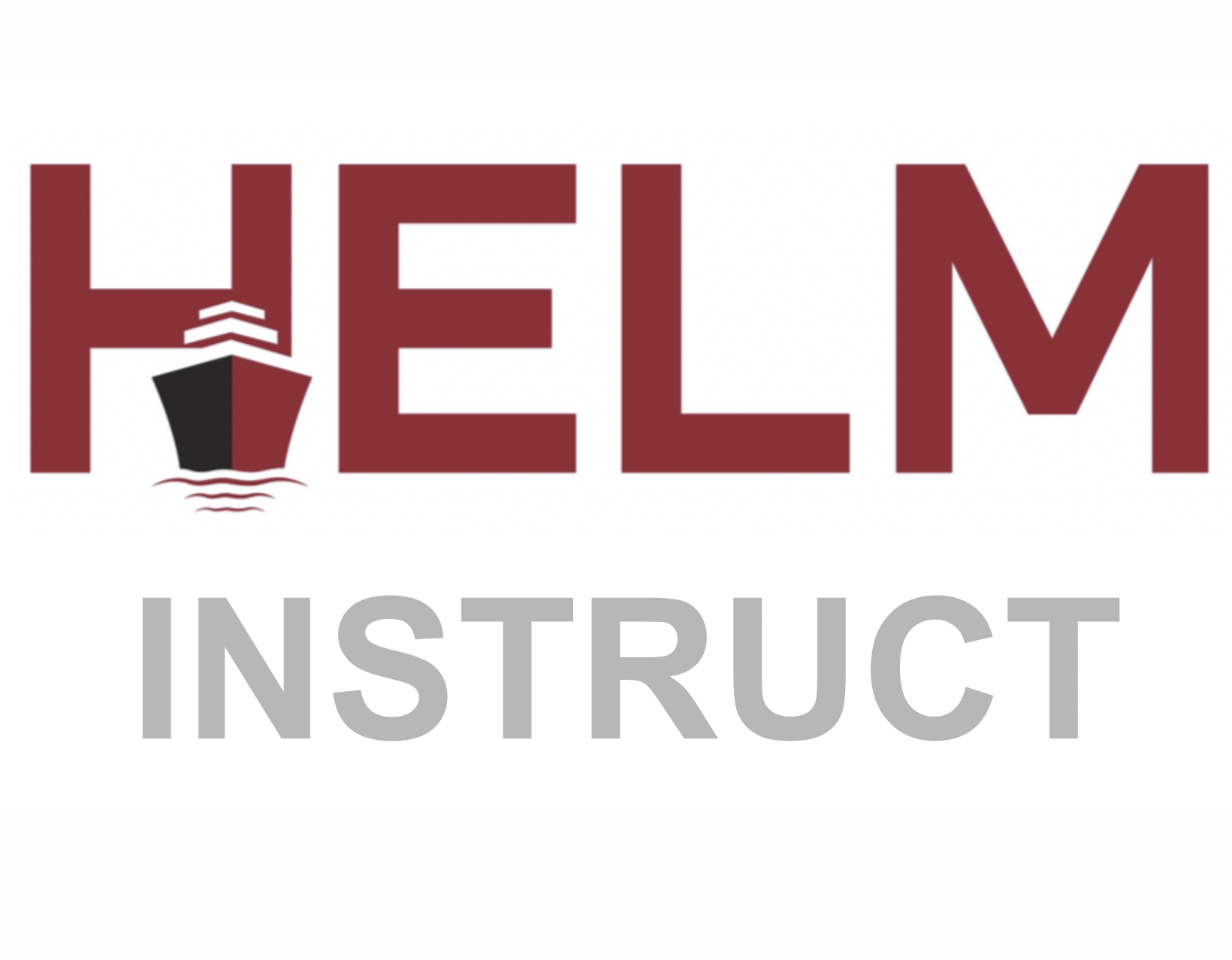
Y. Zhang, Y. Mai, J. Somerville Roberts, R. Bommasani, Y. Dubois, P. Liang
GitHubTLDR: Multidimensional evaluation of instruction following LLM with absolute scores.
Evaluation, NLP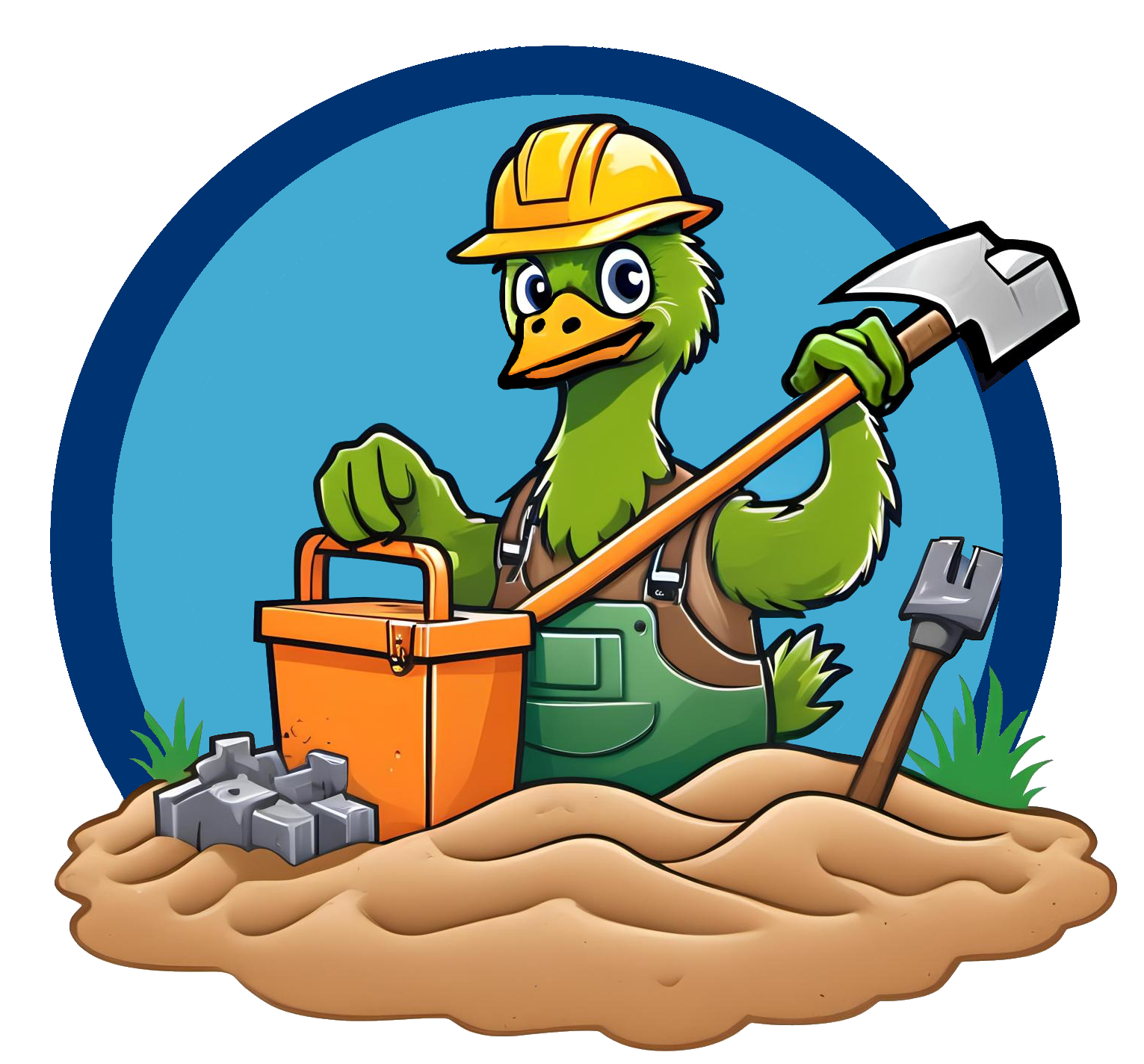
Y. Ruan*, H. Dong*, A. Wang, S. Pitis, Y. Zhou, J. Ba, Y. Dubois, C. J. Maddison, T. Hashimoto
ICLR 2024 Spotlight Presentation πTLDR: An LM-based emulation framework for identifying the risks of LM agents at scale.
Selected Papers Evaluation, NLP, Safety,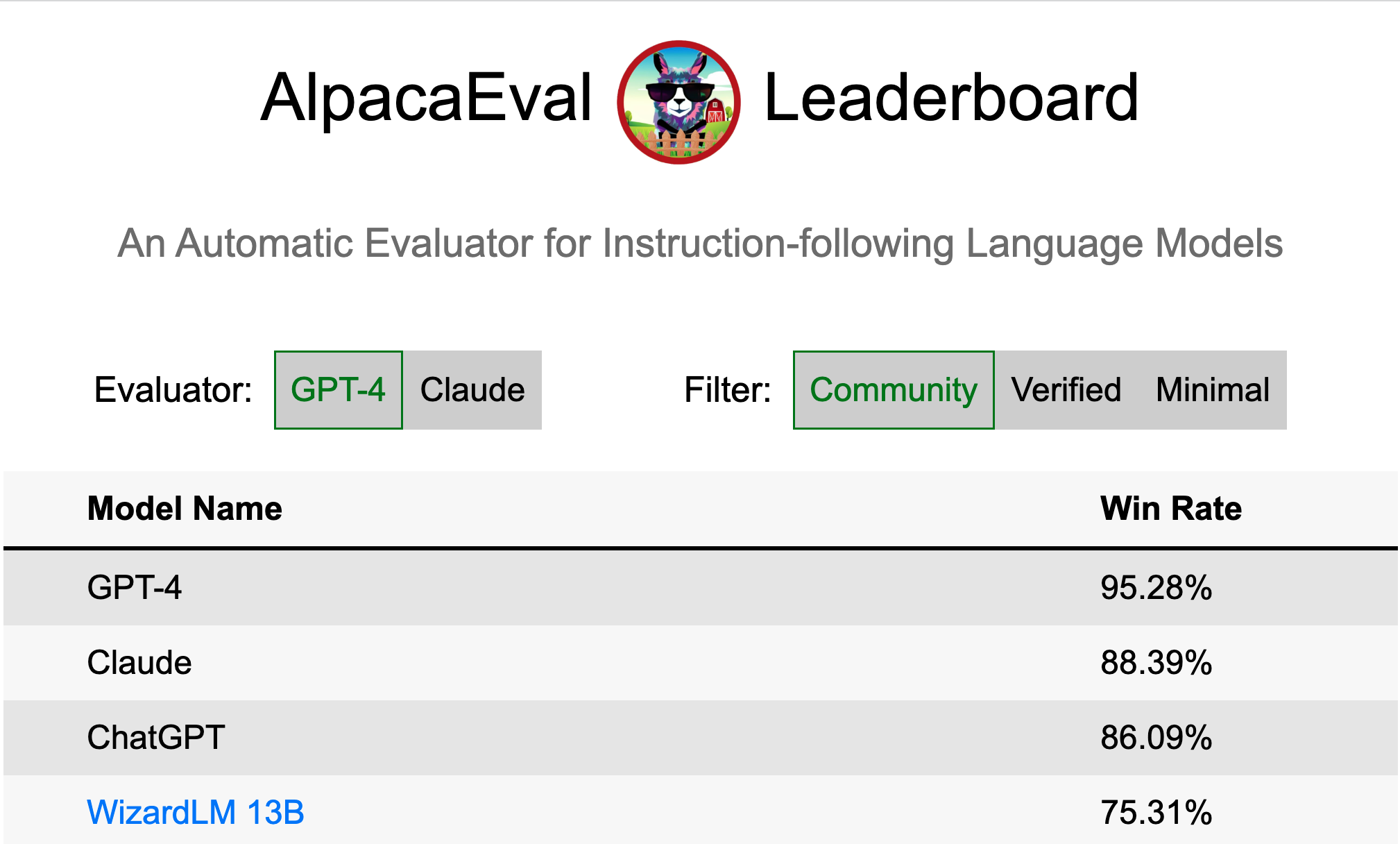
X. Li*, T. Zhang*, Y. Dubois*, R. Taori*, I. Gulrajani, C. Guestrin, P. Liang, T. Hashimoto
GitHubTLDR: A validated automatic evaluator for instruction-following language models. High-quality, cheap, and fast.
Selected Papers Evaluation, NLP, RLHF,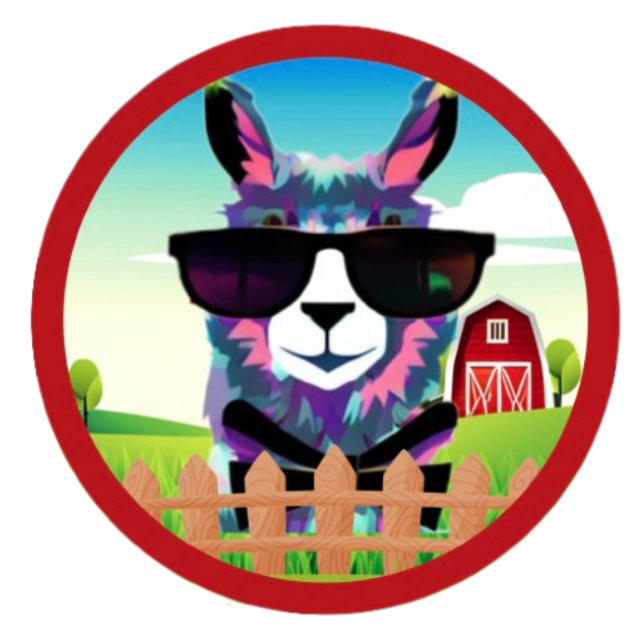
Y. Dubois*, X. Li*, R. Taori*, T. Zhang*, I. Gulrajani, J. Ba, C. Guestrin, P. Liang, T. Hashimoto
NeurIPS 2023 Spotlight Presentation πTLDR: AlpacaFarm replicates the RLHF process at a fraction of the time (<24h) and cost ($<200), enabling the research community to advance instruction following research.
Selected Papers Evaluation, NLP, RLHF,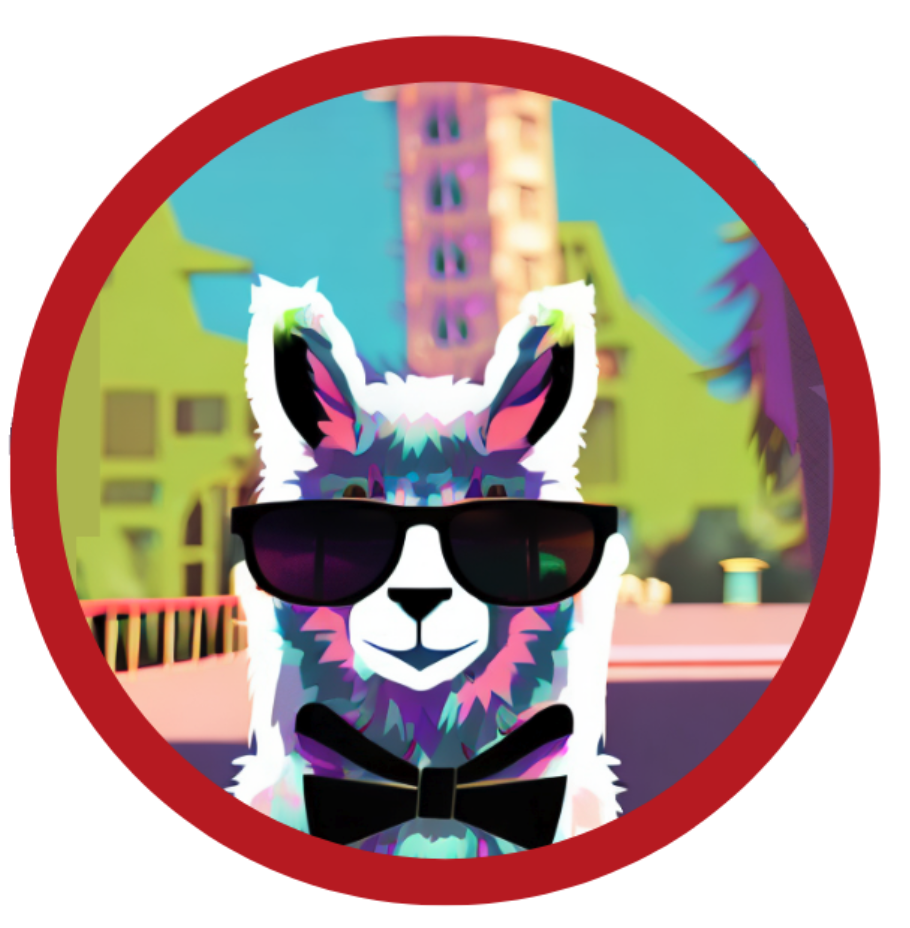
R. Taori*, I. Gulrajani*, T. Zhang*, Y. Dubois*, X. Li*, C. Guestrin, P. Liang, T. Hashimoto
GitHubTLDR: We introduce Alpaca 7B, a instruction-following fine-tuned LLaMA model. On our preliminary evaluation, Alpaca behaves qualitatively similarly to OpenAIβs text-davinci-003, while being surprisingly small and easy/cheap to reproduce (<600$).
Selected Papers NLP, RLHF, Self-Supervised Learning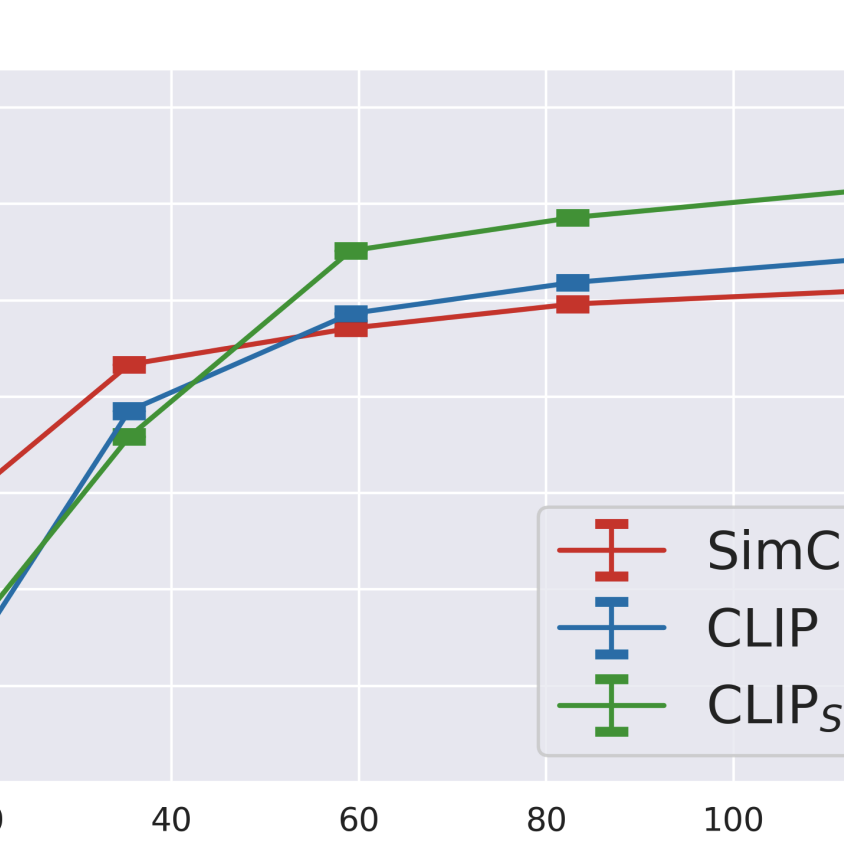
S. Santurkar, Y. Dubois, R. Taori, P. Liang, T. Hashimoto
ICLR 2022TLDR: Our work performs a systematic investigation into whether additional language supervision (in CLIP) helps models learn more transferrable representations.
NLP, Representation Learning, Self-Supervised Learning, Vision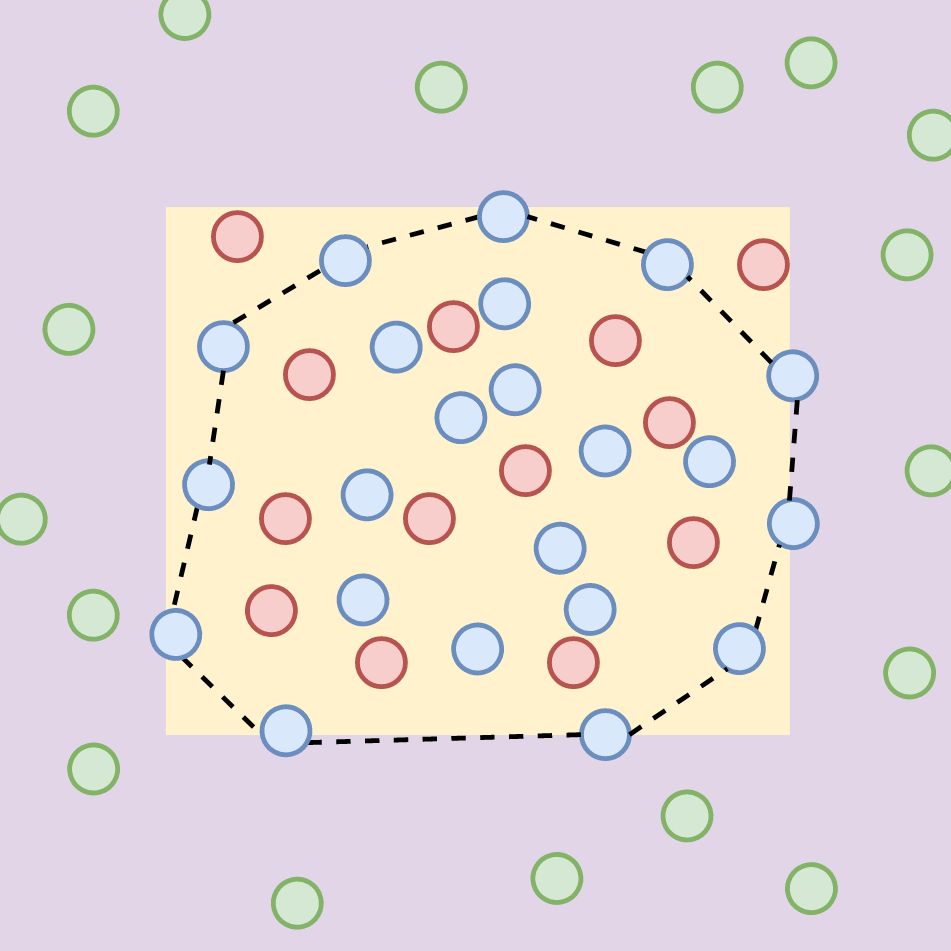
Y. Dubois, Gautier Dagan, Dieuwke Hupkes, Elia Bruni
ACL 2020TLDR: We propose an attention that improves extrapolation capacity of neural NLP models.
NLP, RobustnessNeural Processes
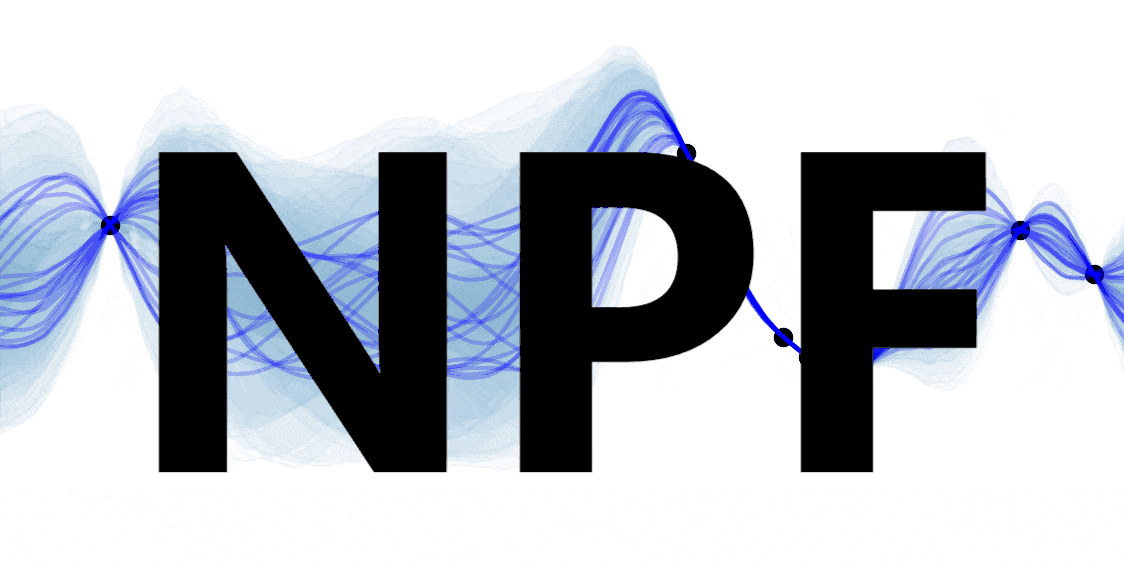
Y. Dubois*, J. Gordon*, A. Foong*
GitHubTLDR: A simple and unifying explanation of the neural process family, which are a collection of models that meta-learn a distribution over predictors.
Equivariance, Neural Processes, Time Series, Uncertainty, Vision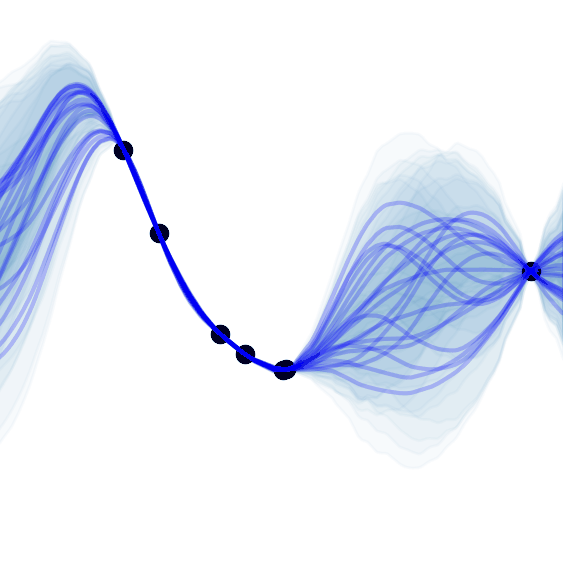
A. Y. K. Foong*, W. P. Bruinsma*, J. Gordon*, Y. Dubois, J. Requeima, R. E. Turner
NeurIPS 2020TLDR: We propose a translation equivariant (latent) neural process.
Equivariance, Neural Processes, Time Series, Uncertainty, Vision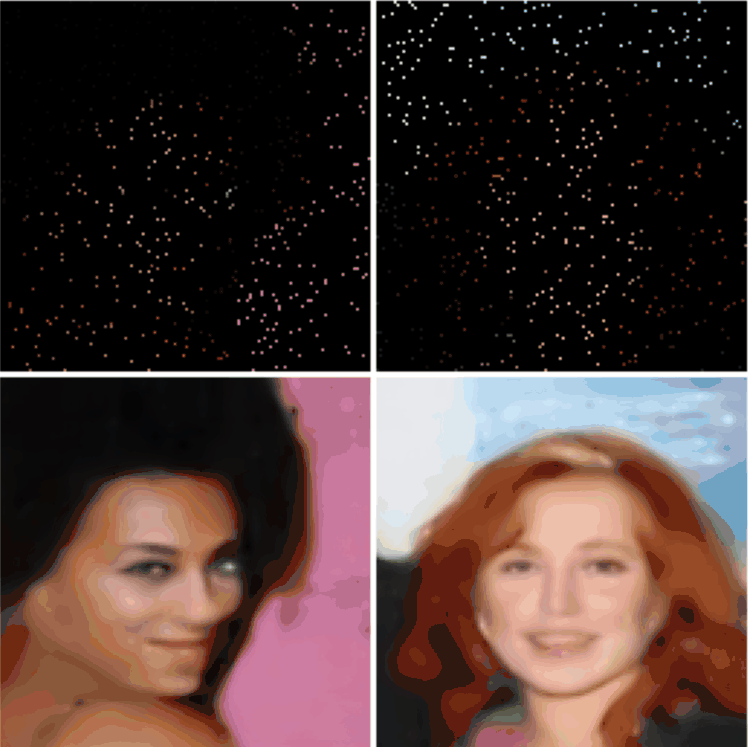
J. Gordon*, W. P. Bruinsma*, A. Y. K. Foong, J. Requeima,Y. Dubois, R. E. Turner
ICLR 2020 Oral Presentation πTLDR: We propose a translation equivariant conditional neural process.
Equivariance, Neural Processes, Time Series, Uncertainty, VisionRLHF
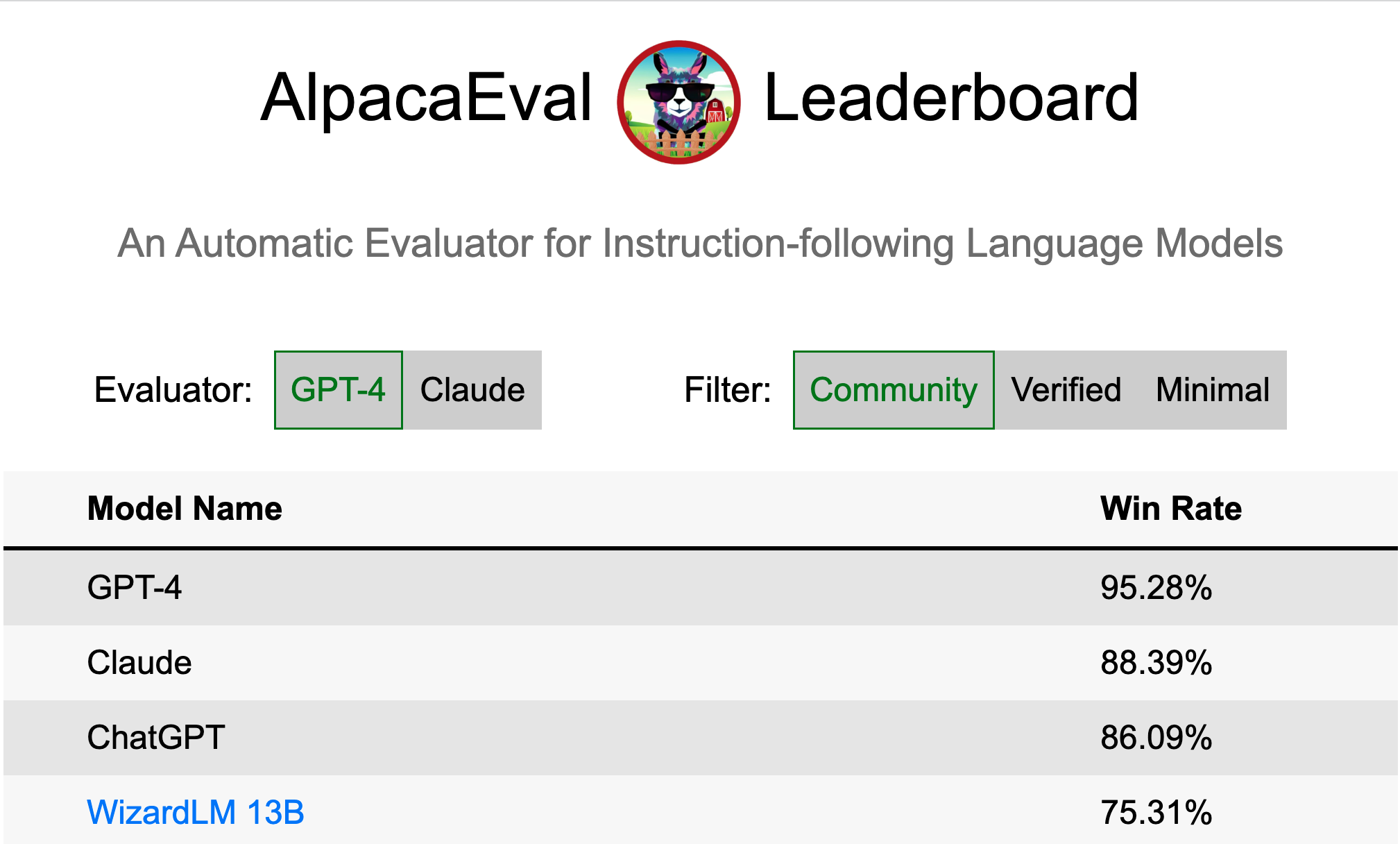
X. Li*, T. Zhang*, Y. Dubois*, R. Taori*, I. Gulrajani, C. Guestrin, P. Liang, T. Hashimoto
GitHubTLDR: A validated automatic evaluator for instruction-following language models. High-quality, cheap, and fast.
Selected Papers Evaluation, NLP, RLHF,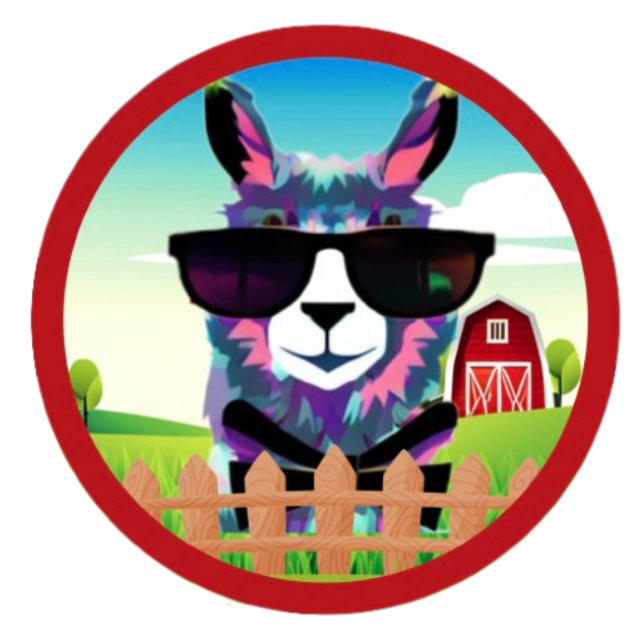
Y. Dubois*, X. Li*, R. Taori*, T. Zhang*, I. Gulrajani, J. Ba, C. Guestrin, P. Liang, T. Hashimoto
NeurIPS 2023 Spotlight Presentation πTLDR: AlpacaFarm replicates the RLHF process at a fraction of the time (<24h) and cost ($<200), enabling the research community to advance instruction following research.
Selected Papers Evaluation, NLP, RLHF,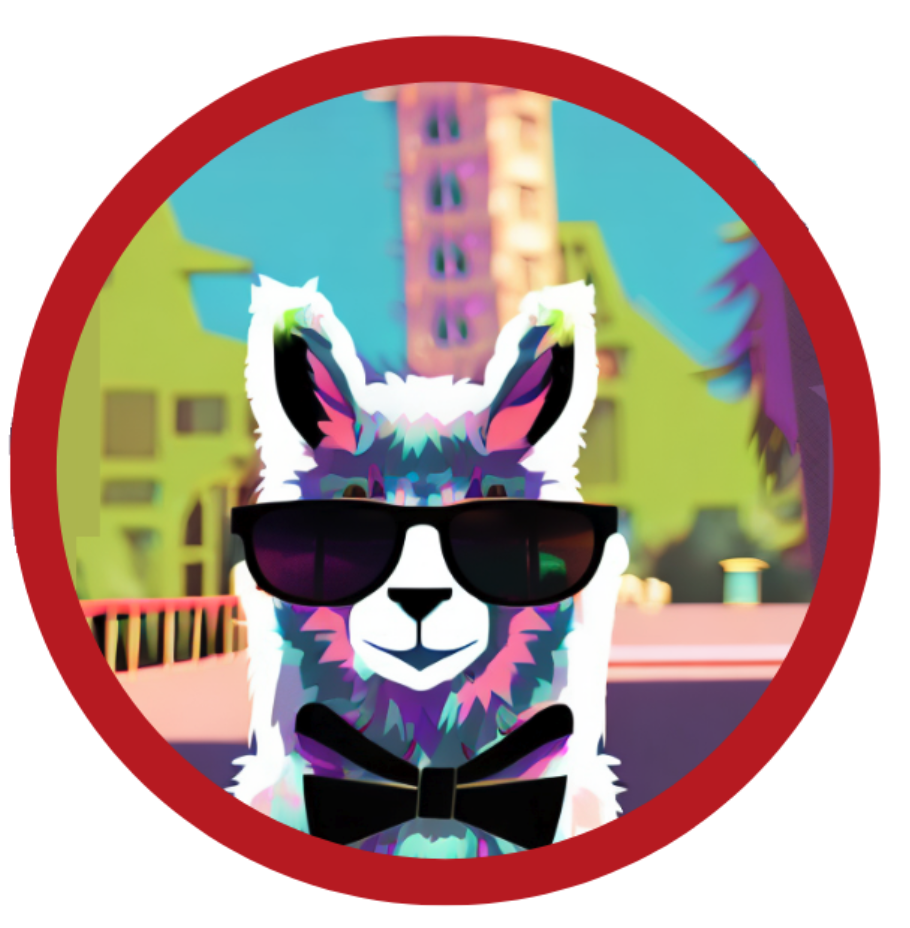
R. Taori*, I. Gulrajani*, T. Zhang*, Y. Dubois*, X. Li*, C. Guestrin, P. Liang, T. Hashimoto
GitHubTLDR: We introduce Alpaca 7B, a instruction-following fine-tuned LLaMA model. On our preliminary evaluation, Alpaca behaves qualitatively similarly to OpenAIβs text-davinci-003, while being surprisingly small and easy/cheap to reproduce (<600$).
Selected Papers NLP, RLHF, Self-Supervised LearningRepresentation Learning
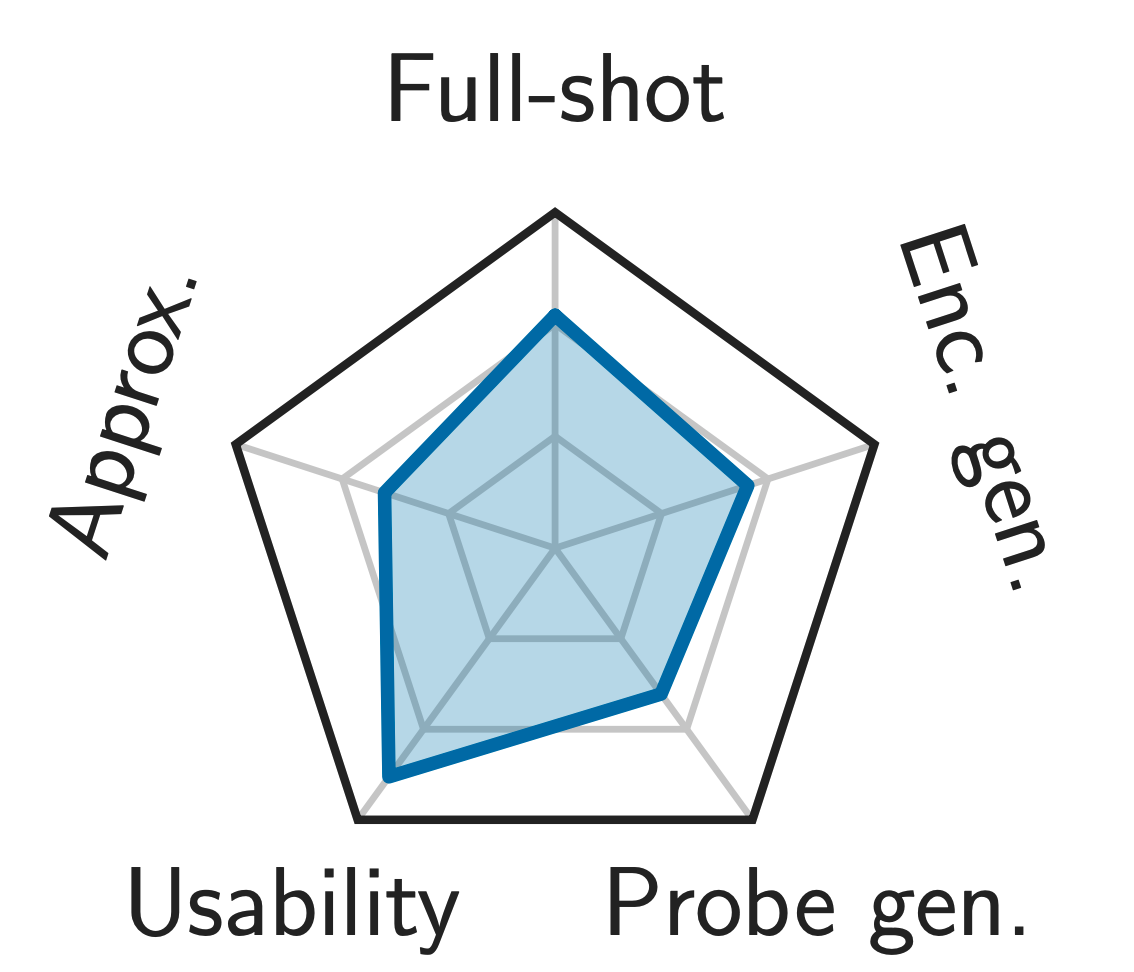
Y. Dubois, T. Hashimoto, P. Liang
ICML 2023 Oral Presentation πTLDR: We derive a risk decomposition for self-supervised learning and use it to evaluate 169 pretrained models.
Selected Papers Evaluation, Representation Learning, Self-Supervised Learning, Vision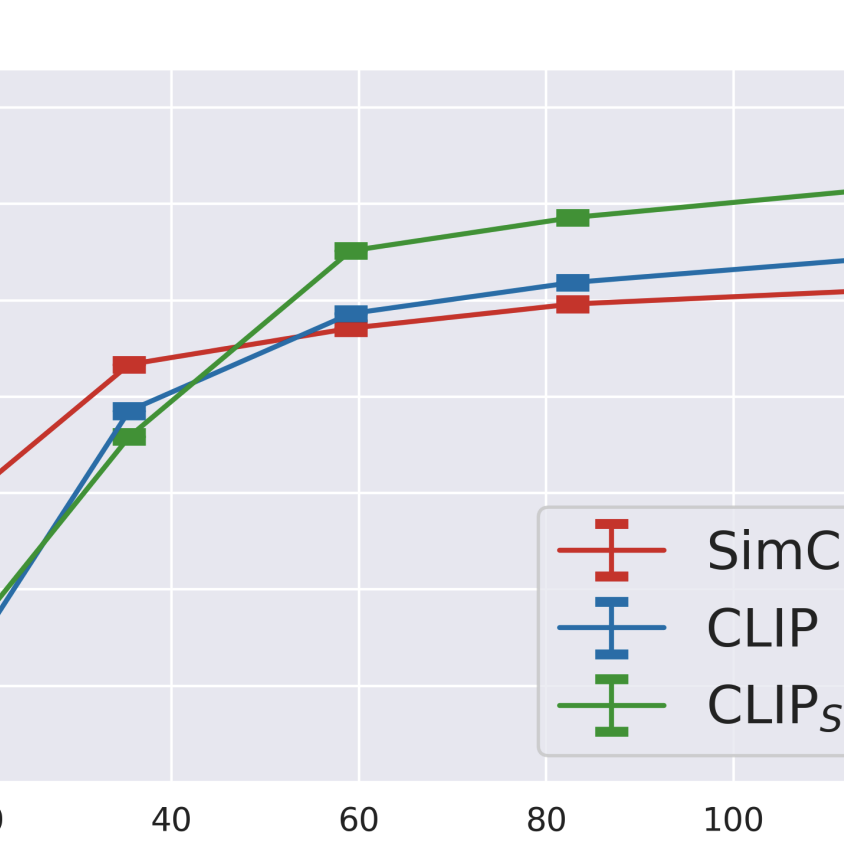
S. Santurkar, Y. Dubois, R. Taori, P. Liang, T. Hashimoto
ICLR 2022TLDR: Our work performs a systematic investigation into whether additional language supervision (in CLIP) helps models learn more transferrable representations.
NLP, Representation Learning, Self-Supervised Learning, Vision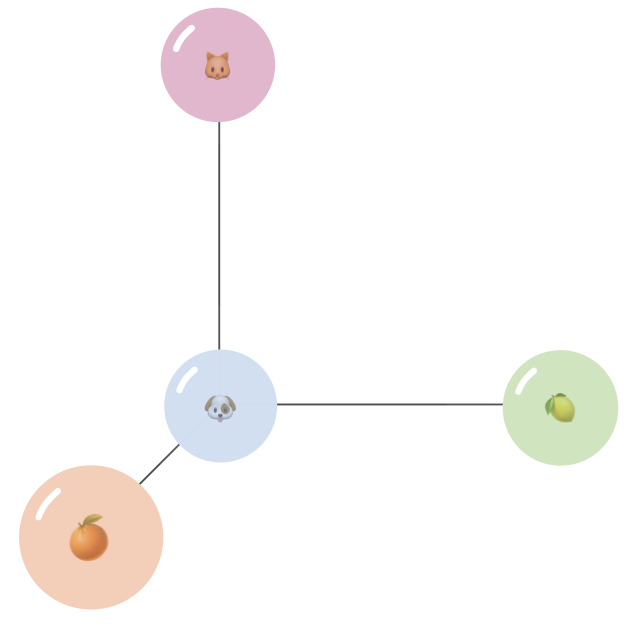
Y. Dubois, T. Hashimoto, S. Ermon, P. Liang
NeurIPS 2022TLDR: We characterize idealized self-supervised representations, which leads to actionable insights for improving SSL algorithms.
Selected Papers Invariance, Representation Learning, Self-Supervised Learning, Vision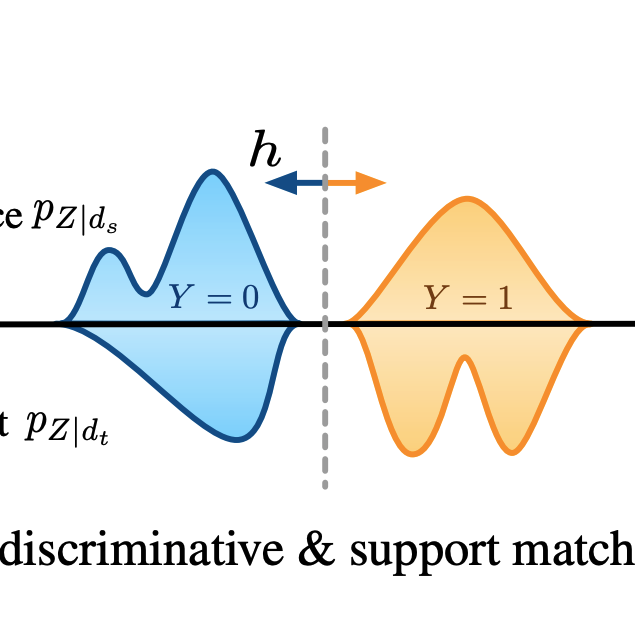
Y Ruan*, Y. Dubois*, C. J. Maddison
ICLR 2021TLDR: We give a simple variational objective whose optima are exactly the set of representations that are robust under covariate shift.
Information Theory, Invariance, Representation Learning, Robustness, Self-Supervised Learning, Vision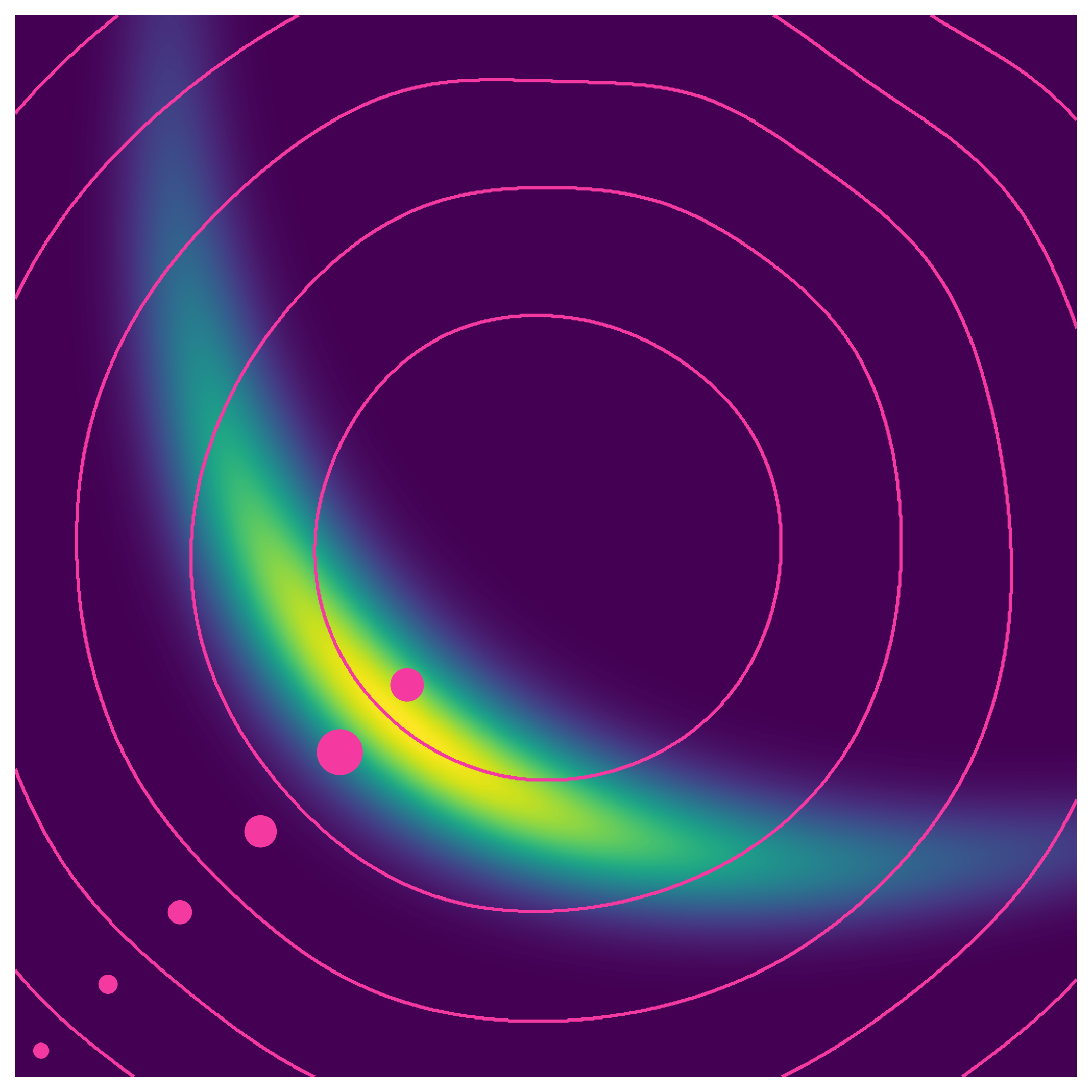
Y. Dubois, B. Bloem-Reddy, K. Ullrich, C. J. Maddison
NeurIPS 2021 Spotlight Presentation πTLDR: We formalize compression with respect to ML algorithms rather than human perception.
Selected Papers Compression, Information Theory, Invariance, Representation Learning, Self-Supervised Learning, Vision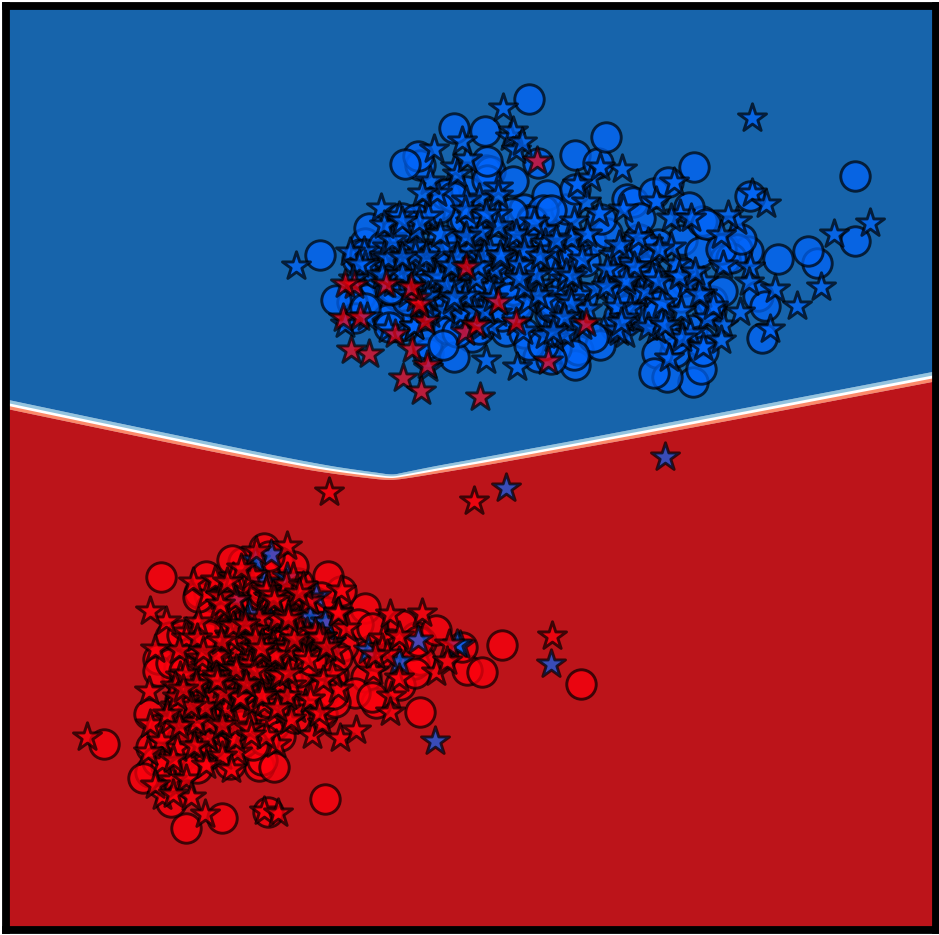
Y. Dubois, D. Kiela, D. J. Schwab, R. Vedantam
NeurIPS 2020 Spotlight Presentation πTLDR: We characterize and approximate optimal representations for supervised learning.
Information Theory, Representation Learning, VisionRobustness
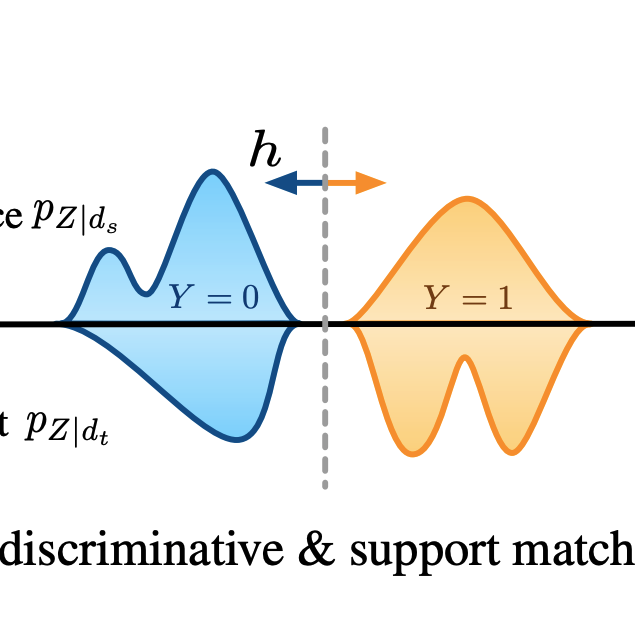
Y Ruan*, Y. Dubois*, C. J. Maddison
ICLR 2021TLDR: We give a simple variational objective whose optima are exactly the set of representations that are robust under covariate shift.
Information Theory, Invariance, Representation Learning, Robustness, Self-Supervised Learning, Vision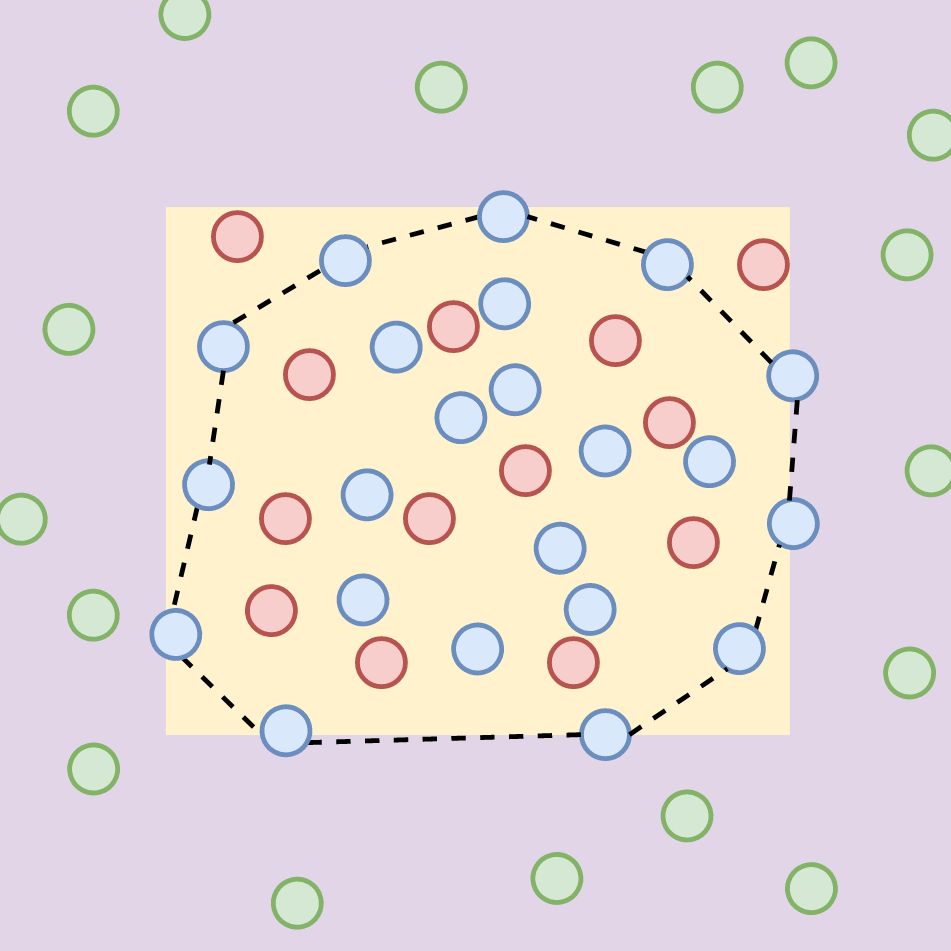
Y. Dubois, Gautier Dagan, Dieuwke Hupkes, Elia Bruni
ACL 2020TLDR: We propose an attention that improves extrapolation capacity of neural NLP models.
NLP, RobustnessSafety
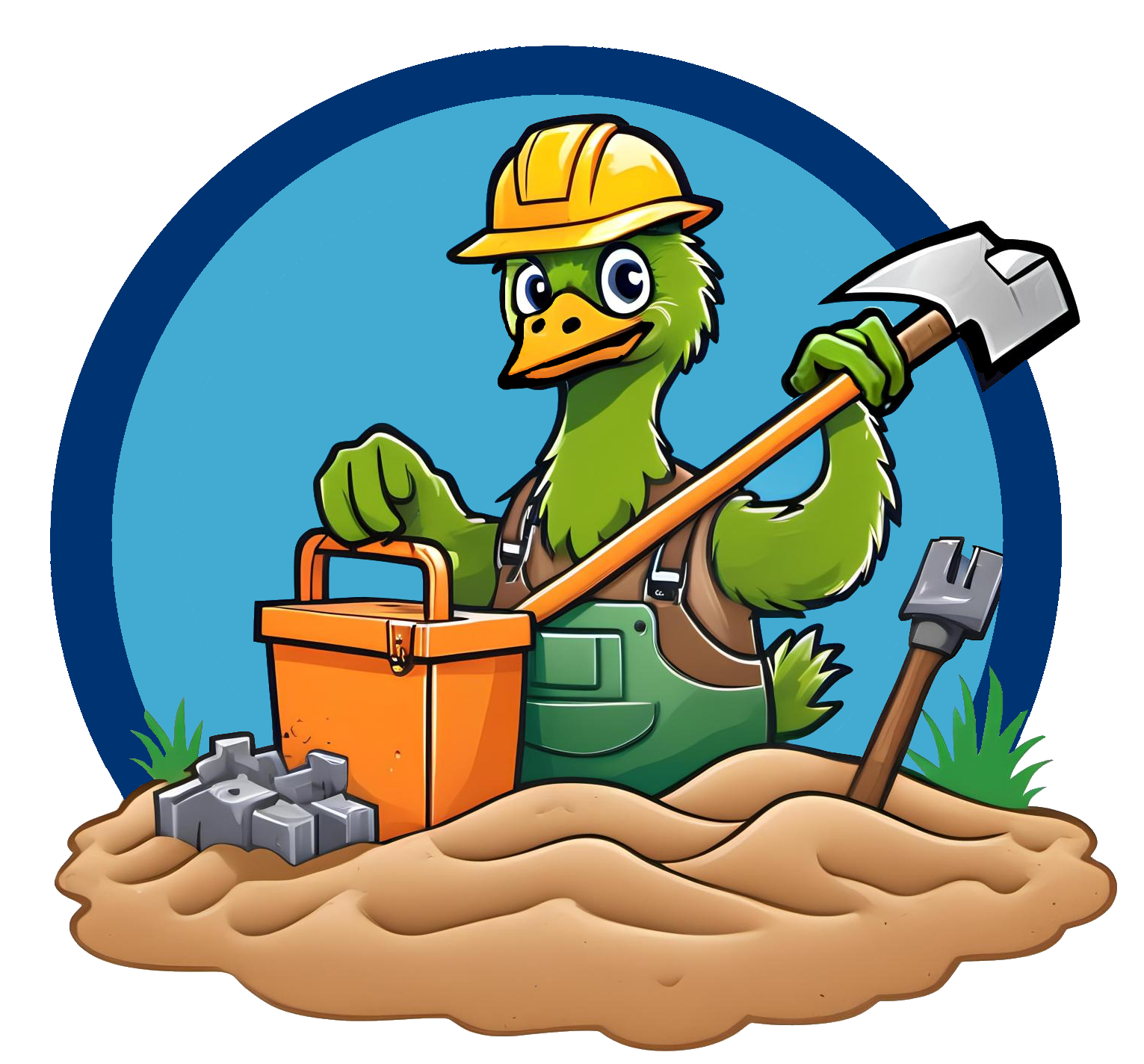
Y. Ruan*, H. Dong*, A. Wang, S. Pitis, Y. Zhou, J. Ba, Y. Dubois, C. J. Maddison, T. Hashimoto
ICLR 2024 Spotlight Presentation πTLDR: An LM-based emulation framework for identifying the risks of LM agents at scale.
Selected Papers Evaluation, NLP, Safety,Self-Supervised Learning
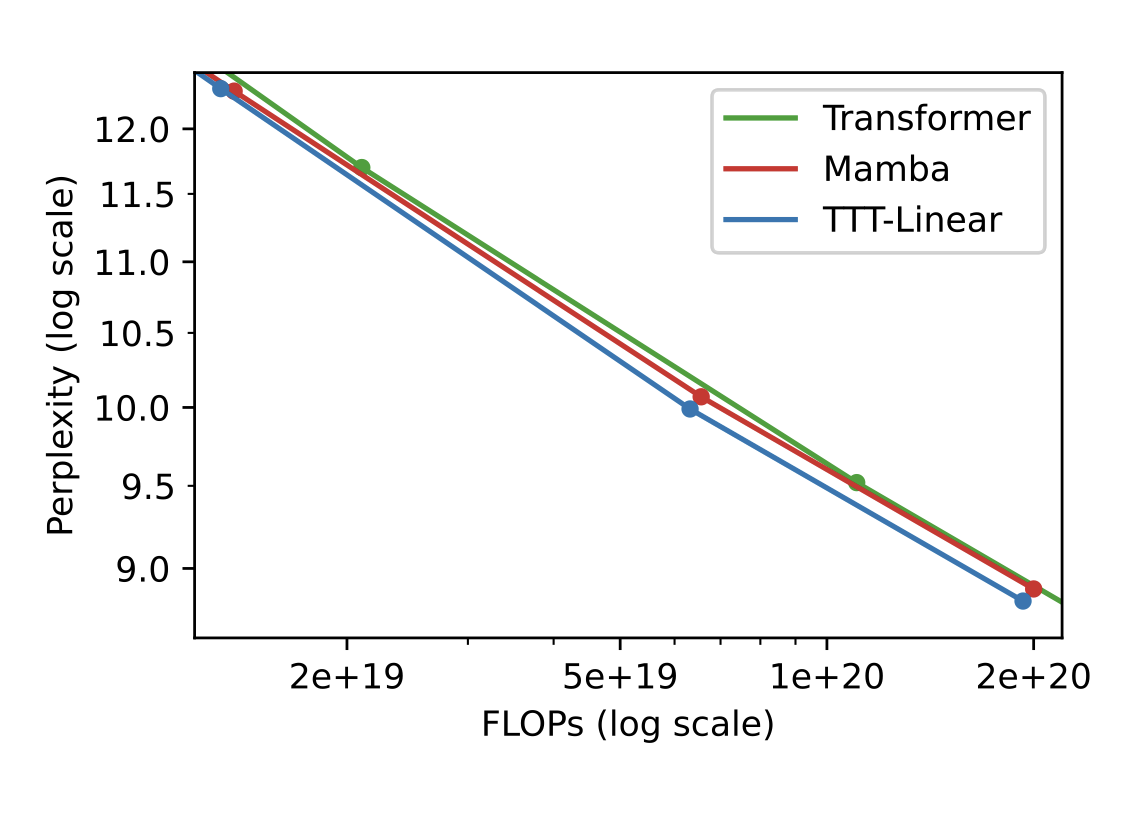
Yu Sun, Xinhao Li, Karan Dalal, Jiarui Xu, Arjun Vikram, Genghan Zhang, Y. Dubois, Xinlei Chen, Xiaolong Wang, Sanmi Koyejo, Tatsunori Hashimoto, Carlos Guestrin
ArXivTLDR: A new language model layer that is more expressive than RNN but more efficient than attention.
NLP, Self-Supervised Learning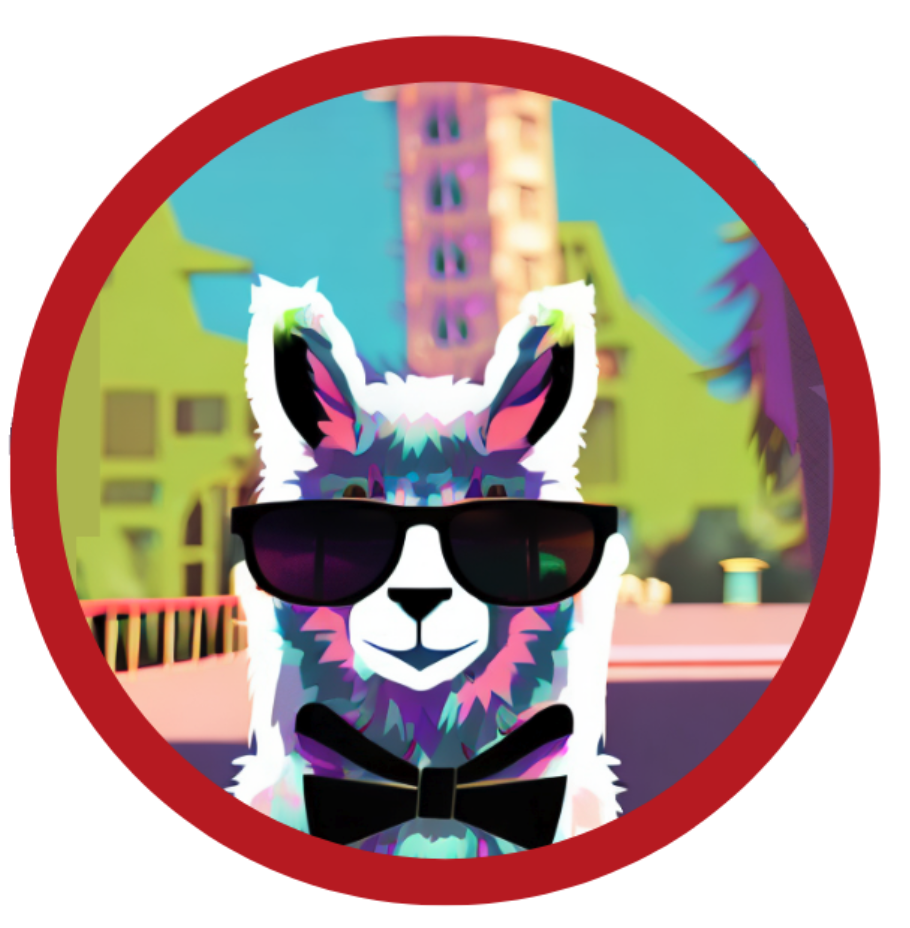
R. Taori*, I. Gulrajani*, T. Zhang*, Y. Dubois*, X. Li*, C. Guestrin, P. Liang, T. Hashimoto
GitHubTLDR: We introduce Alpaca 7B, a instruction-following fine-tuned LLaMA model. On our preliminary evaluation, Alpaca behaves qualitatively similarly to OpenAIβs text-davinci-003, while being surprisingly small and easy/cheap to reproduce (<600$).
Selected Papers NLP, RLHF, Self-Supervised Learning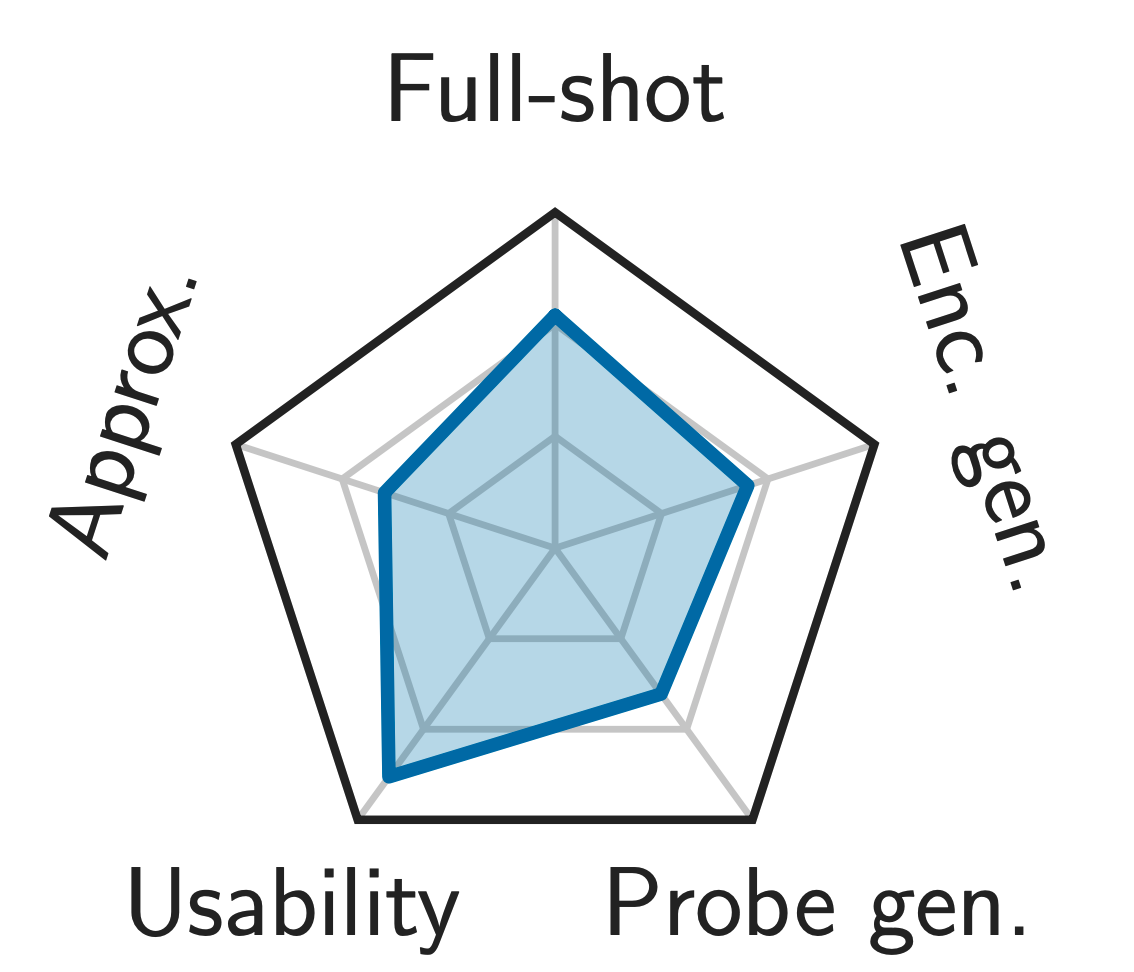
Y. Dubois, T. Hashimoto, P. Liang
ICML 2023 Oral Presentation πTLDR: We derive a risk decomposition for self-supervised learning and use it to evaluate 169 pretrained models.
Selected Papers Evaluation, Representation Learning, Self-Supervised Learning, Vision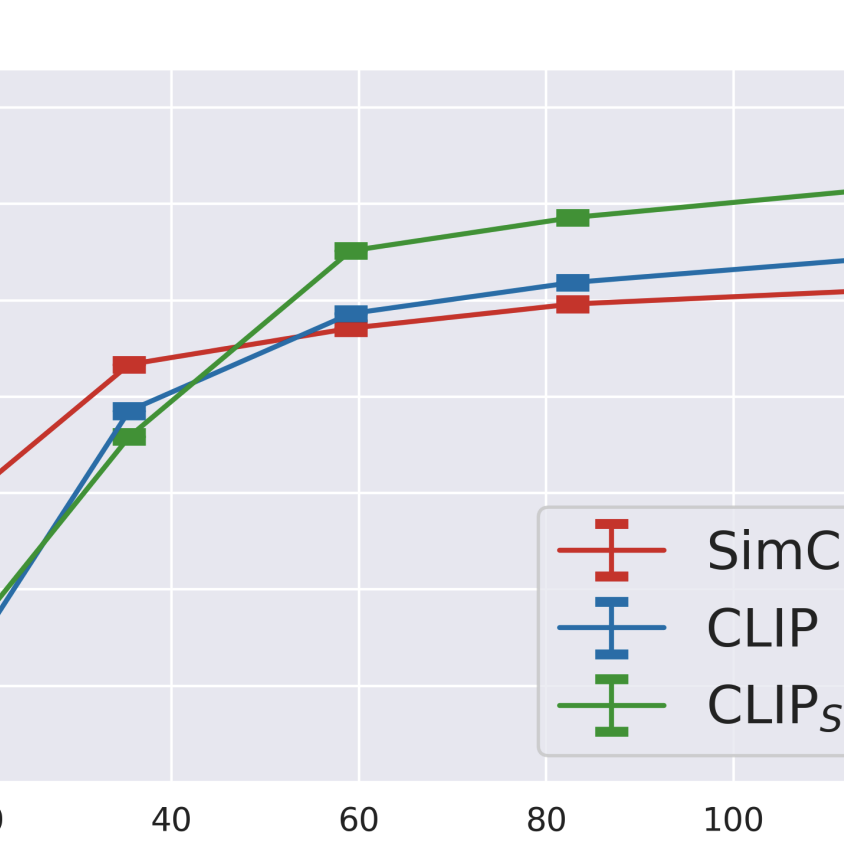
S. Santurkar, Y. Dubois, R. Taori, P. Liang, T. Hashimoto
ICLR 2022TLDR: Our work performs a systematic investigation into whether additional language supervision (in CLIP) helps models learn more transferrable representations.
NLP, Representation Learning, Self-Supervised Learning, Vision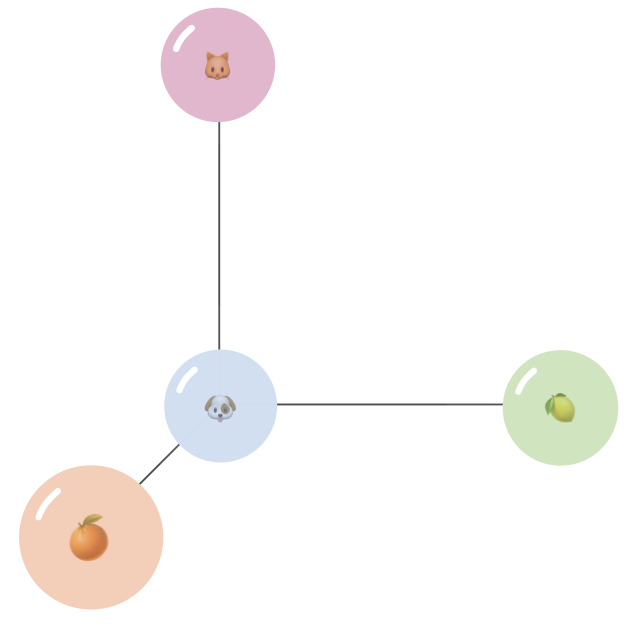
Y. Dubois, T. Hashimoto, S. Ermon, P. Liang
NeurIPS 2022TLDR: We characterize idealized self-supervised representations, which leads to actionable insights for improving SSL algorithms.
Selected Papers Invariance, Representation Learning, Self-Supervised Learning, Vision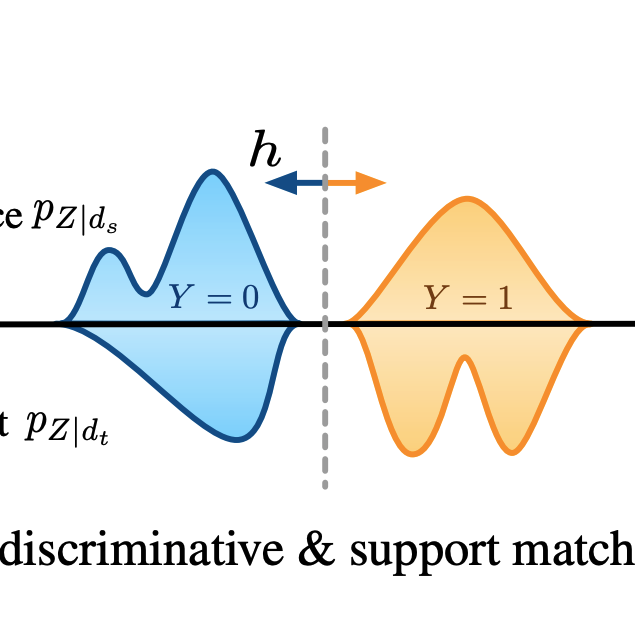
Y Ruan*, Y. Dubois*, C. J. Maddison
ICLR 2021TLDR: We give a simple variational objective whose optima are exactly the set of representations that are robust under covariate shift.
Information Theory, Invariance, Representation Learning, Robustness, Self-Supervised Learning, Vision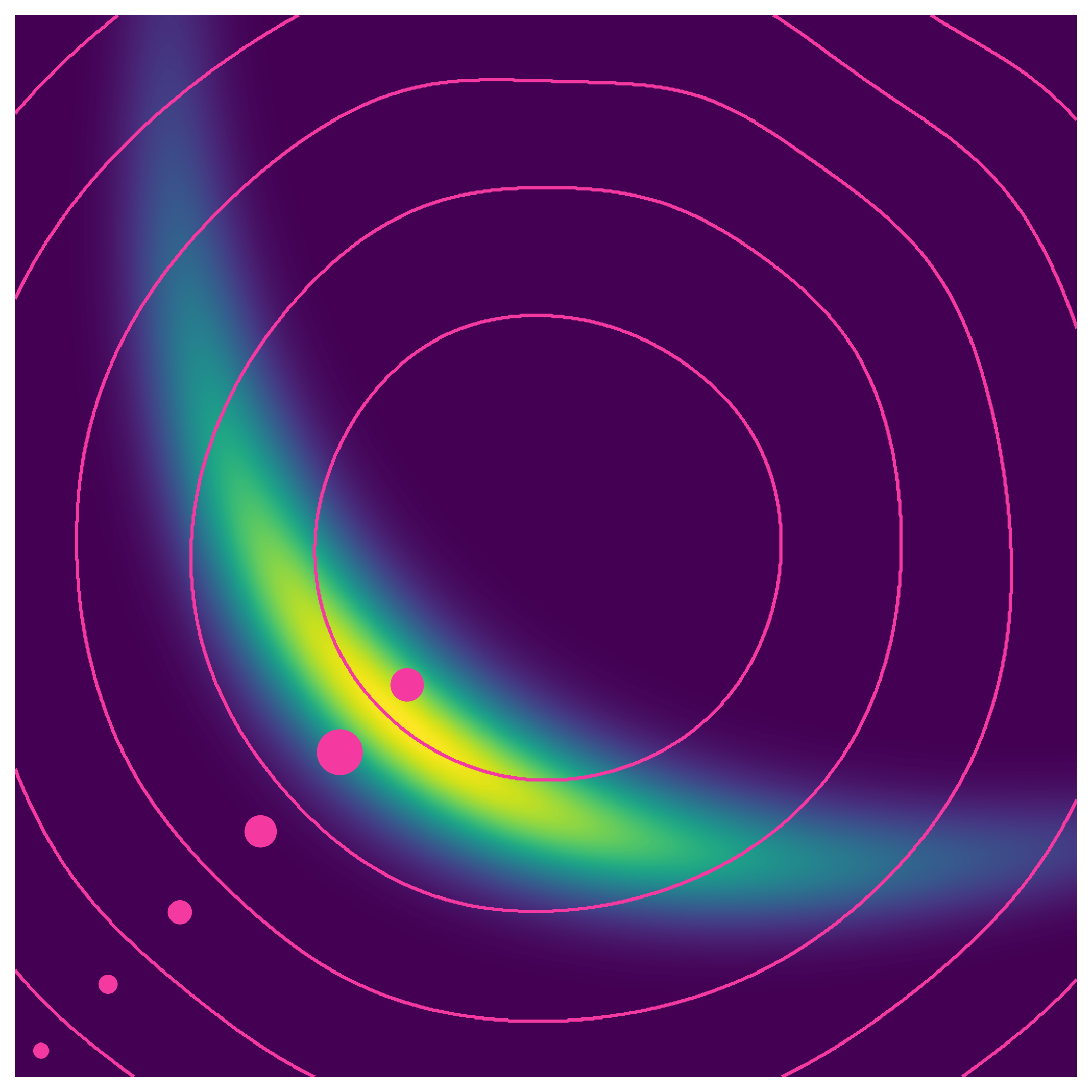
Y. Dubois, B. Bloem-Reddy, K. Ullrich, C. J. Maddison
NeurIPS 2021 Spotlight Presentation πTLDR: We formalize compression with respect to ML algorithms rather than human perception.
Selected Papers Compression, Information Theory, Invariance, Representation Learning, Self-Supervised Learning, VisionTime Series
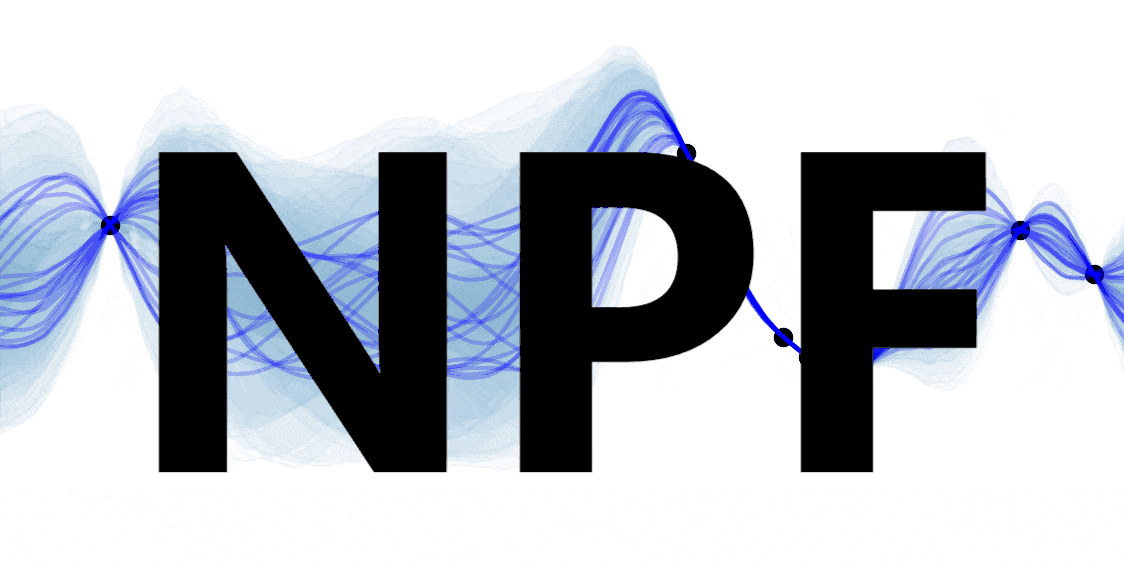
Y. Dubois*, J. Gordon*, A. Foong*
GitHubTLDR: A simple and unifying explanation of the neural process family, which are a collection of models that meta-learn a distribution over predictors.
Equivariance, Neural Processes, Time Series, Uncertainty, Vision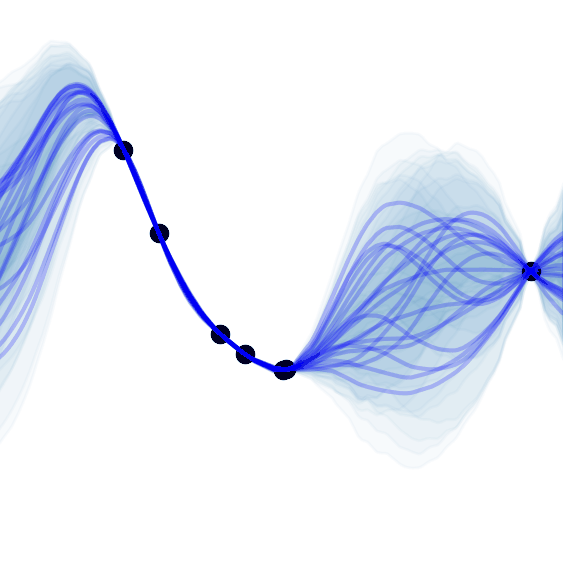
A. Y. K. Foong*, W. P. Bruinsma*, J. Gordon*, Y. Dubois, J. Requeima, R. E. Turner
NeurIPS 2020TLDR: We propose a translation equivariant (latent) neural process.
Equivariance, Neural Processes, Time Series, Uncertainty, Vision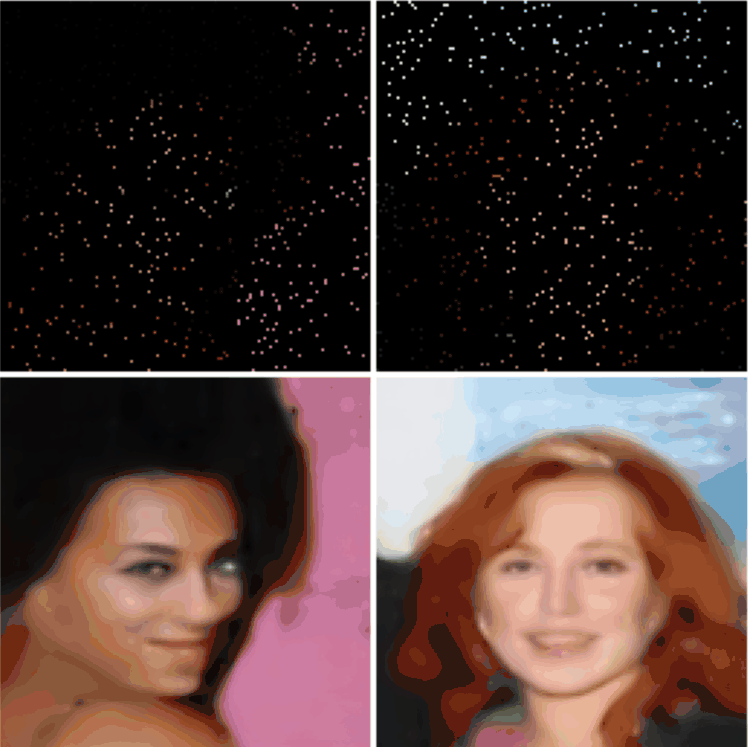
J. Gordon*, W. P. Bruinsma*, A. Y. K. Foong, J. Requeima,Y. Dubois, R. E. Turner
ICLR 2020 Oral Presentation πTLDR: We propose a translation equivariant conditional neural process.
Equivariance, Neural Processes, Time Series, Uncertainty, VisionUncertainty
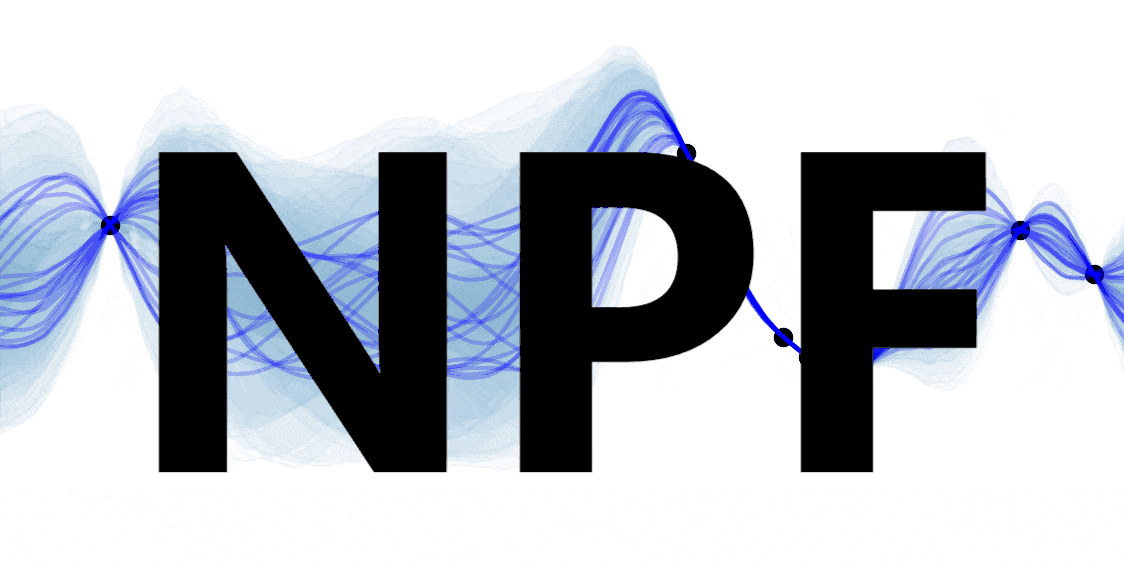
Y. Dubois*, J. Gordon*, A. Foong*
GitHubTLDR: A simple and unifying explanation of the neural process family, which are a collection of models that meta-learn a distribution over predictors.
Equivariance, Neural Processes, Time Series, Uncertainty, Vision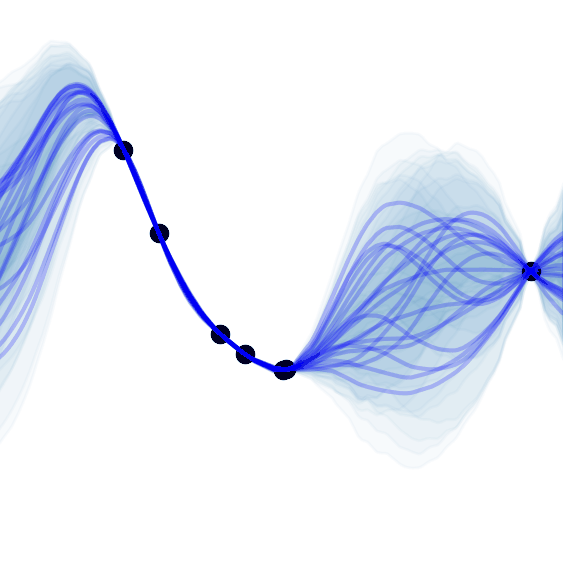
A. Y. K. Foong*, W. P. Bruinsma*, J. Gordon*, Y. Dubois, J. Requeima, R. E. Turner
NeurIPS 2020TLDR: We propose a translation equivariant (latent) neural process.
Equivariance, Neural Processes, Time Series, Uncertainty, Vision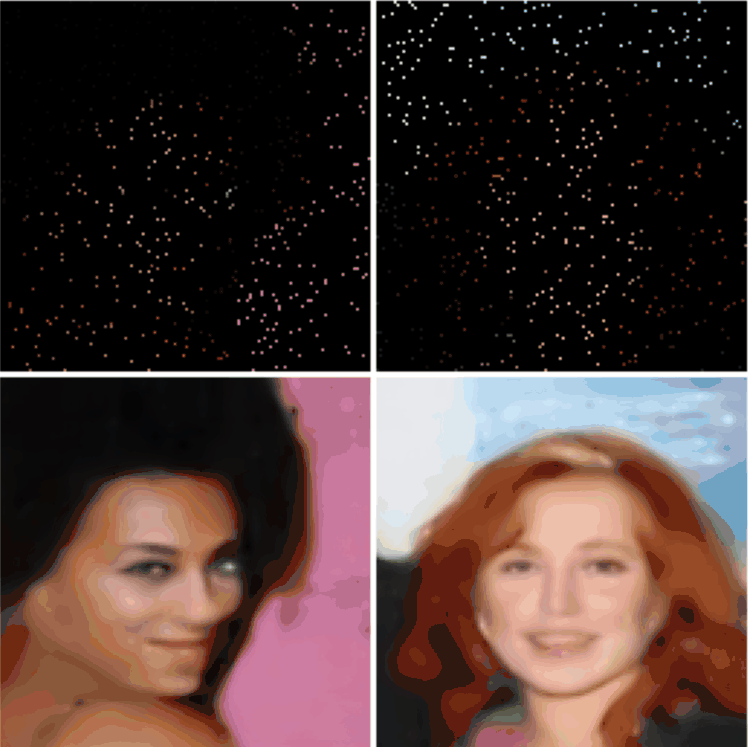
J. Gordon*, W. P. Bruinsma*, A. Y. K. Foong, J. Requeima,Y. Dubois, R. E. Turner
ICLR 2020 Oral Presentation πTLDR: We propose a translation equivariant conditional neural process.
Equivariance, Neural Processes, Time Series, Uncertainty, VisionVision
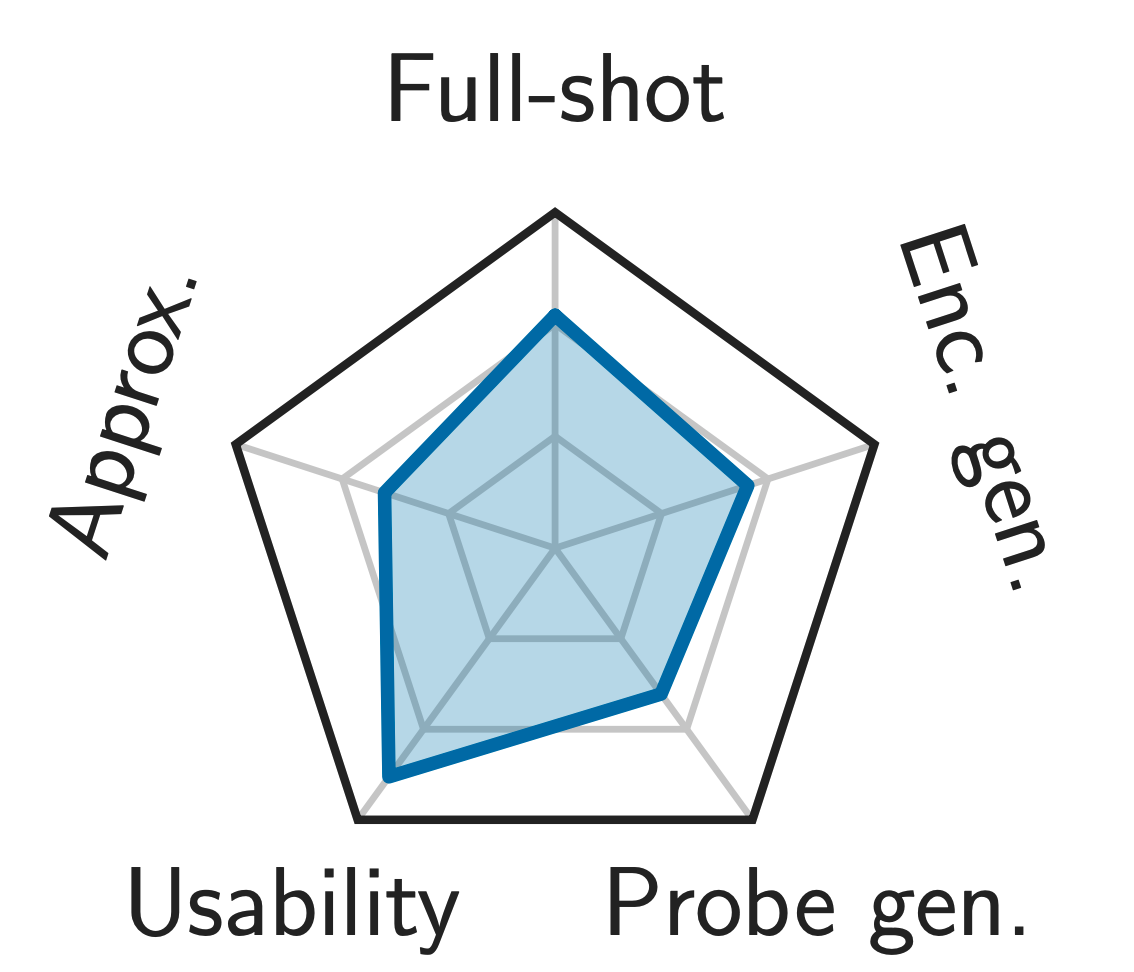
Y. Dubois, T. Hashimoto, P. Liang
ICML 2023 Oral Presentation πTLDR: We derive a risk decomposition for self-supervised learning and use it to evaluate 169 pretrained models.
Selected Papers Evaluation, Representation Learning, Self-Supervised Learning, Vision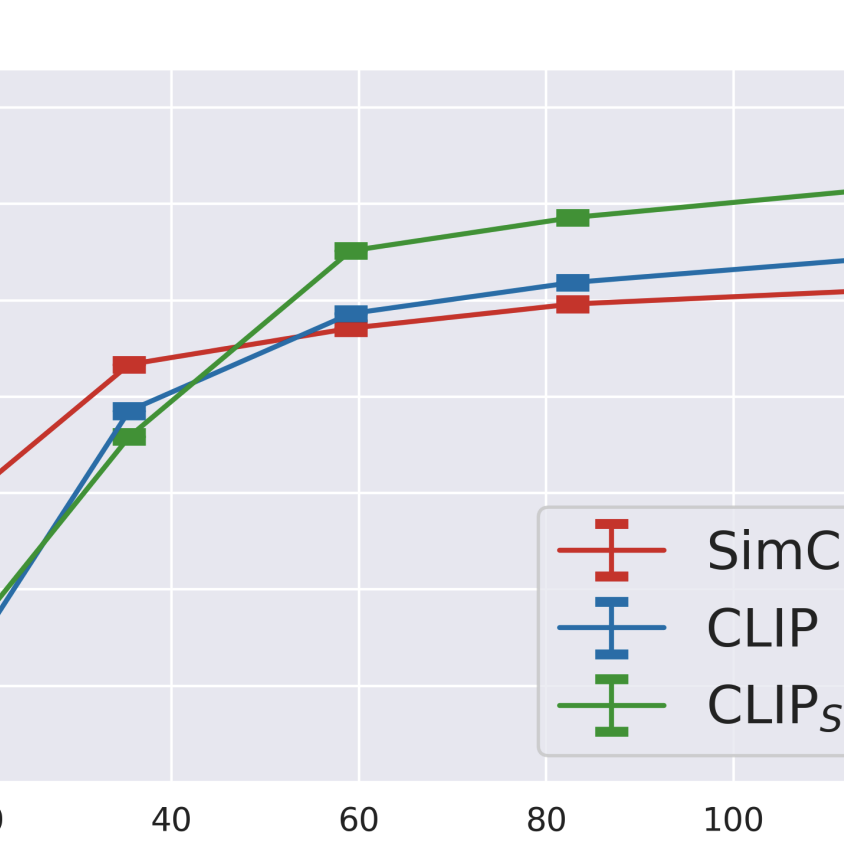
S. Santurkar, Y. Dubois, R. Taori, P. Liang, T. Hashimoto
ICLR 2022TLDR: Our work performs a systematic investigation into whether additional language supervision (in CLIP) helps models learn more transferrable representations.
NLP, Representation Learning, Self-Supervised Learning, Vision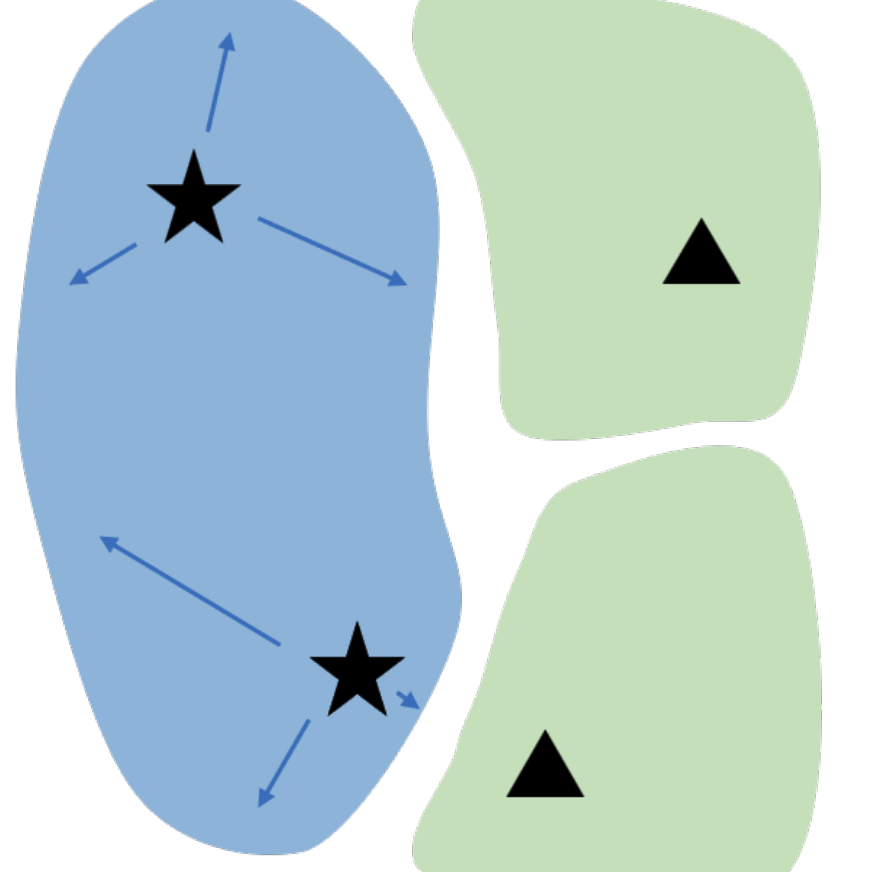
N. Miao, E. Mathieu, Y. Dubois, T. Rainforth, Y. W. Teh, A. Foster, H. Kim
ICML 2023TLDR: We introduce a method for automatically learning input-specific augmentations from data.
Invariance, Vision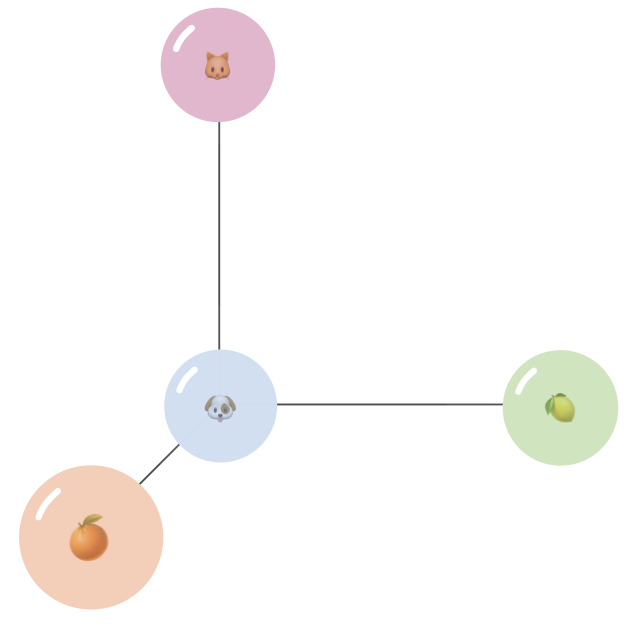
Y. Dubois, T. Hashimoto, S. Ermon, P. Liang
NeurIPS 2022TLDR: We characterize idealized self-supervised representations, which leads to actionable insights for improving SSL algorithms.
Selected Papers Invariance, Representation Learning, Self-Supervised Learning, Vision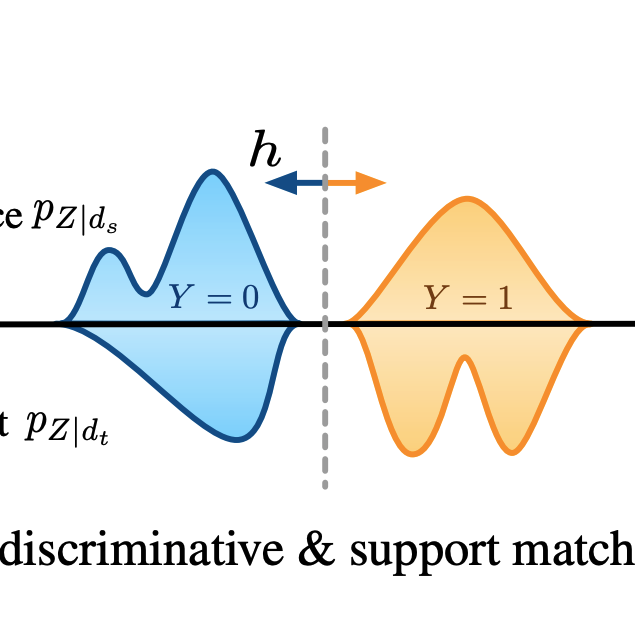
Y Ruan*, Y. Dubois*, C. J. Maddison
ICLR 2021TLDR: We give a simple variational objective whose optima are exactly the set of representations that are robust under covariate shift.
Information Theory, Invariance, Representation Learning, Robustness, Self-Supervised Learning, Vision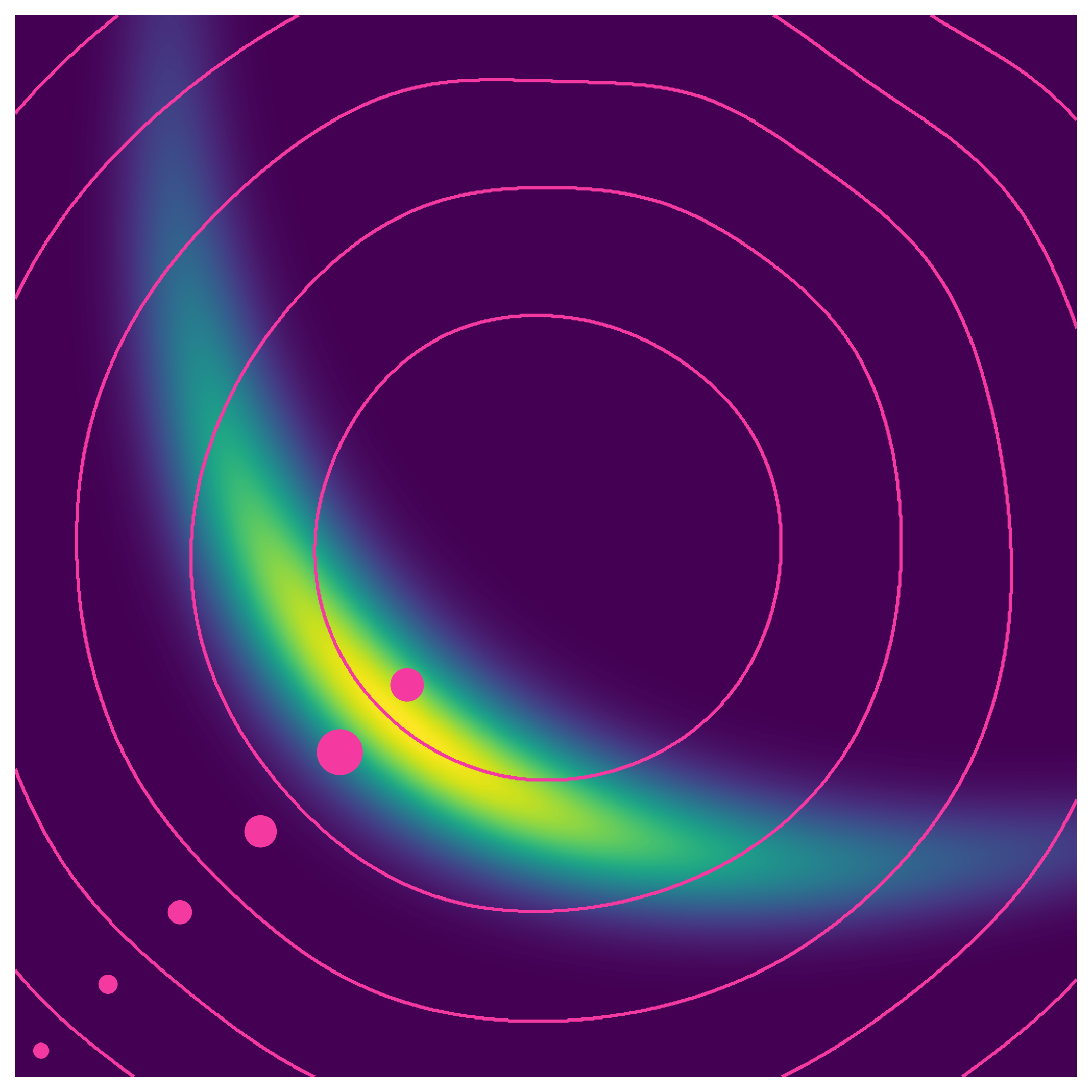
Y. Dubois, B. Bloem-Reddy, K. Ullrich, C. J. Maddison
NeurIPS 2021 Spotlight Presentation πTLDR: We formalize compression with respect to ML algorithms rather than human perception.
Selected Papers Compression, Information Theory, Invariance, Representation Learning, Self-Supervised Learning, Vision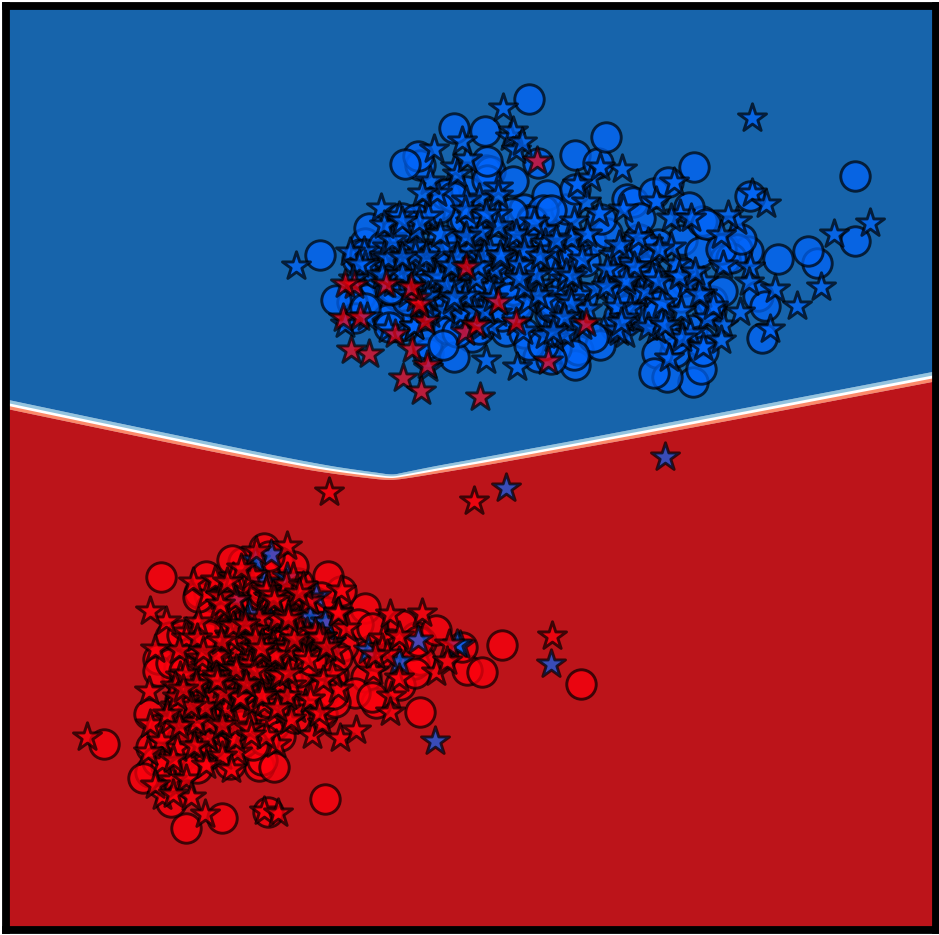
Y. Dubois, D. Kiela, D. J. Schwab, R. Vedantam
NeurIPS 2020 Spotlight Presentation πTLDR: We characterize and approximate optimal representations for supervised learning.
Information Theory, Representation Learning, Vision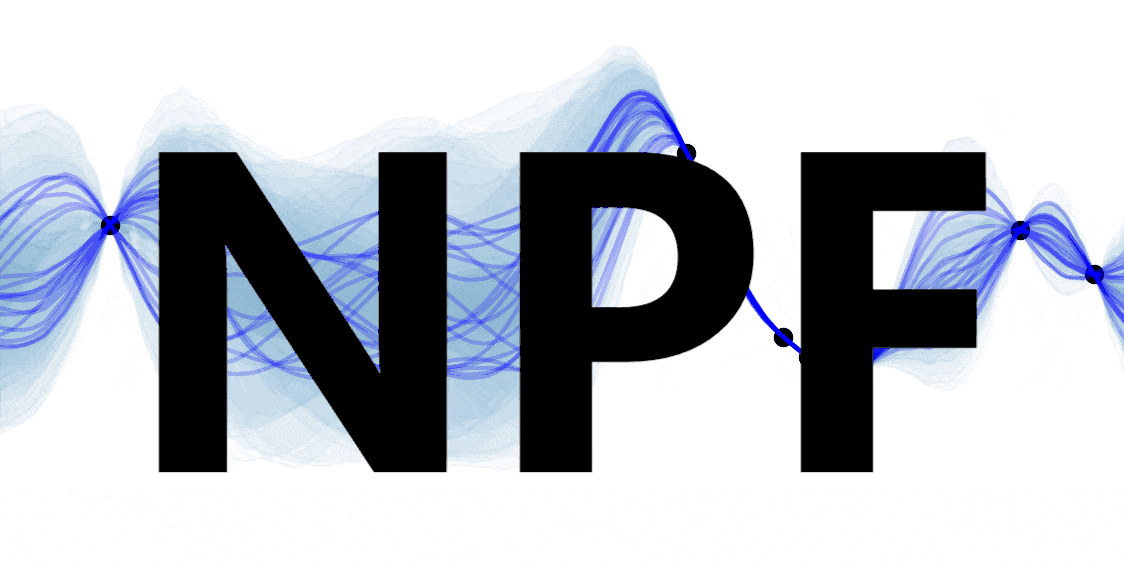
Y. Dubois*, J. Gordon*, A. Foong*
GitHubTLDR: A simple and unifying explanation of the neural process family, which are a collection of models that meta-learn a distribution over predictors.
Equivariance, Neural Processes, Time Series, Uncertainty, Vision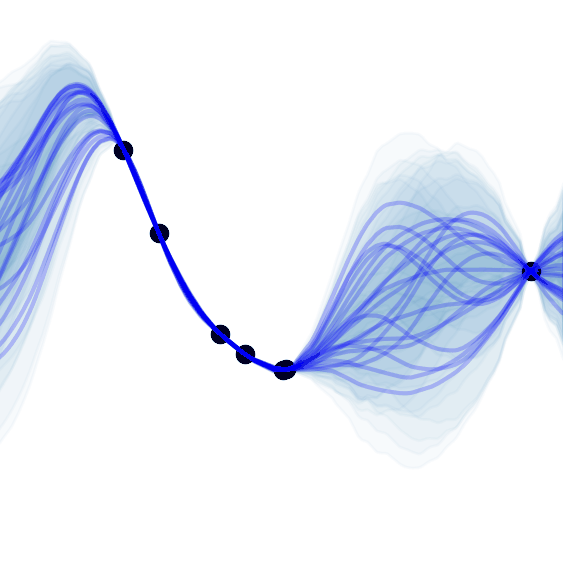
A. Y. K. Foong*, W. P. Bruinsma*, J. Gordon*, Y. Dubois, J. Requeima, R. E. Turner
NeurIPS 2020TLDR: We propose a translation equivariant (latent) neural process.
Equivariance, Neural Processes, Time Series, Uncertainty, Vision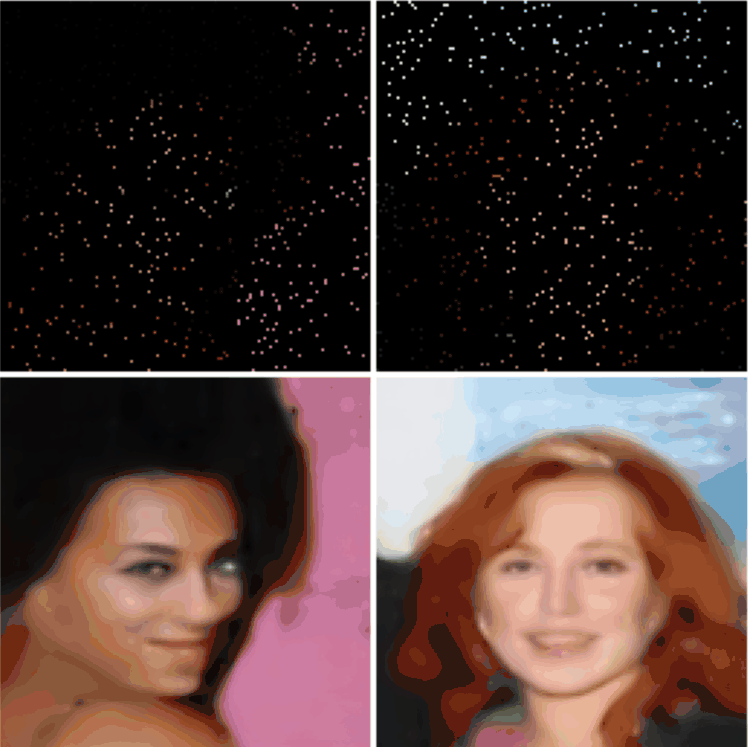
J. Gordon*, W. P. Bruinsma*, A. Y. K. Foong, J. Requeima,Y. Dubois, R. E. Turner
ICLR 2020 Oral Presentation πTLDR: We propose a translation equivariant conditional neural process.
Equivariance, Neural Processes, Time Series, Uncertainty, Vision